COCO Reader with augmentations¶
Reader operator that reads a COCO dataset (or subset of COCO), which consists of an annotation file and the images directory.
[1]:
from __future__ import print_function
from nvidia.dali.pipeline import Pipeline
import nvidia.dali.ops as ops
import nvidia.dali.types as types
import numpy as np
from time import time
subset = "val"
file_root = "/data/coco/coco-2017/coco2017/" + subset + "2017"
annotations_file = "/data/coco/coco-2017/coco2017/annotations/instances_" + subset + "2017.json"
num_gpus = 1
batch_size = 16
Create reader, decoder and flip operator for images and bounding boxes
[1]:
class COCOPipeline(Pipeline):
def __init__(self, batch_size, num_threads, device_id):
super(COCOPipeline, self).__init__(
batch_size, num_threads, device_id, seed=15)
self.input = ops.COCOReader(
file_root=file_root,
annotations_file=annotations_file,
shard_id=device_id,
num_shards=num_gpus,
ratio=True,
ltrb=True)
self.decode = ops.ImageDecoder(device="mixed", output_type=types.RGB)
self.flip = ops.Flip(device="gpu")
self.bbflip = ops.BbFlip(device="cpu", ltrb=True)
self.paste_pos = ops.Uniform(range=(0, 1))
self.paste_ratio = ops.Uniform(range=(1, 2))
self.coin = ops.CoinFlip(probability=0.5)
self.coin2 = ops.CoinFlip(probability=0.5)
self.paste = ops.Paste(device="gpu", fill_value=(32, 64, 128))
self.bbpaste = ops.BBoxPaste(device="cpu", ltrb=True)
self.prospective_crop = ops.RandomBBoxCrop(
device="cpu",
aspect_ratio=[0.5, 2.0],
thresholds=[0.1, 0.3, 0.5],
scaling=[0.8, 1.0],
ltrb=True)
self.slice = ops.Slice(device="gpu")
def define_graph(self):
rng = self.coin()
rng2 = self.coin2()
inputs, bboxes, labels = self.input()
images = self.decode(inputs)
# Paste and BBoxPaste need to use same scales and positions
ratio = self.paste_ratio()
px = self.paste_pos()
py = self.paste_pos()
images = self.paste(images, paste_x=px, paste_y=py, ratio=ratio)
bboxes = self.bbpaste(bboxes, paste_x=px, paste_y=py, ratio=ratio)
crop_begin, crop_size, bboxes, labels = self.prospective_crop(bboxes, labels)
images = self.slice(images, crop_begin, crop_size)
images = self.flip(images, horizontal=rng, vertical=rng2)
bboxes = self.bbflip(bboxes, horizontal=rng, vertical=rng2)
return (images, bboxes, labels)
[1]:
start = time()
pipes = [COCOPipeline(batch_size=batch_size, num_threads=2, device_id=device_id) for device_id in range(num_gpus)]
for pipe in pipes:
pipe.build()
total_time = time() - start
print("Computation graph built and dataset loaded in %f seconds." % total_time)
Computation graph built and dataset loaded in 0.133818 seconds.
[1]:
pipe_out = [pipe.run() for pipe in pipes]
images_cpu = pipe_out[0][0].as_cpu()
bboxes_cpu = pipe_out[0][1]
labels_cpu = pipe_out[0][2]
Bounding boxes returned by the operator are lists of floats in format [left, top, right, bottom] in relative cooradinates (ratio=**True**).
[1]:
img_index = 4
bboxes = bboxes_cpu.at(4)
bboxes
[1]:
array([[0.35708272, 0.21298414, 0.7092717 , 0.9250916 ],
[0.37862933, 0.71613264, 0.47067046, 0.7850058 ],
[0.64946544, 0.7192394 , 0.7538513 , 0.80209315],
[0.788571 , 0.8646966 , 0.79831743, 0.8863505 ],
[0.8066003 , 0.7938396 , 0.81441784, 0.80944836],
[0.47354114, 0.7297014 , 0.5090437 , 0.7740011 ],
[0.79964495, 0.75910354, 0.8300644 , 0.7933717 ],
[0.7725954 , 0.7599083 , 0.7831815 , 0.7877945 ],
[0.1961242 , 0.6791882 , 0.3481078 , 0.8887461 ],
[0.3789811 , 0.7184534 , 0.4721682 , 0.7850058 ],
[0.33182585, 0.7102747 , 0.3813411 , 0.78414494],
[0.8793073 , 0.8202472 , 0.88524145, 0.826704 ]], dtype=float32)
Let’s see the ground truth bounding boxes drawn on the image.
[1]:
import matplotlib.pyplot as plt
import matplotlib.patches as patches
import random
img = images_cpu.at(img_index)
H = img.shape[0]
W = img.shape[1]
fig,ax = plt.subplots(1)
ax.imshow(img)
bboxes = bboxes_cpu.at(img_index)
labels = labels_cpu.at(img_index)
categories_set = set()
for label in labels:
categories_set.add(label[0])
category_id_to_color = dict(
[(cat_id, [random.uniform(0, 1), random.uniform(0, 1), random.uniform(0, 1)]) for cat_id in categories_set])
for bbox, label in zip(bboxes, labels):
rect = patches.Rectangle(
(bbox[0] * W, bbox[1] * H), # Absolute corner coordinates
(bbox[2] - bbox[0]) * W, # Absolute bounding box width
(bbox[3] - bbox[1]) * H, # Absolute bounding box height
linewidth=1,
edgecolor=category_id_to_color[label[0]],
facecolor='none')
ax.add_patch(rect)
plt.show()
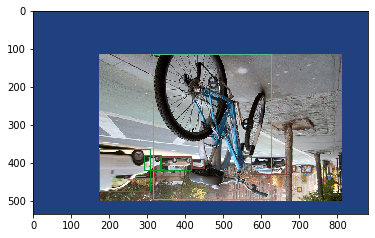