BERT Results
Training Accuracy Results
Training accuracy: NVIDIA DGX SuperPOD (16 × 8 × A100 80GB for 4b BERT model)
Training the 4B BERT model for 95 Billion takes 1.5 days. The figure below shows the loss curve.
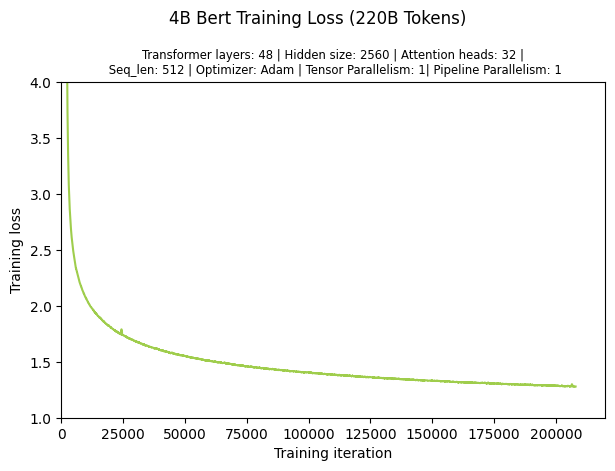
4B BERT Training Loss (220B Tokens)
The table below shows the converged training loss, the throughput, and the total time to train for the 4B BERT model, using a given number of GPUs and a given Global Batch Size (GBS).
Training Performance Results
Training performance: NVIDIA DGX SuperPOD (20 × 8 × A100 80GB for 4B BERT model)
NVIDIA measured the throughput of training a 4B parameter BERT model on NVIDIA DGX SuperPOD using different numbers of nodes. Scaling from 1 node to 16 nodes yielded a 12.71× speed-up. The table and chart below show the performance results.
Nodes |
||||||
---|---|---|---|---|---|---|
1 |
2 |
4 |
8 |
16 |
||
Tokens per Second |
57287 |
108695 |
215358 |
393167 |
728178 |
|
4B |
Perfect Linear Scaling (Tokens) |
57287 |
114574 |
229148 |
458296 |
916592 |
Speed-up |
1x |
1.89x |
3.75x |
6.86x |
12.71x |
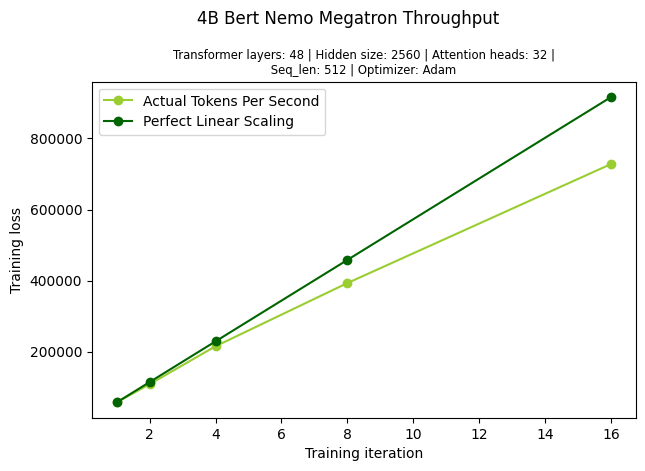
4B BERT NeMo Framework Throughput