cuSPARSE
The API reference guide for cuSPARSE, the CUDA sparse matrix library.
1. Introduction
Provide Feedback: Math-Libs-Feedback@nvidia.com
cuSPARSE Release Notes: cuda-toolkit-release-notes
cuSPARSE GitHub Samples: CUDALibrarySamples
It is implemented on top of the NVIDIA® CUDA™ runtime (which is part of the CUDA Toolkit) and is designed to be called from C and C++.
The library routines can be classified into four categories:
Level 1: operations between a vector in sparse format and a vector in dense format
Level 2: operations between a matrix in sparse format and a vector in dense format
Level 3: operations between a matrix in sparse format and a set of vectors in dense format (which can also usually be viewed as a dense tall matrix)
Conversion: operations that allow conversion between different matrix formats, and compression of csr matrices.
The cuSPARSE library allows developers to access the computational resources of the NVIDIA graphics processing unit (GPU), although it does not auto-parallelize across multiple GPUs. The cuSPARSE API assumes that input and output data reside in GPU (device) memory, unless it is explicitly indicated otherwise by the string DevHostPtr
in a function parameter’s name.
It is the responsibility of the developer to allocate memory and to copy data between GPU memory and CPU memory using standard CUDA runtime API routines, such as cudaMalloc()
, cudaFree()
, cudaMemcpy()
, and cudaMemcpyAsync()
.
1.1. Naming Conventions
The cuSPARSE library functions are available for data types float
, double
, cuComplex
, and cuDoubleComplex
. The sparse Level 1, Level 2, and Level 3 functions follow this naming convention:
cusparse
<t
>[<matrix data format
>]<operation
>[<output matrix data format
>]
where <t
> can be S
, D
, C
, Z
, or X
, corresponding to the data types float
, double
, cuComplex
, cuDoubleComplex
, and the generic type, respectively.
The <matrix data format
> can be dense
, coo
, csr
, or csc
, corresponding to the dense, coordinate, compressed sparse row, and compressed sparse column formats, respectively.
Finally, the <operation
> can be axpyi
, gthr
, gthrz
, roti
, or sctr
, corresponding to the Level 1 functions; it also can be mv
or sv
, corresponding to the Level 2 functions, as well as mm
or sm
, corresponding to the Level 3 functions.
All of the functions have the return type cusparseStatus_t
and are explained in more detail in the chapters that follow.
1.2. Asynchronous Execution
The cuSPARSE library functions are executed asynchronously with respect to the host and may return control to the application on the host before the result is ready. Developers can use the cudaDeviceSynchronize()
function to ensure that the execution of a particular cuSPARSE library routine has completed.
A developer can also use the cudaMemcpy()
routine to copy data from the device to the host and vice versa, using the cudaMemcpyDeviceToHost
and cudaMemcpyHostToDevice
parameters, respectively. In this case there is no need to add a call to cudaDeviceSynchronize()
because the call to cudaMemcpy()
with the above parameters is blocking and completes only when the results are ready on the host.
1.3. Static Library Support
Starting with release 6.5, the cuSPARSE Library is also delivered in a static form as libcusparse_static.a
on Linux.
For example, to compile a small application using cuSPARSE against the dynamic library, the following command can be used:
nvcc myCusparseApp.c -lcusparse -o myCusparseApp>
Whereas to compile against the static cuSPARSE library, the following command has to be used:
nvcc myCusparseApp.c -lcusparse_static -o myCusparseApp>
It is also possible to use the native Host C++ compiler. Depending on the Host Operating system, some additional libraries like pthread
or dl
might be needed on the linking line. The following command on Linux is suggested:
g++ myCusparseApp.c -lcusparse_static -lcudart_static -lpthread -ldl -I <cuda-toolkit-path>/include -L <cuda-toolkit-path>/lib64 -o myCusparseApp
Note that in the latter case, the library cuda
is not needed. The CUDA Runtime will try to open explicitly the cuda
library if needed. In the case of a system which does not have the CUDA driver installed, this allows the application to gracefully manage this issue and potentially run if a CPU-only path is available.
1.4. Library Dependencies
Starting with CUDA 12.0, cuSPARSE will depend on nvJitLink library for JIT (just-in-time) LTO (link-time-optimization) capabilities; refer to the cusparseSpMMOp APIs for more information.
If the user links to the dynamic library, the environment variables for loading the libraries (such as LD_LIBRARY_PATH
on Linux and PATH
on Windows) must include the path where libnvjitlink.so
is located. If it is in the same directory as cuSPARSE, the user doesn’t need to take any action. If linking to the static library, the user needs to link with -lnvjitlink
and set LIBRARY_PATH/PATH
accordingly.
2. Using the cuSPARSE API
This chapter describes how to use the cuSPARSE library API. It is not a reference for the cuSPARSE API data types and functions; that is provided in subsequent chapters.
2.1. Thread Safety
The library is thread safe and its functions can be called from multiple host threads. However, simultaneous read/writes of the same objects (or of the same handle) are not safe. Hence the handle must be private per thread, i.e., only one handle per thread is safe.
2.2. Scalar Parameters
In the cuSPARSE API, the scalar parameters \(\alpha\) and \(\beta\) can be passed by reference on the host or the device.
The few functions that return a scalar result, such as nnz()
, return the resulting value by reference on the host or the device. Even though these functions return immediately, similarly to those that return matrix and vector results, the scalar result is not ready until execution of the routine on the GPU completes. This requires proper synchronization be used when reading the result from the host.
This feature allows the cuSPARSE library functions to execute completely asynchronously using streams, even when \(\alpha\) and \(\beta\) are generated by a previous kernel. This situation arises, for example, when the library is used to implement iterative methods for the solution of linear systems and eigenvalue problems [3].
2.3. Parallelism with Streams
If the application performs several small independent computations, or if it makes data transfers in parallel with the computation, CUDA streams can be used to overlap these tasks.
The application can conceptually associate a stream with each task. To achieve the overlap of computation between the tasks, the developer should create CUDA streams using the function cudaStreamCreate()
and set the stream to be used by each individual cuSPARSE library routine by calling cusparseSetStream()
just before calling the actual cuSPARSE routine. Then, computations performed in separate streams would be overlapped automatically on the GPU, when possible. This approach is especially useful when the computation performed by a single task is relatively small and is not enough to fill the GPU with work, or when there is a data transfer that can be performed in parallel with the computation.
When streams are used, we recommend using the new cuSPARSE API with scalar parameters and results passed by reference in the device memory to achieve maximum computational overlap.
Although a developer can create many streams, in practice it is not possible to have more than 16 concurrent kernels executing at the same time.
2.4. Compatibility and Versioning
The cuSPARSE APIs are intended to be backward compatible at the source level with future releases (unless stated otherwise in the release notes of a specific future release). In other words, if a program uses cuSPARSE, it should continue to compile and work correctly with newer versions of cuSPARSE without source code changes. cuSPARSE is not guaranteed to be backward compatible at the binary level. Using different versions of the cusparse.h
header file and the shared library is not supported. Using different versions of cuSPARSE and the CUDA runtime is not supported. The APIs should be backward compatible at the source level for public functions in most cases
2.5. Optimization Notes
Most of the cuSPARSE routines can be optimized by exploiting CUDA Graphs capture and Hardware Memory Compression features.
More in details, a single cuSPARSE call or a sequence of calls can be captured by a CUDA Graph and executed in a second moment. This minimizes kernels launch overhead and allows the CUDA runtime to optimize the whole workflow. A full example of CUDA graphs capture applied to a cuSPARSE routine can be found in cuSPARSE Library Samples - CUDA Graph.
Secondly, the data types and functionalities involved in cuSPARSE are suitable for Hardware Memory Compression available in Ampere GPU devices (compute capability 8.0) or above. The feature allows memory compression for data with enough zero bytes without no loss of information. The device memory must be allocation with the CUDA driver APIs. A full example of Hardware Memory Compression applied to a cuSPARSE routine can be found in cuSPARSE Library Samples - Memory Compression.
3. cuSPARSE Indexing and Data Formats
The cuSPARSE library supports dense and sparse vector, and dense and sparse matrix formats.
3.1. Index Base Format
The library supports zero- and one-based indexing. The index base is selected through the cusparseIndexBase_t
type, which is passed as a standalone parameter or as a field in the matrix descriptor cusparseMatDescr_t
type.
3.1.1. Vector Formats
This section describes dense and sparse vector formats.
3.1.1.1. Dense Format
Dense vectors are represented with a single data array that is stored linearly in memory, such as the following \(7 \times 1\) dense vector.
\(\begin{bmatrix} 1.0 & 0.0 & 0.0 & 2.0 & 3.0 & 0.0 & 4.0 \\ \end{bmatrix}\) |
(This vector is referenced again in the next section.)
3.1.1.2. Sparse Format
Sparse vectors are represented with two arrays.
The data array has the nonzero values from the equivalent array in dense format.
The integer index array has the positions of the corresponding nonzero values in the equivalent array in dense format.
For example, the dense vector in section 3.2.1 can be stored as a sparse vector with one-based indexing.
\(\begin{matrix} & & \begin{bmatrix} 1.0 & 2.0 & 3.0 & 4.0 \\ \end{bmatrix} \\ & & \begin{bmatrix} {1\phantom{.0}} & {4\phantom{.0}} & {5\phantom{.0}} & {7\phantom{.0}} \\ \end{bmatrix} \\ \end{matrix}\) |
It can also be stored as a sparse vector with zero-based indexing.
\(\begin{matrix} & & \begin{bmatrix} 1.0 & 2.0 & 3.0 & 4.0 \\ \end{bmatrix} \\ & & \begin{bmatrix} {0\phantom{.0}} & {3\phantom{.0}} & {4\phantom{.0}} & {6\phantom{.0}} \\ \end{bmatrix} \\ \end{matrix}\) |
In each example, the top row is the data array and the bottom row is the index array, and it is assumed that the indices are provided in increasing order and that each index appears only once.
3.2. Matrix Formats
Dense and several sparse formats for matrices are discussed in this section.
3.2.1. Dense Format
The dense matrix X
is assumed to be stored in column-major format in memory and is represented by the following parameters.
|
(integer) |
The number of rows in the matrix. |
|
(integer) |
The number of columns in the matrix. |
|
(integer) |
The leading dimension of |
|
(pointer) |
Points to the data array containing the matrix elements. It is assumed that enough storage is allocated for |
For example, m×n
dense matrix X
with leading dimension ldX
can be stored with one-based indexing as shown.
\(\begin{bmatrix} X_{1,1} & X_{1,2} & \cdots & X_{1,n} \\ X_{2,1} & X_{2,2} & \cdots & X_{2,n} \\ \vdots & \vdots & \ddots & \vdots \\ X_{m,1} & X_{m,2} & \cdots & X_{m,n} \\ \vdots & \vdots & \ddots & \vdots \\ X_{ldX,1} & X_{ldX,2} & \cdots & X_{ldX,n} \\ \end{bmatrix}\) |
Its elements are arranged linearly in memory in the order below.
\(\begin{bmatrix} X_{1,1} & X_{2,1} & \cdots & X_{m,1} & \cdots & X_{ldX,1} & \cdots & X_{1,n} & X_{2,n} & \cdots & X_{m,n} & \cdots & X_{ldX,n} \\ \end{bmatrix}\) |
Note
This format and notation are similar to those used in the NVIDIA CUDA cuBLAS library.
3.2.2. Coordinate Format (COO)
The m×n
sparse matrix A
is represented in COO format by the following parameters.
|
(integer) |
The number of nonzero elements in the matrix. |
|
(pointer) |
Points to the data array of length |
|
(pointer) |
Points to the integer array of length |
|
(pointer) |
Points to the integer array of length |
A sparse matrix in COO format is assumed to be stored in row-major format. Each COO entry consists of a row, column pair. The COO format is assumed to be sorted by row. Both sorted and unsorted column indices are supported.
For example, consider the following \(4 \times 5\) matrix A
.
\(\begin{bmatrix} 1.0 & 4.0 & 0.0 & 0.0 & 0.0 \\ 0.0 & 2.0 & 3.0 & 0.0 & 0.0 \\ 5.0 & 0.0 & 0.0 & 7.0 & 8.0 \\ 0.0 & 0.0 & 9.0 & 0.0 & 6.0 \\ \end{bmatrix}\) |
It is stored in COO format with zero-based indexing this way.
\(\begin{matrix} \text{cooValA} & = & \begin{bmatrix} 1.0 & 4.0 & 2.0 & 3.0 & 5.0 & 7.0 & 8.0 & 9.0 & 6.0 \\ \end{bmatrix} \\ \text{cooRowIndA} & = & \begin{bmatrix} {0\phantom{.0}} & {0\phantom{.0}} & {1\phantom{.0}} & {1\phantom{.0}} & {2\phantom{.0}} & {2\phantom{.0}} & {2\phantom{.0}} & {3\phantom{.0}} & {3\phantom{.0}} \\ \end{bmatrix} \\ \text{cooColIndA} & = & \begin{bmatrix} {0\phantom{.0}} & {1\phantom{.0}} & {1\phantom{.0}} & {2\phantom{.0}} & {0\phantom{.0}} & {3\phantom{.0}} & {4\phantom{.0}} & {2\phantom{.0}} & {4\phantom{.0}} \\ \end{bmatrix} \\ \end{matrix}\) |
In the COO format with one-based indexing, it is stored as shown.
\(\begin{matrix} \text{cooValA} & = & \begin{bmatrix} 1.0 & 4.0 & 2.0 & 3.0 & 5.0 & 7.0 & 8.0 & 9.0 & 6.0 \\ \end{bmatrix} \\ \text{cooRowIndA} & = & \begin{bmatrix} {1\phantom{.0}} & {1\phantom{.0}} & {2\phantom{.0}} & {2\phantom{.0}} & {3\phantom{.0}} & {3\phantom{.0}} & {3\phantom{.0}} & {4\phantom{.0}} & {4\phantom{.0}} \\ \end{bmatrix} \\ \text{cooColIndA} & = & \begin{bmatrix} {1\phantom{.0}} & {2\phantom{.0}} & {2\phantom{.0}} & {3\phantom{.0}} & {1\phantom{.0}} & {4\phantom{.0}} & {5\phantom{.0}} & {3\phantom{.0}} & {5\phantom{.0}} \\ \end{bmatrix} \\ \end{matrix}\) |
3.2.3. Compressed Sparse Row Format (CSR)
The only way the CSR differs from the COO format is that the array containing the row indices is compressed in CSR format. The m×n
sparse matrix A
is represented in CSR format by the following parameters.
|
(integer) |
The number of nonzero elements in the matrix. |
|
(pointer) |
Points to the data array of length |
|
(pointer) |
Points to the integer array of length |
|
(pointer) |
Points to the integer array of length |
Sparse matrices in CSR format are assumed to be stored in row-major CSR format. Both sorted and unsorted column indices are supported.
Consider again the \(4 \times 5\) matrixA
.
\(\begin{bmatrix} 1.0 & 4.0 & 0.0 & 0.0 & 0.0 \\ 0.0 & 2.0 & 3.0 & 0.0 & 0.0 \\ 5.0 & 0.0 & 0.0 & 7.0 & 8.0 \\ 0.0 & 0.0 & 9.0 & 0.0 & 6.0 \\ \end{bmatrix}\) |
It is stored in CSR format with zero-based indexing as shown.
\(\begin{matrix} \text{csrValA} & = & \begin{bmatrix} 1.0 & 4.0 & 2.0 & 3.0 & 5.0 & 7.0 & 8.0 & 9.0 & 6.0 \\ \end{bmatrix} \\ \text{csrRowPtrA} & = & \begin{bmatrix} {0\phantom{.0}} & {2\phantom{.0}} & {4\phantom{.0}} & {7\phantom{.0}} & {9\phantom{.0}} \\ \end{bmatrix} \\ \text{csrColIndA} & = & \begin{bmatrix} {0\phantom{.0}} & {1\phantom{.0}} & {1\phantom{.0}} & {2\phantom{.0}} & {0\phantom{.0}} & {3\phantom{.0}} & {4\phantom{.0}} & {2\phantom{.0}} & {4\phantom{.0}} \\ \end{bmatrix} \\ \end{matrix}\) |
This is how it is stored in CSR format with one-based indexing.
\(\begin{matrix} \text{csrValA} & = & \begin{bmatrix} 1.0 & 4.0 & 2.0 & 3.0 & 5.0 & 7.0 & 8.0 & 9.0 & 6.0 \\ \end{bmatrix} \\ \text{csrRowPtrA} & = & \begin{bmatrix} {1\phantom{.0}} & {3\phantom{.0}} & {5\phantom{.0}} & {8\phantom{.0}} & {10\phantom{.0}} \\ \end{bmatrix} \\ \text{csrColIndA} & = & \begin{bmatrix} {1\phantom{.0}} & {2\phantom{.0}} & {2\phantom{.0}} & {3\phantom{.0}} & {1\phantom{.0}} & {4\phantom{.0}} & {5\phantom{.0}} & {3\phantom{.0}} & {5\phantom{.0}} \\ \end{bmatrix} \\ \end{matrix}\) |
3.2.4. Compressed Sparse Column Format (CSC)
The CSC format is different from the COO format in two ways: the matrix is stored in column-major format, and the array containing the column indices is compressed in CSC format. The m×n
matrix A
is represented in CSC format by the following parameters.
|
(integer) |
The number of nonzero elements in the matrix. |
|
(pointer) |
Points to the data array of length |
|
(pointer) |
Points to the integer array of length |
|
(pointer) |
Points to the integer array of length |
Note
The matrix A
in CSR format has exactly the same memory layout as its transpose in CSC format (and vice versa).
For example, consider once again the \(4 \times 5\) matrix A
.
\(\begin{bmatrix} 1.0 & 4.0 & 0.0 & 0.0 & 0.0 \\ 0.0 & 2.0 & 3.0 & 0.0 & 0.0 \\ 5.0 & 0.0 & 0.0 & 7.0 & 8.0 \\ 0.0 & 0.0 & 9.0 & 0.0 & 6.0 \\ \end{bmatrix}\) |
It is stored in CSC format with zero-based indexing this way.
\(\begin{matrix} \text{cscValA} & = & \begin{bmatrix} 1.0 & 5.0 & 4.0 & 2.0 & 3.0 & 9.0 & 7.0 & 8.0 & 6.0 \\ \end{bmatrix} \\ \text{cscRowIndA} & = & \begin{bmatrix} {0\phantom{.0}} & {2\phantom{.0}} & {0\phantom{.0}} & {1\phantom{.0}} & {1\phantom{.0}} & {3\phantom{.0}} & {2\phantom{.0}} & {2\phantom{.0}} & {3\phantom{.0}} \\ \end{bmatrix} \\ \text{cscColPtrA} & = & \begin{bmatrix} {0\phantom{.0}} & {2\phantom{.0}} & {4\phantom{.0}} & {6\phantom{.0}} & {7\phantom{.0}} & {9\phantom{.0}} \\ \end{bmatrix} \\ \end{matrix}\) |
In CSC format with one-based indexing, this is how it is stored.
\(\begin{matrix} \text{cscValA} & = & \begin{bmatrix} 1.0 & 5.0 & 4.0 & 2.0 & 3.0 & 9.0 & 7.0 & 8.0 & 6.0 \\ \end{bmatrix} \\ \text{cscRowIndA} & = & \begin{bmatrix} {1\phantom{.0}} & {3\phantom{.0}} & {1\phantom{.0}} & {2\phantom{.0}} & {2\phantom{.0}} & {4\phantom{.0}} & {3\phantom{.0}} & {3\phantom{.0}} & {4\phantom{.0}} \\ \end{bmatrix} \\ \text{cscColPtrA} & = & \begin{bmatrix} {1\phantom{.0}} & {3\phantom{.0}} & {5\phantom{.0}} & {7\phantom{.0}} & {8\phantom{.0}} & {10\phantom{.0}} \\ \end{bmatrix} \\ \end{matrix}\) |
Each pair of row and column indices appears only once.
3.2.5. Block Compressed Sparse Row Format (BSR)
The only difference between the CSR and BSR formats is the format of the storage element. The former stores primitive data types (single
, double
, cuComplex
, and cuDoubleComplex
) whereas the latter stores a two-dimensional square block of primitive data types. The dimension of the square block is \(blockDim\) . The m×n
sparse matrix A
is equivalent to a block sparse matrix \(A_{b}\) with \(mb = \frac{m + blockDim - 1}{blockDim}\) block rows and \(nb = \frac{n + blockDim - 1}{blockDim}\) block columns. If \(m\) or \(n\) is not multiple of \(blockDim\) , then zeros are filled into \(A_{b}\) .
A
is represented in BSR format by the following parameters.
|
(integer) |
Block dimension of matrix |
|
(integer) |
The number of block rows of |
|
(integer) |
The number of block columns of |
|
(integer) |
The number of nonzero blocks in the matrix. |
|
(pointer) |
Points to the data array of length \(nnzb \ast blockDim^{2}\) that holds all elements of nonzero blocks of |
|
(pointer) |
Points to the integer array of length |
|
(pointer) |
Points to the integer array of length |
As with CSR format, (row, column) indices of BSR are stored in row-major order. The index arrays are first sorted by row indices and then within the same row by column indices.
For example, consider again the 4×5
matrix A
.
\(\begin{bmatrix} 1.0 & 4.0 & 0.0 & 0.0 & 0.0 \\ 0.0 & 2.0 & 3.0 & 0.0 & 0.0 \\ 5.0 & 0.0 & 0.0 & 7.0 & 8.0 \\ 0.0 & 0.0 & 9.0 & 0.0 & 6.0 \\ \end{bmatrix}\) |
If \(blockDim\) is equal to 2, then \(mb\) is 2, \(nb\) is 3, and matrix A
is split into 2×3
block matrix \(A_{b}\) . The dimension of \(A_{b}\) is 4×6
, slightly bigger than matrix \(A\) , so zeros are filled in the last column of \(A_{b}\) . The element-wise view of \(A_{b}\) is this.
\(\begin{bmatrix} 1.0 & 4.0 & 0.0 & 0.0 & 0.0 & 0.0 \\ 0.0 & 2.0 & 3.0 & 0.0 & 0.0 & 0.0 \\ 5.0 & 0.0 & 0.0 & 7.0 & 8.0 & 0.0 \\ 0.0 & 0.0 & 9.0 & 0.0 & 6.0 & 0.0 \\ \end{bmatrix}\) |
Based on zero-based indexing, the block-wise view of \(A_{b}\) can be represented as follows.
\(A_{b} = \begin{bmatrix} A_{00} & A_{01} & A_{02} \\ A_{10} & A_{11} & A_{12} \\ \end{bmatrix}\) |
The basic element of BSR is a nonzero \(A_{ij}\) block, one that contains at least one nonzero element of A
. Five of six blocks are nonzero in \(A_{b}\) .
\(A_{00} = \begin{bmatrix} 1 & 4 \\ 0 & 2 \\ \end{bmatrix},A_{01} = \begin{bmatrix} 0 & 0 \\ 3 & 0 \\ \end{bmatrix},A_{10} = \begin{bmatrix} 5 & 0 \\ 0 & 0 \\ \end{bmatrix},A_{11} = \begin{bmatrix} 0 & 7 \\ 9 & 0 \\ \end{bmatrix},A_{12} = \begin{bmatrix} 8 & 0 \\ 6 & 0 \\ \end{bmatrix}\) |
BSR format only stores the information of nonzero blocks, including block indices \((i,j)\) and values \(A_{ij}\) . Also row indices are compressed in CSR format.
\(\begin{matrix} \text{bsrValA} & = & \begin{bmatrix} A_{00} & A_{01} & A_{10} & A_{11} & A_{12} \\ \end{bmatrix} \\ \text{bsrRowPtrA} & = & \begin{bmatrix} {0\phantom{.0}} & {2\phantom{.0}} & 5 \\ \end{bmatrix} \\ \text{bsrColIndA} & = & \begin{bmatrix} {0\phantom{.0}} & {1\phantom{.0}} & {0\phantom{.0}} & {1\phantom{.0}} & 2 \\ \end{bmatrix} \\ \end{matrix}\) |
There are two ways to arrange the data element of block \(A_{ij}\) : row-major order and column-major order. Under column-major order, the physical storage of bsrValA
is this.
\(\begin{matrix} {bsrValA = \lbrack 1\phantom{.0}0\phantom{.0}4\phantom{.0}2\phantom{.0}} & {0\phantom{.0}3\phantom{.0}0\phantom{.0}0\phantom{.0}} & {5\phantom{.0}0\phantom{.0}0\phantom{.0}0\phantom{.0}} & {0\phantom{.0}9\phantom{.0}7\phantom{.0}0\phantom{.0}} & {8\phantom{.0}6\phantom{.0}0\phantom{.0}0\phantom{.0}\rbrack} \\ \end{matrix}\) |
Under row-major order, the physical storage of bsrValA
is this.
\(\begin{matrix} {bsrValA = \lbrack 1\phantom{.0}4\phantom{.0}0\phantom{.0}2\phantom{.0}} & {0\phantom{.0}0\phantom{.0}3\phantom{.0}0\phantom{.0}} & {5\phantom{.0}0\phantom{.0}0\phantom{.0}0\phantom{.0}} & {0\phantom{.0}7\phantom{.0}9\phantom{.0}0\phantom{.0}} & {8\phantom{.0}0\phantom{.0}6\phantom{.0}0\phantom{.0}\rbrack} \\ \end{matrix}\) |
Similarly, in BSR format with one-based indexing and column-major order, A
can be represented by the following.
\(A_{b} = \begin{bmatrix} A_{11} & A_{12} & A_{13} \\ A_{21} & A_{22} & A_{23} \\ \end{bmatrix}\) |
\(\begin{matrix} {bsrValA = \lbrack 1\phantom{.0}0\phantom{.0}4\phantom{.0}2\phantom{.0}} & {0\phantom{.0}3\phantom{.0}0\phantom{.0}0\phantom{.0}} & {5\phantom{.0}0\phantom{.0}0\phantom{.0}0\phantom{.0}} & {0\phantom{.0}9\phantom{.0}7\phantom{.0}0\phantom{.0}} & {8\phantom{.0}6\phantom{.0}0\phantom{.0}0\phantom{.0}\rbrack} \\ \end{matrix}\) |
\(\begin{matrix} \text{bsrRowPtrA} & = & \begin{bmatrix} {1\phantom{.0}} & {3\phantom{.0}} & 6 \\ \end{bmatrix} \\ \text{bsrColIndA} & = & \begin{bmatrix} {1\phantom{.0}} & {2\phantom{.0}} & {1\phantom{.0}} & {2\phantom{.0}} & 3 \\ \end{bmatrix} \\ \end{matrix}\) |
Note
The general BSR format has two parameters, rowBlockDim
and colBlockDim
. rowBlockDim
is number of rows within a block and colBlockDim
is number of columns within a block. If rowBlockDim=colBlockDim
, general BSR format is the same as BSR format. If rowBlockDim=colBlockDim=1
, general BSR format is the same as CSR format. The conversion routine gebsr2gebsr is used to do conversion among CSR, BSR and general BSR.
Note
In the cuSPARSE Library, the storage format of blocks in BSR format can be column-major or row-major, independently of the base index. However, if the developer uses BSR format from the Math Kernel Library (MKL) and wants to directly interface with the cuSPARSE Library, then cusparseDirection_t
CUSPARSE_DIRECTION_COLUMN
should be used if the base index is one; otherwise, cusparseDirection_t
CUSPARSE_DIRECTION_ROW
should be used.
3.2.6. Extended BSR Format (BSRX)
BSRX is the same as the BSR format, but the array bsrRowPtrA
is separated into two parts. The first nonzero block of each row is still specified by the array bsrRowPtrA
, which is the same as in BSR, but the position next to the last nonzero block of each row is specified by the array bsrEndPtrA
. Briefly, BSRX format is simply like a 4-vector variant of BSR format.
Matrix A
is represented in BSRX format by the following parameters.
|
(integer) |
Block dimension of matrix |
|
(integer) |
The number of block rows of |
|
(integer) |
The number of block columns of |
|
(integer) |
number of nonzero blocks in the matrix |
|
(pointer) |
Points to the data array of length \(nnzb \ast blockDim^{2}\) that holds all the elements of the nonzero blocks of |
|
(pointer) |
Points to the integer array of length |
|
(pointer) |
Points to the integer array of length |
|
(pointer) |
Points to the integer array of length |
A simple conversion between BSR and BSRX can be done as follows. Suppose the developer has a 2×3
block sparse matrix \(A_{b}\) represented as shown.
\(A_{b} = \begin{bmatrix} A_{00} & A_{01} & A_{02} \\ A_{10} & A_{11} & A_{12} \\ \end{bmatrix}\) |
Assume it has this BSR format.
\(\begin{matrix} \text{bsrValA of BSR} & = & \begin{bmatrix} A_{00} & A_{01} & A_{10} & A_{11} & A_{12} \\ \end{bmatrix} \\ \text{bsrRowPtrA of BSR} & = & \begin{bmatrix} {0\phantom{.0}} & {2\phantom{.0}} & 5 \\ \end{bmatrix} \\ \text{bsrColIndA of BSR} & = & \begin{bmatrix} {0\phantom{.0}} & {1\phantom{.0}} & {0\phantom{.0}} & {1\phantom{.0}} & 2 \\ \end{bmatrix} \\ \end{matrix}\) |
The bsrRowPtrA
of the BSRX format is simply the first two elements of the bsrRowPtrA
BSR format. The bsrEndPtrA
of BSRX format is the last two elements of the bsrRowPtrA
of BSR format.
\(\begin{matrix} \text{bsrRowPtrA of BSRX} & = & \begin{bmatrix} {0\phantom{.0}} & 2 \\ \end{bmatrix} \\ \text{bsrEndPtrA of BSRX} & = & \begin{bmatrix} {2\phantom{.0}} & 5 \\ \end{bmatrix} \\ \end{matrix}\) |
The advantage of the BSRX format is that the developer can specify a submatrix in the original BSR format by modifying bsrRowPtrA
and bsrEndPtrA
while keeping bsrColIndA
and bsrValA
unchanged.
For example, to create another block matrix \(\widetilde{A} = \begin{bmatrix}
O & O & O \\
O & A_{11} & O \\
\end{bmatrix}\) that is slightly different from \(A\) , the developer can keep bsrColIndA
and bsrValA
, but reconstruct \(\widetilde{A}\) by properly setting of bsrRowPtrA
and bsrEndPtrA
. The following 4-vector characterizes \(\widetilde{A}\) .
\(\begin{matrix} {\text{bsrValA of }\widetilde{A}} & = & \begin{bmatrix} A_{00} & A_{01} & A_{10} & A_{11} & A_{12} \\ \end{bmatrix} \\ {\text{bsrColIndA of }\widetilde{A}} & = & \begin{bmatrix} {0\phantom{.0}} & {1\phantom{.0}} & {0\phantom{.0}} & {1\phantom{.0}} & 2 \\ \end{bmatrix} \\ {\text{bsrRowPtrA of }\widetilde{A}} & = & \begin{bmatrix} {0\phantom{.0}} & 3 \\ \end{bmatrix} \\ {\text{bsrEndPtrA of }\widetilde{A}} & = & \begin{bmatrix} {0\phantom{.0}} & 4 \\ \end{bmatrix} \\ \end{matrix}\) |
4. cuSPARSE Types Reference
4.1. Data types
The float
, double
, cuComplex
, and cuDoubleComplex
data types are supported. The first two are standard C data types, while the last two are exported from cuComplex.h
.
4.2. cusparseStatus_t
This data type represents the status returned by the library functions and it can have the following values
Value |
Description |
---|---|
|
The operation completed successfully |
|
The cuSPARSE library was not initialized. This is usually caused by the lack of a prior call, an error in the CUDA Runtime API called by the cuSPARSE routine, or an error in the hardware setup To correct: call The error also applies to generic APIs ( Generic APIs reference) for indicating a matrix/vector descriptor not initialized |
|
Resource allocation failed inside the cuSPARSE library. This is usually caused by a device memory allocation ( To correct: prior to the function call, deallocate previously allocated memory as much as possible |
|
An unsupported value or parameter was passed to the function (a negative vector size, for example) To correct: ensure that all the parameters being passed have valid values |
|
The function requires a feature absent from the device architecture To correct: compile and run the application on a device with appropriate compute capability |
|
The GPU program failed to execute. This is often caused by a launch failure of the kernel on the GPU, which can be caused by multiple reasons To correct: check that the hardware, an appropriate version of the driver, and the cuSPARSE library are correctly installed |
|
An internal cuSPARSE operation failed To correct: check that the hardware, an appropriate version of the driver, and the cuSPARSE library are correctly installed. Also, check that the memory passed as a parameter to the routine is not being deallocated prior to the routine completion |
|
The matrix type is not supported by this function. This is usually caused by passing an invalid matrix descriptor to the function To correct: check that the fields in |
|
The operation or data type combination is currently not supported by the function |
|
The resources for the computation, such as GPU global or shared memory, are not sufficient to complete the operation. The error can also indicate that the current computation mode (e.g. bit size of sparse matrix indices) does not allow to handle the given input |
4.3. cusparseHandle_t
This is a pointer type to an opaque cuSPARSE context, which the user must initialize by calling prior to calling cusparseCreate()
any other library function. The handle created and returned by cusparseCreate()
must be passed to every cuSPARSE function.
4.4. cusparsePointerMode_t
This type indicates whether the scalar values are passed by reference on the host or device. It is important to point out that if several scalar values are passed by reference in the function call, all of them will conform to the same single pointer mode. The pointer mode can be set and retrieved using cusparseSetPointerMode()
and cusparseGetPointerMode()
routines, respectively.
Value |
Meaning |
---|---|
|
the scalars are passed by reference on the host. |
|
the scalars are passed by reference on the device. |
4.5. cusparseOperation_t
This type indicates which operations need to be performed with the sparse matrix.
Value |
Meaning |
---|---|
|
the non-transpose operation is selected. |
|
the transpose operation is selected. |
|
the conjugate transpose operation is selected. |
4.6. cusparseAction_t
This type indicates whether the operation is performed only on indices or on data and indices.
Value |
Meaning |
---|---|
|
the operation is performed only on indices. |
|
the operation is performed on data and indices. |
4.7. cusparseDirection_t
This type indicates whether the elements of a dense matrix should be parsed by rows or by columns (assuming column-major storage in memory of the dense matrix) in function cusparse[S|D|C|Z]nnz. Besides storage format of blocks in BSR format is also controlled by this type.
Value |
Meaning |
---|---|
|
the matrix should be parsed by rows. |
|
the matrix should be parsed by columns. |
4.8. cusparseMatDescr_t
This structure is used to describe the shape and properties of a matrix.
typedef struct {
cusparseMatrixType_t MatrixType;
cusparseFillMode_t FillMode;
cusparseDiagType_t DiagType;
cusparseIndexBase_t IndexBase;
} cusparseMatDescr_t;
4.8.1. cusparseDiagType_t
This type indicates if the matrix diagonal entries are unity. The diagonal elements are always assumed to be present, but if CUSPARSE_DIAG_TYPE_UNIT
is passed to an API routine, then the routine assumes that all diagonal entries are unity and will not read or modify those entries. Note that in this case the routine assumes the diagonal entries are equal to one, regardless of what those entries are actually set to in memory.
Value |
Meaning |
---|---|
|
the matrix diagonal has non-unit elements. |
|
the matrix diagonal has unit elements. |
4.8.2. cusparseFillMode_t
This type indicates if the lower or upper part of a matrix is stored in sparse storage.
Value |
Meaning |
---|---|
|
the lower triangular part is stored. |
|
the upper triangular part is stored. |
4.8.3. cusparseIndexBase_t
This type indicates if the base of the matrix indices is zero or one.
Value |
Meaning |
---|---|
|
the base index is zero. |
|
the base index is one. |
4.8.4. cusparseMatrixType_t
This type indicates the type of matrix stored in sparse storage. Notice that for symmetric, Hermitian and triangular matrices only their lower or upper part is assumed to be stored.
The whole idea of matrix type and fill mode is to keep minimum storage for symmetric/Hermitian matrix, and also to take advantage of symmetric property on SpMV (Sparse Matrix Vector multiplication). To compute y=A*x
when A
is symmetric and only lower triangular part is stored, two steps are needed. First step is to compute y=(L+D)*x
and second step is to compute y=L^T*x + y
. Given the fact that the transpose operation y=L^T*x
is 10x slower than non-transpose version y=L*x
, the symmetric property does not show up any performance gain. It is better for the user to extend the symmetric matrix to a general matrix and apply y=A*x
with matrix type CUSPARSE_MATRIX_TYPE_GENERAL
.
In general, SpMV, preconditioners (incomplete Cholesky or incomplete LU) and triangular solver are combined together in iterative solvers, for example PCG and GMRES. If the user always uses general matrix (instead of symmetric matrix), there is no need to support other than general matrix in preconditioners. Therefore the new routines, [bsr|csr]sv2
(triangular solver), [bsr|csr]ilu02
(incomplete LU) and [bsr|csr]ic02
(incomplete Cholesky), only support matrix type CUSPARSE_MATRIX_TYPE_GENERAL
.
Value |
Meaning |
---|---|
|
the matrix is general. |
|
the matrix is symmetric. |
|
the matrix is Hermitian. |
|
the matrix is triangular. |
4.9. cusparseColorInfo_t
This is a pointer type to an opaque structure holding the information used in csrcolor()
.
4.10. cusparseSolvePolicy_t
This type indicates whether level information is generated and used in csrsv2, csric02, csrilu02, bsrsv2, bsric02 and bsrilu02
.
Value |
Meaning |
---|---|
|
no level information is generated and used. |
|
generate and use level information. |
4.11. bsric02Info_t
This is a pointer type to an opaque structure holding the information used in bsric02_bufferSize()
, bsric02_analysis()
, and bsric02()
.
4.12. bsrilu02Info_t
This is a pointer type to an opaque structure holding the information used in bsrilu02_bufferSize()
, bsrilu02_analysis()
, and bsrilu02()
.
4.13. bsrsm2Info_t
This is a pointer type to an opaque structure holding the information used in bsrsm2_bufferSize()
, bsrsm2_analysis()
, and bsrsm2_solve()
.
4.14. bsrsv2Info_t
This is a pointer type to an opaque structure holding the information used in bsrsv2_bufferSize()
, bsrsv2_analysis()
, and bsrsv2_solve()
.
4.15. csric02Info_t
This is a pointer type to an opaque structure holding the information used in csric02_bufferSize()
, csric02_analysis()
, and csric02()
.
4.16. csrilu02Info_t
This is a pointer type to an opaque structure holding the information used in csrilu02_bufferSize()
, csrilu02_analysis()
, and csrilu02()
.
5. cuSPARSE Management Function Reference
The cuSPARSE functions for managing the library are described in this section.
5.1. cusparseCreate()
cusparseStatus_t
cusparseCreate(cusparseHandle_t *handle)
This function initializes the cuSPARSE library and creates a handle on the cuSPARSE context. It must be called before any other cuSPARSE API function is invoked. It allocates hardware resources necessary for accessing the GPU.
Param. |
In/out |
Meaning |
---|---|---|
|
IN |
The pointer to the handle to the cuSPARSE context |
See cusparseStatus_t for the description of the return status
5.2. cusparseDestroy()
cusparseStatus_t
cusparseDestroy(cusparseHandle_t handle)
This function releases CPU-side resources used by the cuSPARSE library. The release of GPU-side resources may be deferred until the application shuts down.
Param. |
In/out |
Meaning |
---|---|---|
|
IN |
The handle to the cuSPARSE context |
See cusparseStatus_t for the description of the return status
5.3. cusparseGetErrorName()
const char*
cusparseGetErrorString(cusparseStatus_t status)
The function returns the string representation of an error code enum name. If the error code is not recognized, “unrecognized error code” is returned.
Param. |
In/out |
Meaning |
---|---|---|
|
IN |
Error code to convert to string |
|
OUT |
Pointer to a NULL-terminated string |
5.4. cusparseGetErrorString()
const char*
cusparseGetErrorString(cusparseStatus_t status)
Returns the description string for an error code. If the error code is not recognized, “unrecognized error code” is returned.
Param. |
In/out |
Meaning |
---|---|---|
|
IN |
Error code to convert to string |
|
OUT |
Pointer to a NULL-terminated string |
5.5. cusparseGetProperty()
cusparseStatus_t
cusparseGetProperty(libraryPropertyType type,
int* value)
The function returns the value of the requested property. Refer to libraryPropertyType
for supported types.
Param. |
In/out |
Meaning |
---|---|---|
|
IN |
Requested property |
|
OUT |
Value of the requested property |
libraryPropertyType
(defined in library_types.h
):
Value |
Meaning |
---|---|
|
Enumerator to query the major version |
|
Enumerator to query the minor version |
|
Number to identify the patch level |
See cusparseStatus_t for the description of the return status
5.6. cusparseGetVersion()
cusparseStatus_t
cusparseGetVersion(cusparseHandle_t handle,
int* version)
This function returns the version number of the cuSPARSE library.
Param. |
In/out |
Meaning |
---|---|---|
|
IN |
cuSPARSE handle |
|
OUT |
The version number of the library |
See cusparseStatus_t for the description of the return status
5.7. cusparseGetPointerMode()
cusparseStatus_t
cusparseGetPointerMode(cusparseHandlet handle,
cusparsePointerMode_t *mode)
This function obtains the pointer mode used by the cuSPARSE library. Please see the section on the cusparsePointerMode_t
type for more details.
Param. |
In/out |
Meaning |
---|---|---|
|
IN |
The handle to the cuSPARSE context |
|
OUT |
One of the enumerated pointer mode types |
See cusparseStatus_t for the description of the return status
5.8. cusparseSetPointerMode()
cusparseStatus_t
cusparseSetPointerMode(cusparseHandle_t handle,
cusparsePointerMode_t mode)
This function sets the pointer mode used by the cuSPARSE library. The default is for the values to be passed by reference on the host. Please see the section on the cublasPointerMode_t
type for more details.
Param. |
In/out |
Meaning |
---|---|---|
|
IN |
The handle to the cuSPARSE context |
|
IN |
One of the enumerated pointer mode types |
See cusparseStatus_t for the description of the return status
5.9. cusparseGetStream()
cusparseStatus_t
cusparseGetStream(cusparseHandle_t handle, cudaStream_t *streamId)
This function gets the cuSPARSE library stream, which is being used to to execute all calls to the cuSPARSE library functions. If the cuSPARSE library stream is not set, all kernels use the default NULL stream.
Param. |
In/out |
Meaning |
---|---|---|
|
IN |
The handle to the cuSPARSE context |
|
OUT |
The stream used by the library |
See cusparseStatus_t for the description of the return status
5.10. cusparseSetStream()
cusparseStatus_t
cusparseSetStream(cusparseHandle_t handle, cudaStream_t streamId)
This function sets the stream to be used by the cuSPARSE library to execute its routines.
Param. |
In/out |
Meaning |
---|---|---|
|
IN |
The handle to the cuSPARSE context |
|
IN |
The stream to be used by the library |
See cusparseStatus_t for the description of the return status
6. cuSPARSE Logging
cuSPARSE logging mechanism can be enabled by setting the following environment variables before launching the target application:
CUSPARSE_LOG_LEVEL=<level>
- while level is one of the following levels:
0
- Off - logging is disabled (default)1
- Error - only errors will be logged2
- Trace - API calls that launch CUDA kernels will log their parameters and important information3
- Hints - hints that can potentially improve the application’s performance4
- Info - provides general information about the library execution, may contain details about heuristic status5
- API Trace - API calls will log their parameter and important information
CUSPARSE_LOG_MASK=<mask>
- while mask is a combination of the following masks:
0
- Off1
- Error2
- Trace4
- Hints8
- Info16
- API Trace
CUSPARSE_LOG_FILE=<file_name>
- while file name is a path to a logging file. File name may contain %i, that will be replaced with the process id. E.g “<file_name>_%i.log”.
If CUSPARSE_LOG_FILE
is not defined, the log messages are printed to stdout.
Another option is to use the experimental cuSPARSE logging API. See:
Note
The logging mechanism is not available for the legacy APIs.
6.1. cusparseLoggerSetCallback()
cusparseStatus_t
cusparseLoggerSetCallback(cusparseLoggerCallback_t callback)
Experimental: The function sets the logging callback function.
Param. |
In/out |
Meaning |
---|---|---|
|
IN |
Pointer to a callback function |
where cusparseLoggerCallback_t
has the following signature:
void (*cusparseLoggerCallback_t)(int logLevel,
const char* functionName,
const char* message)
Param. |
In/out |
Meaning |
---|---|---|
|
IN |
Selected log level |
|
IN |
The name of the API that logged this message |
|
IN |
The log message |
See cusparseStatus_t for the description of the return status
6.2. cusparseLoggerSetFile()
cusparseStatus_t
cusparseLoggerSetFile(FILE* file)
Experimental: The function sets the logging output file. Note: once registered using this function call, the provided file handle must not be closed unless the function is called again to switch to a different file handle.
Param. |
In/out |
Meaning |
---|---|---|
|
IN |
Pointer to an open file. File should have write permission |
See cusparseStatus_t for the description of the return status
6.3. cusparseLoggerOpenFile()
cusparseStatus_t
cusparseLoggerOpenFile(const char* logFile)
Experimental: The function opens a logging output file in the given path.
Param. |
In/out |
Meaning |
---|---|---|
|
IN |
Path of the logging output file |
See cusparseStatus_t for the description of the return status
6.4. cusparseLoggerSetLevel()
cusparseStatus_t
cusparseLoggerSetLevel(int level)
Experimental: The function sets the value of the logging level. path.
Param. |
In/out |
Meaning |
---|---|---|
|
IN |
Value of the logging level |
See cusparseStatus_t for the description of the return status
6.5. cusparseLoggerSetMask()
cusparseStatus_t
cusparseLoggerSetMask(int mask)
Experimental: The function sets the value of the logging mask.
Param. |
In/out |
Meaning |
---|---|---|
|
IN |
Value of the logging mask |
See cusparseStatus_t for the description of the return status
6.6. cublasLtLoggerForceDisable()
cusparseStatus_t
cublasLtLoggerForceDisable()
Experimental: The function disables logging for the entier run.
See cusparseStatus_t for the description of the return status
7. cuSPARSE Helper Function Reference
The cuSPARSE helper functions are described in this section.
7.1. cusparseCreateColorInfo()
cusparseStatus_t
cusparseCreateColorInfo(cusparseColorInfo_t* info)
This function creates and initializes the cusparseColorInfo_t
structure to default values.
Input
|
the pointer to the |
See cusparseStatus_t for the description of the return status
7.2. cusparseCreateMatDescr()
cusparseStatus_t
cusparseCreateMatDescr(cusparseMatDescr_t *descrA)
This function initializes the matrix descriptor. It sets the fields MatrixType
and IndexBase
to the default values CUSPARSE_MATRIX_TYPE_GENERAL
and CUSPARSE_INDEX_BASE_ZERO
, respectively, while leaving other fields uninitialized.
Input
|
the pointer to the matrix descriptor. |
See cusparseStatus_t for the description of the return status
7.3. cusparseDestroyColorInfo()
cusparseStatus_t
cusparseDestroyColorInfo(cusparseColorInfo_t info)
This function destroys and releases any memory required by the structure.
Input
|
the pointer to the structure of |
See cusparseStatus_t for the description of the return status
7.4. cusparseDestroyMatDescr()
cusparseStatus_t
cusparseDestroyMatDescr(cusparseMatDescr_t descrA)
This function releases the memory allocated for the matrix descriptor.
Input
|
the matrix descriptor. |
See cusparseStatus_t for the description of the return status
7.5. cusparseGetMatDiagType()
cusparseDiagType_t
cusparseGetMatDiagType(const cusparseMatDescr_t descrA)
This function returns the DiagType
field of the matrix descriptor descrA
.
Input
|
the matrix descriptor. |
Returned
|
One of the enumerated diagType types. |
7.6. cusparseGetMatFillMode()
cusparseFillMode_t
cusparseGetMatFillMode(const cusparseMatDescr_t descrA)
This function returns the FillMode
field of the matrix descriptor descrA
.
Input
|
the matrix descriptor. |
Returned
|
One of the enumerated fillMode types. |
7.7. cusparseGetMatIndexBase()
cusparseIndexBase_t
cusparseGetMatIndexBase(const cusparseMatDescr_t descrA)
This function returns the IndexBase
field of the matrix descriptor descrA
.
Input
|
the matrix descriptor. |
Returned
|
One of the enumerated indexBase types. |
7.8. cusparseGetMatType()
cusparseMatrixType_t
cusparseGetMatType(const cusparseMatDescr_t descrA)
This function returns the MatrixType
field of the matrix descriptor descrA
.
Input
|
the matrix descriptor. |
Returned
|
One of the enumerated matrix types. |
7.9. cusparseSetMatDiagType()
cusparseStatus_t
cusparseSetMatDiagType(cusparseMatDescr_t descrA,
cusparseDiagType_t diagType)
This function sets the DiagType
field of the matrix descriptor descrA
.
Input
|
One of the enumerated diagType types. |
Output
|
the matrix descriptor. |
See cusparseStatus_t for the description of the return status
7.10. cusparseSetMatFillMode()
cusparseStatus_t
cusparseSetMatFillMode(cusparseMatDescr_t descrA,
cusparseFillMode_t fillMode)
This function sets the FillMode
field of the matrix descriptor descrA
.
Input
|
One of the enumerated fillMode types. |
Output
|
the matrix descriptor. |
See cusparseStatus_t for the description of the return status
7.11. cusparseSetMatIndexBase()
cusparseStatus_t
cusparseSetMatIndexBase(cusparseMatDescr_t descrA,
cusparseIndexBase_t base)
This function sets the IndexBase
field of the matrix descriptor descrA
.
Input
|
One of the enumerated indexBase types. |
Output
|
the matrix descriptor. |
See cusparseStatus_t for the description of the return status
7.12. cusparseSetMatType()
cusparseStatus_t
cusparseSetMatType(cusparseMatDescr_t descrA, cusparseMatrixType_t type)
This function sets the MatrixType
field of the matrix descriptor descrA
.
Input
|
One of the enumerated matrix types. |
Output
|
the matrix descriptor. |
See cusparseStatus_t for the description of the return status
7.13. cusparseCreateCsric02Info()
cusparseStatus_t
cusparseCreateCsric02Info(csric02Info_t *info);
This function creates and initializes the solve and analysis structure of incomplete Cholesky to default values.
Input
|
the pointer to the solve and analysis structure of incomplete Cholesky. |
See cusparseStatus_t for the description of the return status
7.14. cusparseDestroyCsric02Info()
cusparseStatus_t
cusparseDestroyCsric02Info(csric02Info_t info);
This function destroys and releases any memory required by the structure.
Input
|
the solve |
See cusparseStatus_t for the description of the return status
7.15. cusparseCreateCsrilu02Info()
cusparseStatus_t
cusparseCreateCsrilu02Info(csrilu02Info_t *info);
This function creates and initializes the solve and analysis structure of incomplete LU to default values.
Input
|
the pointer to the solve and analysis structure of incomplete LU. |
See cusparseStatus_t for the description of the return status
7.16. cusparseDestroyCsrilu02Info()
cusparseStatus_t
cusparseDestroyCsrilu02Info(csrilu02Info_t info);
This function destroys and releases any memory required by the structure.
Input
|
the solve |
See cusparseStatus_t for the description of the return status
7.17. cusparseCreateBsrsv2Info()
cusparseStatus_t
cusparseCreateBsrsv2Info(bsrsv2Info_t *info);
This function creates and initializes the solve and analysis structure of bsrsv2 to default values.
Input
|
the pointer to the solve and analysis structure of bsrsv2. |
See cusparseStatus_t for the description of the return status
7.18. cusparseDestroyBsrsv2Info()
cusparseStatus_t
cusparseDestroyBsrsv2Info(bsrsv2Info_t info);
This function destroys and releases any memory required by the structure.
Input
|
the solve |
See cusparseStatus_t for the description of the return status
7.19. cusparseCreateBsrsm2Info()
cusparseStatus_t
cusparseCreateBsrsm2Info(bsrsm2Info_t *info);
This function creates and initializes the solve and analysis structure of bsrsm2 to default values.
Input
|
the pointer to the solve and analysis structure of bsrsm2. |
See cusparseStatus_t for the description of the return status
7.20. cusparseDestroyBsrsm2Info()
cusparseStatus_t
cusparseDestroyBsrsm2Info(bsrsm2Info_t info);
This function destroys and releases any memory required by the structure.
Input
|
the solve |
See cusparseStatus_t for the description of the return status
7.21. cusparseCreateBsric02Info()
cusparseStatus_t
cusparseCreateBsric02Info(bsric02Info_t *info);
This function creates and initializes the solve and analysis structure of block incomplete Cholesky to default values.
Input
|
the pointer to the solve and analysis structure of block incomplete Cholesky. |
See cusparseStatus_t for the description of the return status
7.22. cusparseDestroyBsric02Info()
cusparseStatus_t
cusparseDestroyBsric02Info(bsric02Info_t info);
This function destroys and releases any memory required by the structure.
Input
|
the solve |
See cusparseStatus_t for the description of the return status
7.23. cusparseCreateBsrilu02Info()
cusparseStatus_t
cusparseCreateBsrilu02Info(bsrilu02Info_t *info);
This function creates and initializes the solve and analysis structure of block incomplete LU to default values.
Input
|
the pointer to the solve and analysis structure of block incomplete LU. |
See cusparseStatus_t for the description of the return status
7.24. cusparseDestroyBsrilu02Info()
cusparseStatus_t
cusparseDestroyBsrilu02Info(bsrilu02Info_t info);
This function destroys and releases any memory required by the structure.
Input
|
the solve |
See cusparseStatus_t for the description of the return status
7.25. cusparseCreatePruneInfo()
cusparseStatus_t
cusparseCreatePruneInfo(pruneInfo_t *info);
This function creates and initializes structure of prune
to default values.
Input
|
the pointer to the structure of |
See cusparseStatus_t for the description of the return status
7.26. cusparseDestroyPruneInfo()
cusparseStatus_t
cusparseDestroyPruneInfo(pruneInfo_t info);
This function destroys and releases any memory required by the structure.
Input
|
the structure of |
See cusparseStatus_t for the description of the return status
8. cuSPARSE Level 2 Function Reference
This chapter describes the sparse linear algebra functions that perform operations between sparse matrices and dense vectors.
In particular, the solution of sparse triangular linear systems is implemented in two phases. First, during the analysis phase, the sparse triangular matrix is analyzed to determine the dependencies between its elements by calling the appropriate csrsv2_analysis()
function. The analysis is specific to the sparsity pattern of the given matrix and to the selected cusparseOperation_t
type. The information from the analysis phase is stored in the parameter of type csrsv2Info_t
that has been initialized previously with a call to cusparseCreateCsrsv2Info()
.
Second, during the solve phase, the given sparse triangular linear system is solved using the information stored in the csrsv2Info_t
parameter by calling the appropriate csrsv2_solve()
function. The solve phase may be performed multiple times with different right-hand sides, while the analysis phase needs to be performed only once. This is especially useful when a sparse triangular linear system must be solved for a set of different right-hand sides one at a time, while its coefficient matrix remains the same.
Finally, once all the solves have completed, the opaque data structure pointed to by the csrsv2Info_t
parameter can be released by calling cusparseDestroyCsrsv2Info()
8.1. cusparse<t>bsrmv()
cusparseStatus_t
cusparseSbsrmv(cusparseHandle_t handle,
cusparseDirection_t dir,
cusparseOperation_t trans,
int mb,
int nb,
int nnzb,
const float* alpha,
const cusparseMatDescr_t descr,
const float* bsrVal,
const int* bsrRowPtr,
const int* bsrColInd,
int blockDim,
const float* x,
const float* beta,
float* y)
cusparseStatus_t
cusparseDbsrmv(cusparseHandle_t handle,
cusparseDirection_t dir,
cusparseOperation_t trans,
int mb,
int nb,
int nnzb,
const double* alpha,
const cusparseMatDescr_t descr,
const double* bsrVal,
const int* bsrRowPtr,
const int* bsrColInd,
int blockDim,
const double* x,
const double* beta,
double* y)
cusparseStatus_t
cusparseCbsrmv(cusparseHandle_t handle,
cusparseDirection_t dir,
cusparseOperation_t trans,
int mb,
int nb,
int nnzb,
const cuComplex* alpha,
const cusparseMatDescr_t descr,
const cuComplex* bsrVal,
const int* bsrRowPtr,
const int* bsrColInd,
int blockDim,
const cuComplex* x,
const cuComplex* beta,
cuComplex* y)
cusparseStatus_t
cusparseZbsrmv(cusparseHandle_t handle,
cusparseDirection_t dir,
cusparseOperation_t trans,
int mb,
int nb,
int nnzb,
const cuDoubleComplex* alpha,
const cusparseMatDescr_t descr,
const cuDoubleComplex* bsrVal,
const int* bsrRowPtr,
const int* bsrColInd,
int blockDim,
const cuDoubleComplex* x,
const cuDoubleComplex* beta,
cuDoubleComplex* y)
This function performs the matrix-vector operation
\(\text{y} = \alpha \ast \text{op}(A) \ast \text{x} + \beta \ast \text{y}\) |
where \(A\text{ is an }(mb \ast blockDim) \times (nb \ast blockDim)\) sparse matrix that is defined in BSR storage format by the three arrays bsrVal
, bsrRowPtr
, and bsrColInd
); x
and y
are vectors; \(\alpha\text{ and }\beta\) are scalars; and
\(\text{op}(A) = \begin{cases} A & \text{if trans == CUSPARSE_OPERATION_NON_TRANSPOSE} \\ A^{T} & \text{if trans == CUSPARSE_OPERATION_TRANSPOSE} \\ A^{H} & \text{if trans == CUSPARSE_OPERATION_CONJUGATE_TRANSPOSE} \\ \end{cases}\)
bsrmv()
has the following properties:
The routine requires no extra storage
The routine supports asynchronous execution
The routine supports CUDA graph capture
Several comments on bsrmv()
:
Only
blockDim > 1
is supportedOnly
CUSPARSE_OPERATION_NON_TRANSPOSE
is supported, that is
\(\text{y} = \alpha \ast A \ast \text{x} + \beta{} \ast \text{y}\) |
Only
CUSPARSE_MATRIX_TYPE_GENERAL
is supported.The size of vector
x
should be \((nb \ast blockDim)\) at least, and the size of vectory
should be \((mb \ast blockDim)\) at least; otherwise, the kernel may returnCUSPARSE_STATUS_EXECUTION_FAILED
because of an out-of-bounds array.
For example, suppose the user has a CSR format and wants to try bsrmv()
, the following code demonstrates how to use csr2bsr()
conversion and bsrmv()
multiplication in single precision.
// Suppose that A is m x n sparse matrix represented by CSR format,
// hx is a host vector of size n, and hy is also a host vector of size m.
// m and n are not multiple of blockDim.
// step 1: transform CSR to BSR with column-major order
int base, nnz;
int nnzb;
cusparseDirection_t dirA = CUSPARSE_DIRECTION_COLUMN;
int mb = (m + blockDim-1)/blockDim;
int nb = (n + blockDim-1)/blockDim;
cudaMalloc((void**)&bsrRowPtrC, sizeof(int) *(mb+1));
cusparseXcsr2bsrNnz(handle, dirA, m, n,
descrA, csrRowPtrA, csrColIndA, blockDim,
descrC, bsrRowPtrC, &nnzb);
cudaMalloc((void**)&bsrColIndC, sizeof(int)*nnzb);
cudaMalloc((void**)&bsrValC, sizeof(float)*(blockDim*blockDim)*nnzb);
cusparseScsr2bsr(handle, dirA, m, n,
descrA, csrValA, csrRowPtrA, csrColIndA, blockDim,
descrC, bsrValC, bsrRowPtrC, bsrColIndC);
// step 2: allocate vector x and vector y large enough for bsrmv
cudaMalloc((void**)&x, sizeof(float)*(nb*blockDim));
cudaMalloc((void**)&y, sizeof(float)*(mb*blockDim));
cudaMemcpy(x, hx, sizeof(float)*n, cudaMemcpyHostToDevice);
cudaMemcpy(y, hy, sizeof(float)*m, cudaMemcpyHostToDevice);
// step 3: perform bsrmv
cusparseSbsrmv(handle, dirA, transA, mb, nb, nnzb, &alpha,
descrC, bsrValC, bsrRowPtrC, bsrColIndC, blockDim, x, &beta, y);
Input
|
handle to the cuSPARSE library context. |
|
storage format of blocks, either |
|
the operation \(\text{op}(A)\) . Only |
|
number of block rows of matrix \(A\). |
|
number of block columns of matrix \(A\). |
|
number of nonzero blocks of matrix \(A\). |
|
<type> scalar used for multiplication. |
|
the descriptor of matrix \(A\). The supported matrix type is |
|
<type> array of |
|
integer array of |
|
integer array of |
|
block dimension of sparse matrix \(A\), larger than zero. |
|
<type> vector of \(nb \ast blockDim\) elements. |
|
<type> scalar used for multiplication. If |
|
<type> vector of \(mb \ast blockDim\) elements. |
Output
|
<type> updated vector. |
See cusparseStatus_t for the description of the return status.
8.2. cusparse<t>bsrxmv()
cusparseStatus_t
cusparseSbsrxmv(cusparseHandle_t handle,
cusparseDirection_t dir,
cusparseOperation_t trans,
int sizeOfMask,
int mb,
int nb,
int nnzb,
const float* alpha,
const cusparseMatDescr_t descr,
const float* bsrVal,
const int* bsrMaskPtr,
const int* bsrRowPtr,
const int* bsrEndPtr,
const int* bsrColInd,
int blockDim,
const float* x,
const float* beta,
float* y)
cusparseStatus_t
cusparseDbsrxmv(cusparseHandle_t handle,
cusparseDirection_t dir,
cusparseOperation_t trans,
int sizeOfMask,
int mb,
int nb,
int nnzb,
const double* alpha,
const cusparseMatDescr_t descr,
const double* bsrVal,
const int* bsrMaskPtr,
const int* bsrRowPtr,
const int* bsrEndPtr,
const int* bsrColInd,
int blockDim,
const double* x,
const double* beta,
double* y)
cusparseStatus_t
cusparseCbsrxmv(cusparseHandle_t handle,
cusparseDirection_t dir,
cusparseOperation_t trans,
int sizeOfMask,
int mb,
int nb,
int nnzb,
const cuComplex* alpha,
const cusparseMatDescr_t descr,
const cuComplex* bsrVal,
const int* bsrMaskPtr,
const int* bsrRowPtr,
const int* bsrEndPtr,
const int* bsrColInd,
int blockDim,
const cuComplex* x,
const cuComplex* beta,
cuComplex* y)
cusparseStatus_t
cusparseZbsrxmv(cusparseHandle_t handle,
cusparseDirection_t dir,
cusparseOperation_t trans,
int sizeOfMask,
int mb,
int nb,
int nnzb,
const cuDoubleComplex* alpha,
const cusparseMatDescr_t descr,
const cuDoubleComplex* bsrVal,
const int* bsrMaskPtr,
const int* bsrRowPtr,
const int* bsrEndPtr,
const int* bsrColInd,
int blockDim,
const cuDoubleComplex* x,
const cuDoubleComplex* beta,
cuDoubleComplex* y)
This function performs a bsrmv
and a mask operation
\(\text{y(mask)} = (\alpha \ast \text{op}(A) \ast \text{x} + \beta \ast \text{y})\text{(mask)}\) |
where \(A\text{ is an }(mb \ast blockDim) \times (nb \ast blockDim)\) sparse matrix that is defined in BSRX storage format by the four arrays bsrVal
, bsrRowPtr
, bsrEndPtr
, and bsrColInd
); x
and y
are vectors; \(\alpha\text{~and~}\beta\) are scalars; and
\(\text{op}(A) = \begin{cases} A & \text{if trans == CUSPARSE_OPERATION_NON_TRANSPOSE} \\ A^{T} & \text{if trans == CUSPARSE_OPERATION_TRANSPOSE} \\ A^{H} & \text{if trans == CUSPARSE_OPERATION_CONJUGATE_TRANSPOSE} \\ \end{cases}\)
The mask operation is defined by array bsrMaskPtr
which contains updated block row indices of \(y\) . If row \(i\) is not specified in bsrMaskPtr
, then bsrxmv()
does not touch row block \(i\) of \(A\) and \(y\) .
For example, consider the \(2 \times 3\) block matrix \(A\):
\(\begin{matrix} {A = \begin{bmatrix} A_{11} & A_{12} & O \\ A_{21} & A_{22} & A_{23} \\ \end{bmatrix}} \\ \end{matrix}\) |
and its one-based BSR format (three vector form) is
\(\begin{matrix} \text{bsrVal} & = & \begin{bmatrix} A_{11} & A_{12} & A_{21} & A_{22} & A_{23} \\ \end{bmatrix} \\ \text{bsrRowPtr} & = & \begin{bmatrix} {1\phantom{.0}} & {3\phantom{.0}} & 6 \\ \end{bmatrix} \\ \text{bsrColInd} & = & \begin{bmatrix} {1\phantom{.0}} & {2\phantom{.0}} & {1\phantom{.0}} & {2\phantom{.0}} & 3 \\ \end{bmatrix} \\ \end{matrix}\) |
Suppose we want to do the following bsrmv
operation on a matrix \(\overset{¯}{A}\) which is slightly different from \(A\) .
\(\begin{bmatrix} y_{1} \\ y_{2} \\ \end{bmatrix}:=alpha \ast (\widetilde{A} = \begin{bmatrix} O & O & O \\ O & A_{22} & O \\ \end{bmatrix}) \ast \begin{bmatrix} x_{1} \\ x_{2} \\ x_{3} \\ \end{bmatrix} + \begin{bmatrix} y_{1} \\ {beta \ast y_{2}} \\ \end{bmatrix}\) |
We don’t need to create another BSR format for the new matrix \(\overset{¯}{A}\) , all that we should do is to keep bsrVal
and bsrColInd
unchanged, but modify bsrRowPtr
and add an additional array bsrEndPtr
which points to the last nonzero elements per row of \(\overset{¯}{A}\) plus 1.
For example, the following bsrRowPtr
and bsrEndPtr
can represent matrix \(\overset{¯}{A}\) :
\(\begin{matrix} \text{bsrRowPtr} & = & \begin{bmatrix} {1\phantom{.0}} & 4 \\ \end{bmatrix} \\ \text{bsrEndPtr} & = & \begin{bmatrix} {1\phantom{.0}} & 5 \\ \end{bmatrix} \\ \end{matrix}\) |
Further we can use a mask operator (specified by array bsrMaskPtr
) to update particular block row indices of \(y\) only because \(y_{1}\) is never changed. In this case, bsrMaskPtr
\(=\) [2] and sizeOfMask
=1.
The mask operator is equivalent to the following operation:
\(\begin{bmatrix} ? \\ y_{2} \\ \end{bmatrix}:=alpha \ast \begin{bmatrix} ? & ? & ? \\ O & A_{22} & O \\ \end{bmatrix} \ast \begin{bmatrix} x_{1} \\ x_{2} \\ x_{3} \\ \end{bmatrix} + beta \ast \begin{bmatrix} ? \\ y_{2} \\ \end{bmatrix}\) |
If a block row is not present in the bsrMaskPtr
, then no calculation is performed on that row, and the corresponding value in y
is unmodified. The question mark “?” is used to inidcate row blocks not in bsrMaskPtr
.
In this case, first row block is not present in bsrMaskPtr
, so bsrRowPtr[0]
and bsrEndPtr[0]
are not touched also.
\(\begin{matrix} \text{bsrRowPtr} & = & \begin{bmatrix} {?\phantom{.0}} & 4 \\ \end{bmatrix} \\ \text{bsrEndPtr} & = & \begin{bmatrix} {?\phantom{.0}} & 5 \\ \end{bmatrix} \\ \end{matrix}\) |
bsrxmv()
has the following properties:
The routine requires no extra storage
The routine supports asynchronous execution
The routine supports CUDA graph capture
A couple of comments on bsrxmv()
:
Only
blockDim > 1
is supportedOnly
CUSPARSE_OPERATION_NON_TRANSPOSE
andCUSPARSE_MATRIX_TYPE_GENERAL
are supported.Parameters
bsrMaskPtr
,bsrRowPtr
,bsrEndPtr
andbsrColInd
are consistent with base index, either one-based or zero-based. The above example is one-based.
Input
|
handle to the cuSPARSE library context. |
|
storage format of blocks, either |
|
the operation \(\text{op}(A)\) . Only |
|
number of updated block rows of \(y\). |
|
number of block rows of matrix \(A\). |
|
number of block columns of matrix \(A\). |
|
number of nonzero blocks of matrix \(A\). |
|
<type> scalar used for multiplication. |
|
the descriptor of matrix \(A\). The supported matrix type is |
|
<type> array of |
|
integer array of |
|
integer array of |
|
integer array of |
|
integer array of |
|
block dimension of sparse matrix \(A\), larger than zero. |
|
<type> vector of \(nb \ast blockDim\) elements. |
|
<type> scalar used for multiplication. If |
|
<type> vector of \(mb \ast blockDim\) elements. |
See cusparseStatus_t for the description of the return status
8.3. cusparse<t>bsrsv2_bufferSize()
cusparseStatus_t
cusparseSbsrsv2_bufferSize(cusparseHandle_t handle,
cusparseDirection_t dirA,
cusparseOperation_t transA,
int mb,
int nnzb,
const cusparseMatDescr_t descrA,
float* bsrValA,
const int* bsrRowPtrA,
const int* bsrColIndA,
int blockDim,
bsrsv2Info_t info,
int* pBufferSizeInBytes)
cusparseStatus_t
cusparseDbsrsv2_bufferSize(cusparseHandle_t handle,
cusparseDirection_t dirA,
cusparseOperation_t transA,
int mb,
int nnzb,
const cusparseMatDescr_t descrA,
double* bsrValA,
const int* bsrRowPtrA,
const int* bsrColIndA,
int blockDim,
bsrsv2Info_t info,
int* pBufferSizeInBytes)
cusparseStatus_t
cusparseCbsrsv2_bufferSize(cusparseHandle_t handle,
cusparseDirection_t dirA,
cusparseOperation_t transA,
int mb,
int nnzb,
const cusparseMatDescr_t descrA,
cuComplex* bsrValA,
const int* bsrRowPtrA,
const int* bsrColIndA,
int blockDim,
bsrsv2Info_t info,
int* pBufferSizeInBytes)
cusparseStatus_t
cusparseZbsrsv2_bufferSize(cusparseHandle_t handle,
cusparseDirection_t dirA,
cusparseOperation_t transA,
int mb,
int nnzb,
const cusparseMatDescr_t descrA,
cuDoubleComplex* bsrValA,
const int* bsrRowPtrA,
const int* bsrColIndA,
int blockDim,
bsrsv2Info_t info,
int* pBufferSizeInBytes)
This function returns size of the buffer used in bsrsv2
, a new sparse triangular linear system op(A)*y =
\(\alpha\)x
.
A
is an (mb*blockDim)x(mb*blockDim)
sparse matrix that is defined in BSR storage format by the three arrays bsrValA
, bsrRowPtrA
, and bsrColIndA
); x
and y
are the right-hand-side and the solution vectors; \(\alpha\) is a scalar; and
\(\text{op}(A) = \begin{cases} A & \text{if trans == CUSPARSE_OPERATION_NON_TRANSPOSE} \\ A^{T} & \text{if trans == CUSPARSE_OPERATION_TRANSPOSE} \\ A^{H} & \text{if trans == CUSPARSE_OPERATION_CONJUGATE_TRANSPOSE} \\ \end{cases}\)
Although there are six combinations in terms of parameter trans
and the upper (lower) triangular part of A
, bsrsv2_bufferSize()
returns the maximum size buffer among these combinations. The buffer size depends on the dimensions mb
, blockDim
, and the number of nonzero blocks of the matrix nnzb
. If the user changes the matrix, it is necessary to call bsrsv2_bufferSize()
again to have the correct buffer size; otherwise a segmentation fault may occur.
The routine requires no extra storage
The routine supports asynchronous execution
The routine supports CUDA graph capture
Input
|
handle to the cuSPARSE library context. |
|
storage format of blocks, either |
|
the operation \(\text{op}(A)\) . |
|
number of block rows of matrix |
|
number of nonzero blocks of matrix |
|
the descriptor of matrix |
|
<type> array of |
|
integer array of |
|
integer array of |
|
block dimension of sparse matrix A; must be larger than zero. |
Output
|
record of internal states based on different algorithms. |
|
number of bytes of the buffer used in the |
See cusparseStatus_t for the description of the return status.
8.4. cusparse<t>bsrsv2_analysis()
cusparseStatus_t
cusparseSbsrsv2_analysis(cusparseHandle_t handle,
cusparseDirection_t dirA,
cusparseOperation_t transA,
int mb,
int nnzb,
const cusparseMatDescr_t descrA,
const float* bsrValA,
const int* bsrRowPtrA,
const int* bsrColIndA,
int blockDim,
bsrsv2Info_t info,
cusparseSolvePolicy_t policy,
void* pBuffer)
cusparseStatus_t
cusparseDbsrsv2_analysis(cusparseHandle_t handle,
cusparseDirection_t dirA,
cusparseOperation_t transA,
int mb,
int nnzb,
const cusparseMatDescr_t descrA,
const double* bsrValA,
const int* bsrRowPtrA,
const int* bsrColIndA,
int blockDim,
bsrsv2Info_t info,
cusparseSolvePolicy_t policy,
void* pBuffer)
cusparseStatus_t
cusparseDbsrsv2_analysis(cusparseHandle_t handle,
cusparseDirection_t dirA,
cusparseOperation_t transA,
int mb,
int nnzb,
const cusparseMatDescr_t descrA,
const cuComplex* bsrValA,
const int* bsrRowPtrA,
const int* bsrColIndA,
int blockDim,
bsrsv2Info_t info,
cusparseSolvePolicy_t policy,
void* pBuffer)
cusparseStatus_t
cusparseZbsrsv2_analysis(cusparseHandle_t handle,
cusparseDirection_t dirA,
cusparseOperation_t transA,
int mb,
int nnzb,
const cusparseMatDescr_t descrA,
const cuDoubleComplex* bsrValA,
const int* bsrRowPtrA,
const int* bsrColIndA,
int blockDim,
bsrsv2Info_t info,
cusparseSolvePolicy_t policy,
void* pBuffer)
This function performs the analysis phase of bsrsv2
, a new sparse triangular linear system op(A)*y =
\(\alpha\)x
.
A
is an (mb*blockDim)x(mb*blockDim)
sparse matrix that is defined in BSR storage format by the three arrays bsrValA
, bsrRowPtrA
, and bsrColIndA
); x
and y
are the right-hand side and the solution vectors; \(\alpha\) is a scalar; and
\(\text{op}(A) = \begin{cases} A & \text{if trans == CUSPARSE_OPERATION_NON_TRANSPOSE} \\ A^{T} & \text{if trans == CUSPARSE_OPERATION_TRANSPOSE} \\ A^{H} & \text{if trans == CUSPARSE_OPERATION_CONJUGATE_TRANSPOSE} \\ \end{cases}\)
The block of BSR format is of size blockDim*blockDim
, stored as column-major or row-major as determined by parameter dirA
, which is either CUSPARSE_DIRECTION_COLUMN
or CUSPARSE_DIRECTION_ROW
. The matrix type must be CUSPARSE_MATRIX_TYPE_GENERAL
, and the fill mode and diagonal type are ignored.
It is expected that this function will be executed only once for a given matrix and a particular operation type.
This function requires a buffer size returned by bsrsv2_bufferSize()
. The address of pBuffer
must be multiple of 128 bytes. If it is not, CUSPARSE_STATUS_INVALID_VALUE
is returned.
Function bsrsv2_analysis()
reports a structural zero and computes level information, which stored in the opaque structure info
. The level information can extract more parallelism for a triangular solver. However bsrsv2_solve()
can be done without level information. To disable level information, the user needs to specify the policy of the triangular solver as CUSPARSE_SOLVE_POLICY_NO_LEVEL
.
Function bsrsv2_analysis()
always reports the first structural zero, even when parameter policy
is CUSPARSE_SOLVE_POLICY_NO_LEVEL
. No structural zero is reported if CUSPARSE_DIAG_TYPE_UNIT
is specified, even if block A(j,j)
is missing for some j
. The user needs to call cusparseXbsrsv2_zeroPivot()
to know where the structural zero is.
It is the user’s choice whether to call bsrsv2_solve()
if bsrsv2_analysis()
reports a structural zero. In this case, the user can still call bsrsv2_solve()
, which will return a numerical zero at the same position as a structural zero. However the result x
is meaningless.
This function requires temporary extra storage that is allocated internally
The routine supports asynchronous execution if the Stream Ordered Memory Allocator is available
The routine supports CUDA graph capture if the Stream Ordered Memory Allocator is available
Input
|
handle to the cuSPARSE library context. |
|
storage format of blocks, either |
|
the operation \(\text{op}(A)\) . |
|
number of block rows of matrix |
|
number of nonzero blocks of matrix |
|
the descriptor of matrix |
|
<type> array of |
|
integer array of |
|
integer array of |
|
block dimension of sparse matrix A, larger than zero. |
|
structure initialized using |
|
the supported policies are |
|
buffer allocated by the user, the size is return by |
Output
|
structure filled with information collected during the analysis phase (that should be passed to the solve phase unchanged). |
See cusparseStatus_t for the description of the return status.
8.5. cusparse<t>bsrsv2_solve()
cusparseStatus_t
cusparseSbsrsv2_solve(cusparseHandle_t handle,
cusparseDirection_t dirA,
cusparseOperation_t transA,
int mb,
int nnzb,
const float* alpha,
const cusparseMatDescr_t descrA,
const float* bsrValA,
const int* bsrRowPtrA,
const int* bsrColIndA,
int blockDim,
bsrsv2Info_t info,
const float* x,
float* y,
cusparseSolvePolicy_t policy,
void* pBuffer)
cusparseStatus_t
cusparseDbsrsv2_solve(cusparseHandle_t handle,
cusparseDirection_t dirA,
cusparseOperation_t transA,
int mb,
int nnzb,
const double* alpha,
const cusparseMatDescr_t descrA,
const double* bsrValA,
const int* bsrRowPtrA,
const int* bsrColIndA,
int blockDim,
bsrsv2Info_t info,
const double* x,
double* y,
cusparseSolvePolicy_t policy,
void* pBuffer)
cusparseStatus_t
cusparseCbsrsv2_solve(cusparseHandle_t handle,
cusparseDirection_t dirA,
cusparseOperation_t transA,
int mb,
int nnzb,
const cuComplex* alpha,
const cusparseMatDescr_t descrA,
const cuComplex* bsrValA,
const int* bsrRowPtrA,
const int* bsrColIndA,
int blockDim,
bsrsv2Info_t info,
const cuComplex* x,
cuComplex* y,
cusparseSolvePolicy_t policy,
void* pBuffer)
cusparseStatus_t
cusparseZbsrsv2_solve(cusparseHandle_t handle,
cusparseDirection_t dirA,
cusparseOperation_t transA,
int mb,
int nnzb,
const cuDoubleComplex* alpha,
const cusparseMatDescr_t descrA,
const cuDoubleComplex* bsrValA,
const int* bsrRowPtrA,
const int* bsrColIndA,
int blockDim,
bsrsv2Info_t info,
const cuDoubleComplex* x,
cuDoubleComplex* y,
cusparseSolvePolicy_t policy,
void* pBuffer)
This function performs the solve phase of bsrsv2
, a new sparse triangular linear system op(A)*y =
\(\alpha\)x
.
A
is an (mb*blockDim)x(mb*blockDim)
sparse matrix that is defined in BSR storage format by the three arrays bsrValA
, bsrRowPtrA
, and bsrColIndA
); x
and y
are the right-hand-side and the solution vectors; \(\alpha\) is a scalar; and
\(\text{op}(A) = \begin{cases} A & \text{if trans == CUSPARSE_OPERATION_NON_TRANSPOSE} \\ A^{T} & \text{if trans == CUSPARSE_OPERATION_TRANSPOSE} \\ A^{H} & \text{if trans == CUSPARSE_OPERATION_CONJUGATE_TRANSPOSE} \\ \end{cases}\)
The block in BSR format is of size blockDim*blockDim
, stored as column-major or row-major as determined by parameter dirA
, which is either CUSPARSE_DIRECTION_COLUMN
or CUSPARSE_DIRECTION_ROW
. The matrix type must be CUSPARSE_MATRIX_TYPE_GENERAL
, and the fill mode and diagonal type are ignored. Function bsrsv02_solve()
can support an arbitrary blockDim
.
This function may be executed multiple times for a given matrix and a particular operation type.
This function requires a buffer size returned by bsrsv2_bufferSize()
. The address of pBuffer
must be multiple of 128 bytes. If it is not, CUSPARSE_STATUS_INVALID_VALUE
is returned.
Although bsrsv2_solve()
can be done without level information, the user still needs to be aware of consistency. If bsrsv2_analysis()
is called with policy CUSPARSE_SOLVE_POLICY_USE_LEVEL
, bsrsv2_solve()
can be run with or without levels. On the other hand, if bsrsv2_analysis()
is called with CUSPARSE_SOLVE_POLICY_NO_LEVEL
, bsrsv2_solve()
can only accept CUSPARSE_SOLVE_POLICY_NO_LEVEL
; otherwise, CUSPARSE_STATUS_INVALID_VALUE
is returned.
The level information may not improve the performance, but may spend extra time doing analysis. For example, a tridiagonal matrix has no parallelism. In this case, CUSPARSE_SOLVE_POLICY_NO_LEVEL
performs better than CUSPARSE_SOLVE_POLICY_USE_LEVEL
. If the user has an iterative solver, the best approach is to do bsrsv2_analysis()
with CUSPARSE_SOLVE_POLICY_USE_LEVEL
once. Then do bsrsv2_solve()
with CUSPARSE_SOLVE_POLICY_NO_LEVEL
in the first run, and with CUSPARSE_SOLVE_POLICY_USE_LEVEL
in the second run, and pick the fastest one to perform the remaining iterations.
Function bsrsv02_solve()
has the same behavior as csrsv02_solve()
. That is, bsr2csr(bsrsv02(A)) = csrsv02(bsr2csr(A))
. The numerical zero of csrsv02_solve()
means there exists some zero A(j,j)
. The numerical zero of bsrsv02_solve()
means there exists some block A(j,j)
that is not invertible.
Function bsrsv2_solve()
reports the first numerical zero, including a structural zero. No numerical zero is reported if CUSPARSE_DIAG_TYPE_UNIT
is specified, even if A(j,j)
is not invertible for some j
. The user needs to call cusparseXbsrsv2_zeroPivot()
to know where the numerical zero is.
The function supports the following properties if pBuffer != NULL
The routine requires no extra storage
The routine supports asynchronous execution
The routine supports CUDA graph capture
For example, suppose L is a lower triangular matrix with unit diagonal, then the following code solves L*y=x
by level information.
// Suppose that L is m x m sparse matrix represented by BSR format,
// The number of block rows/columns is mb, and
// the number of nonzero blocks is nnzb.
// L is lower triangular with unit diagonal.
// Assumption:
// - dimension of matrix L is m(=mb*blockDim),
// - matrix L has nnz(=nnzb*blockDim*blockDim) nonzero elements,
// - handle is already created by cusparseCreate(),
// - (d_bsrRowPtr, d_bsrColInd, d_bsrVal) is BSR of L on device memory,
// - d_x is right hand side vector on device memory.
// - d_y is solution vector on device memory.
// - d_x and d_y are of size m.
cusparseMatDescr_t descr = 0;
bsrsv2Info_t info = 0;
int pBufferSize;
void *pBuffer = 0;
int structural_zero;
int numerical_zero;
const double alpha = 1.;
const cusparseSolvePolicy_t policy = CUSPARSE_SOLVE_POLICY_USE_LEVEL;
const cusparseOperation_t trans = CUSPARSE_OPERATION_NON_TRANSPOSE;
const cusparseDirection_t dir = CUSPARSE_DIRECTION_COLUMN;
// step 1: create a descriptor which contains
// - matrix L is base-1
// - matrix L is lower triangular
// - matrix L has unit diagonal, specified by parameter CUSPARSE_DIAG_TYPE_UNIT
// (L may not have all diagonal elements.)
cusparseCreateMatDescr(&descr);
cusparseSetMatIndexBase(descr, CUSPARSE_INDEX_BASE_ONE);
cusparseSetMatFillMode(descr, CUSPARSE_FILL_MODE_LOWER);
cusparseSetMatDiagType(descr, CUSPARSE_DIAG_TYPE_UNIT);
// step 2: create a empty info structure
cusparseCreateBsrsv2Info(&info);
// step 3: query how much memory used in bsrsv2, and allocate the buffer
cusparseDbsrsv2_bufferSize(handle, dir, trans, mb, nnzb, descr,
d_bsrVal, d_bsrRowPtr, d_bsrColInd, blockDim, &pBufferSize);
// pBuffer returned by cudaMalloc is automatically aligned to 128 bytes.
cudaMalloc((void**)&pBuffer, pBufferSize);
// step 4: perform analysis
cusparseDbsrsv2_analysis(handle, dir, trans, mb, nnzb, descr,
d_bsrVal, d_bsrRowPtr, d_bsrColInd, blockDim,
info, policy, pBuffer);
// L has unit diagonal, so no structural zero is reported.
status = cusparseXbsrsv2_zeroPivot(handle, info, &structural_zero);
if (CUSPARSE_STATUS_ZERO_PIVOT == status){
printf("L(%d,%d) is missing\n", structural_zero, structural_zero);
}
// step 5: solve L*y = x
cusparseDbsrsv2_solve(handle, dir, trans, mb, nnzb, &alpha, descr,
d_bsrVal, d_bsrRowPtr, d_bsrColInd, blockDim, info,
d_x, d_y, policy, pBuffer);
// L has unit diagonal, so no numerical zero is reported.
status = cusparseXbsrsv2_zeroPivot(handle, info, &numerical_zero);
if (CUSPARSE_STATUS_ZERO_PIVOT == status){
printf("L(%d,%d) is zero\n", numerical_zero, numerical_zero);
}
// step 6: free resources
cudaFree(pBuffer);
cusparseDestroyBsrsv2Info(info);
cusparseDestroyMatDescr(descr);
cusparseDestroy(handle);
Input
|
handle to the cuSPARSE library context. |
|
storage format of blocks, either |
|
the operation \(\text{op}(A)\) . |
|
number of block rows and block columns of matrix |
|
<type> scalar used for multiplication. |
|
the descriptor of matrix |
|
<type> array of |
|
integer array of |
|
integer array of |
|
block dimension of sparse matrix |
|
structure with information collected during the analysis phase (that should have been passed to the solve phase unchanged). |
|
<type> right-hand-side vector of size |
|
the supported policies are |
|
buffer allocated by the user, the size is returned by |
Output
|
<type> solution vector of size |
See cusparseStatus_t for the description of the return status.
8.6. cusparseXbsrsv2_zeroPivot()
cusparseStatus_t
cusparseXbsrsv2_zeroPivot(cusparseHandle_t handle,
bsrsv2Info_t info,
int* position)
If the returned error code is CUSPARSE_STATUS_ZERO_PIVOT
, position=j
means A(j,j)
is either structural zero or numerical zero (singular block). Otherwise position=-1
.
The position
can be 0-based or 1-based, the same as the matrix.
Function cusparseXbsrsv2_zeroPivot()
is a blocking call. It calls cudaDeviceSynchronize()
to make sure all previous kernels are done.
The position
can be in the host memory or device memory. The user can set the proper mode with cusparseSetPointerMode()
.
The routine requires no extra storage
The routine supports asynchronous execution if the Stream Ordered Memory Allocator is available
The routine supports CUDA graph capture if the Stream Ordered Memory Allocator is available
Input
|
handle to the cuSPARSE library context. |
|
|
Output
|
if no structural or numerical zero, |
See cusparseStatus_t for the description of the return status
9. cuSPARSE Level 3 Function Reference
This chapter describes sparse linear algebra functions that perform operations between sparse and (usually tall) dense matrices.
In particular, the solution of sparse triangular linear systems with multiple right-hand sides is implemented in two phases. First, during the analysis phase, the sparse triangular matrix is analyzed to determine the dependencies between its elements by calling the appropriate csrsm2_analysis()
function. The analysis is specific to the sparsity pattern of the given matrix and to the selected cusparseOperation_t
type. The information from the analysis phase is stored in the parameter of type csrsm2Info_t
that has been initialized previously with a call to cusparseCreateCsrsm2Info()
.
Second, during the solve phase, the given sparse triangular linear system is solved using the information stored in the csrsm2Info_t
parameter by calling the appropriate csrsm2_solve()
function. The solve phase may be performed multiple times with different multiple right-hand sides, while the analysis phase needs to be performed only once. This is especially useful when a sparse triangular linear system must be solved for different sets of multiple right-hand sides one at a time, while its coefficient matrix remains the same.
Finally, once all the solves have completed, the opaque data structure pointed to by the csrsm2Info_t
parameter can be released by calling cusparseDestroyCsrsm2Info()
.
9.1. cusparse<t>bsrmm()
cusparseStatus_t
cusparseSbsrmm(cusparseHandle_t handle,
cusparseDirection_t dirA,
cusparseOperation_t transA,
cusparseOperation_t transB,
int mb,
int n,
int kb,
int nnzb,
const float* alpha,
const cusparseMatDescr_t descrA,
const float* bsrValA,
const int* bsrRowPtrA,
const int* bsrColIndA,
int blockDim,
const float* B,
int ldb,
const float* beta,
float* C,
int ldc)
cusparseStatus_t
cusparseDbsrmm(cusparseHandle_t handle,
cusparseDirection_t dirA,
cusparseOperation_t transA,
cusparseOperation_t transB,
int mb,
int n,
int kb,
int nnzb,
const double* alpha,
const cusparseMatDescr_t descrA,
const double* bsrValA,
const int* bsrRowPtrA,
const int* bsrColIndA,
int blockDim,
const double* B,
int ldb,
const double* beta,
double* C,
int ldc)
cusparseStatus_t
cusparseCbsrmm(cusparseHandle_t handle,
cusparseDirection_t dirA,
cusparseOperation_t transA,
cusparseOperation_t transB,
int mb,
int n,
int kb,
int nnzb,
const cuComplex* alpha,
const cusparseMatDescr_t descrA,
const cuComplex* bsrValA,
const int* bsrRowPtrA,
const int* bsrColIndA,
int blockDim,
const cuComplex* B,
int ldb,
const cuComplex* beta,
cuComplex* C,
int ldc)
cusparseStatus_t
cusparseZbsrmm(cusparseHandle_t handle,
cusparseDirection_t dirA,
cusparseOperation_t transA,
cusparseOperation_t transB,
int mb,
int n,
int kb,
int nnzb,
const cuDoubleComplex* alpha,
const cusparseMatDescr_t descrA,
const cuDoubleComplex* bsrValA,
const int* bsrRowPtrA,
const int* bsrColIndA,
int blockDim,
const cuDoubleComplex* B,
int ldb,
const cuDoubleComplex* beta,
cuDoubleComplex* C,
int ldc)
This function performs one of the following matrix-matrix operations:
\(C = \alpha \ast \text{op}(A) \ast \text{op}(B) + \beta \ast C\) |
A
is an mb×kb
sparse matrix that is defined in BSR storage format by the three arrays bsrValA
, bsrRowPtrA
, and bsrColIndA
; B
and C
are dense matrices; \(\alpha\text{~and~}\beta\) are scalars; and
\(\text{op}(A) = \begin{cases} A & \text{if transA == CUSPARSE_OPERATION_NON_TRANSPOSE} \\ A^{T} & \text{if transA == CUSPARSE_OPERATION_TRANSPOSE (not\ supported)} \\ A^{H} & \text{if transA == CUSPARSE_OPERATION_CONJUGATE_TRANSPOSE (not supported)} \\ \end{cases}\)
and
\(\text{op}(B) = \begin{cases} B & \text{if transB == CUSPARSE_OPERATION_NON_TRANSPOSE} \\ B^{T} & \text{if transB == CUSPARSE_OPERATION_TRANSPOSE} \\ B^{H} & \text{if transB == CUSPARSE_OPERATION_CONJUGATE_TRANSPOSE (not supported)} \\ \end{cases}\)
The function has the following limitations:
Only
CUSPARSE_MATRIX_TYPE_GENERAL
matrix type is supportedOnly
blockDim > 1
is supportedif
blockDim
≤ 4, then max(mb)/max(n) = 524,272if 4 <
blockDim
≤ 8, then max(mb) = 524,272, max(n) = 262,136if
blockDim
> 8, then m < 65,535 and max(n) = 262,136
The motivation of transpose(B)
is to improve memory access of matrix B
. The computational pattern of A*transpose(B)
with matrix B
in column-major order is equivalent to A*B
with matrix B
in row-major order.
In practice, no operation in an iterative solver or eigenvalue solver uses A*transpose(B)
. However, we can perform A*transpose(transpose(B))
which is the same as A*B
. For example, suppose A
is mb*kb
, B
is k*n
and C
is m*n
, the following code shows usage of cusparseDbsrmm()
.
// A is mb*kb, B is k*n and C is m*n
const int m = mb*blockSize;
const int k = kb*blockSize;
const int ldb_B = k; // leading dimension of B
const int ldc = m; // leading dimension of C
// perform C:=alpha*A*B + beta*C
cusparseSetMatType(descrA, CUSPARSE_MATRIX_TYPE_GENERAL );
cusparseDbsrmm(cusparse_handle,
CUSPARSE_DIRECTION_COLUMN,
CUSPARSE_OPERATION_NON_TRANSPOSE,
CUSPARSE_OPERATION_NON_TRANSPOSE,
mb, n, kb, nnzb, alpha,
descrA, bsrValA, bsrRowPtrA, bsrColIndA, blockSize,
B, ldb_B,
beta, C, ldc);
Instead of using A*B
, our proposal is to transpose B
to Bt
by first calling cublas<t>geam()
, and then to perform A*transpose(Bt)
.
// step 1: Bt := transpose(B)
const int m = mb*blockSize;
const int k = kb*blockSize;
double *Bt;
const int ldb_Bt = n; // leading dimension of Bt
cudaMalloc((void**)&Bt, sizeof(double)*ldb_Bt*k);
double one = 1.0;
double zero = 0.0;
cublasSetPointerMode(cublas_handle, CUBLAS_POINTER_MODE_HOST);
cublasDgeam(cublas_handle, CUBLAS_OP_T, CUBLAS_OP_T,
n, k, &one, B, int ldb_B, &zero, B, int ldb_B, Bt, ldb_Bt);
// step 2: perform C:=alpha*A*transpose(Bt) + beta*C
cusparseDbsrmm(cusparse_handle,
CUSPARSE_DIRECTION_COLUMN,
CUSPARSE_OPERATION_NON_TRANSPOSE,
CUSPARSE_OPERATION_TRANSPOSE,
mb, n, kb, nnzb, alpha,
descrA, bsrValA, bsrRowPtrA, bsrColIndA, blockSize,
Bt, ldb_Bt,
beta, C, ldc);
bsrmm()
has the following properties:
The routine requires no extra storage
The routine supports asynchronous execution
The routine supports CUDA graph capture
Input
|
handle to the cuSPARSE library context. |
|
storage format of blocks, either |
|
the operation |
|
the operation |
|
number of block rows of sparse matrix |
|
number of columns of dense matrix |
|
number of block columns of sparse matrix |
|
number of non-zero blocks of sparse matrix |
|
<type> scalar used for multiplication. |
|
the descriptor of matrix |
|
<type> array of |
|
integer array of |
|
integer array of |
|
block dimension of sparse matrix |
|
array of dimensions |
|
leading dimension of |
|
<type> scalar used for multiplication. If |
|
array of dimensions |
|
leading dimension of |
Output
|
<type> updated array of dimensions |
See cusparseStatus_t for the description of the return status
9.2. cusparse<t>bsrsm2_bufferSize()
cusparseStatus_t
cusparseSbsrsm2_bufferSize(cusparseHandle_t handle,
cusparseDirection_t dirA,
cusparseOperation_t transA,
cusparseOperation_t transX,
int mb,
int n,
int nnzb,
const cusparseMatDescr_t descrA,
float* bsrSortedValA,
const int* bsrSortedRowPtrA,
const int* bsrSortedColIndA,
int blockDim,
bsrsm2Info_t info,
int* pBufferSizeInBytes)
cusparseStatus_t
cusparseDbsrsm2_bufferSize(cusparseHandle_t handle,
cusparseDirection_t dirA,
cusparseOperation_t transA,
cusparseOperation_t transX,
int mb,
int n,
int nnzb,
const cusparseMatDescr_t descrA,
double* bsrSortedValA,
const int* bsrSortedRowPtrA,
const int* bsrSortedColIndA,
int blockDim,
bsrsm2Info_t info,
int* pBufferSizeInBytes)
cusparseStatus_t
cusparseCbsrsm2_bufferSize(cusparseHandle_t handle,
cusparseDirection_t dirA,
cusparseOperation_t transA,
cusparseOperation_t transX,
int mb,
int n,
int nnzb,
const cusparseMatDescr_t descrA,
cuComplex* bsrSortedValA,
const int* bsrSortedRowPtrA,
const int* bsrSortedColIndA,
int blockDim,
bsrsm2Info_t info,
int* pBufferSizeInBytes)
cusparseStatus_t
cusparseZbsrsm2_bufferSize(cusparseHandle_t handle,
cusparseDirection_t dirA,
cusparseOperation_t transA,
cusparseOperation_t transX,
int mb,
int n,
int nnzb,
const cusparseMatDescr_t descrA,
cuDoubleComplex* bsrSortedValA,
const int* bsrSortedRowPtrA,
const int* bsrSortedColIndA,
int blockDim,
bsrsm2Info_t info,
int* pBufferSizeInBytes)
This function returns size of buffer used in bsrsm2()
, a new sparse triangular linear system op(A)*op(X)=
\(\alpha\)op(B)
.
A
is an (mb*blockDim)x(mb*blockDim)
sparse matrix that is defined in BSR storage format by the three arrays bsrValA
, bsrRowPtrA
, and bsrColIndA
); B
and X
are the right-hand-side and the solution matrices; \(\alpha\) is a scalar; and
\(\text{op}(A) = \begin{cases} A & \text{if trans == CUSPARSE_OPERATION_NON_TRANSPOSE} \\ A^{T} & \text{if trans == CUSPARSE_OPERATION_TRANSPOSE} \\ A^{H} & \text{if trans == CUSPARSE_OPERATION_CONJUGATE_TRANSPOSE} \\ \end{cases}\)
Although there are six combinations in terms of parameter trans
and the upper (and lower) triangular part of A
, bsrsm2_bufferSize()
returns the maximum size of the buffer among these combinations. The buffer size depends on dimension mb,blockDim
and the number of nonzeros of the matrix, nnzb
. If the user changes the matrix, it is necessary to call bsrsm2_bufferSize()
again to get the correct buffer size, otherwise a segmentation fault may occur.
The routine requires no extra storage
The routine supports asynchronous execution
The routine supports CUDA graph capture
Input
|
handle to the cuSPARSE library context. |
|
storage format of blocks, either |
|
the operation |
|
the operation |
|
number of block rows of matrix |
|
number of columns of matrix |
|
number of nonzero blocks of matrix |
|
the descriptor of matrix |
|
<type> array of |
|
integer array of |
|
integer array of |
|
block dimension of sparse matrix |
Output
|
record internal states based on different algorithms. |
|
number of bytes of the buffer used in |
See cusparseStatus_t for the description of the return status
9.3. cusparse<t>bsrsm2_analysis()
cusparseStatus_t
cusparseSbsrsm2_analysis(cusparseHandle_t handle,
cusparseDirection_t dirA,
cusparseOperation_t transA,
cusparseOperation_t transX,
int mb,
int n,
int nnzb,
const cusparseMatDescr_t descrA,
const float* bsrSortedVal,
const int* bsrSortedRowPtr,
const int* bsrSortedColInd,
int blockDim,
bsrsm2Info_t info,
cusparseSolvePolicy_t policy,
void* pBuffer)
cusparseStatus_t
cusparseDbsrsm2_analysis(cusparseHandle_t handle,
cusparseDirection_t dirA,
cusparseOperation_t transA,
cusparseOperation_t transX,
int mb,
int n,
int nnzb,
const cusparseMatDescr_t descrA,
const double* bsrSortedVal,
const int* bsrSortedRowPtr,
const int* bsrSortedColInd,
int blockDim,
bsrsm2Info_t info,
cusparseSolvePolicy_t policy,
void* pBuffer)
cusparseStatus_t
cusparseCbsrsm2_analysis(cusparseHandle_t handle,
cusparseDirection_t dirA,
cusparseOperation_t transA,
cusparseOperation_t transX,
int mb,
int n,
int nnzb,
const cusparseMatDescr_t descrA,
const cuComplex* bsrSortedVal,
const int* bsrSortedRowPtr,
const int* bsrSortedColInd,
int blockDim,
bsrsm2Info_t info,
cusparseSolvePolicy_t policy,
void* pBuffer)
cusparseStatus_t
cusparseZbsrsm2_analysis(cusparseHandle_t handle,
cusparseDirection_t dirA,
cusparseOperation_t transA,
cusparseOperation_t transX,
int mb,
int n,
int nnzb,
const cusparseMatDescr_t descrA,
const cuDoubleComplex* bsrSortedVal,
const int* bsrSortedRowPtr,
const int* bsrSortedColInd,
int blockDim,
bsrsm2Info_t info,
cusparseSolvePolicy_t policy,
void* pBuffer)
This function performs the analysis phase of bsrsm2()
, a new sparse triangular linear system op(A)*op(X) =
\(\alpha\)op(B)
.
A
is an (mb*blockDim)x(mb*blockDim)
sparse matrix that is defined in BSR storage format by the three arrays bsrValA
, bsrRowPtrA
, and bsrColIndA
); B
and X
are the right-hand-side and the solution matrices; \(\alpha\) is a scalar; and
\(\text{op}(A) = \begin{cases} A & \text{if trans == CUSPARSE_OPERATION_NON_TRANSPOSE} \\ A^{T} & \text{if trans == CUSPARSE_OPERATION_TRANSPOSE} \\ A^{H} & \text{if trans == CUSPARSE_OPERATION_CONJUGATE_TRANSPOSE} \\ \end{cases}\)
and
\(\text{op}(X) = \begin{cases} X & \text{if transX == CUSPARSE_OPERATION_NON_TRANSPOSE} \\ X^{T} & \text{if transX == CUSPARSE_OPERATION_TRANSPOSE} \\ X^{H} & \text{if transX == CUSPARSE_OPERATION_CONJUGATE_TRANSPOSE (not supported)} \\ \end{cases}\)
and op(B)
and op(X)
are equal.
The block of BSR format is of size blockDim*blockDim
, stored in column-major or row-major as determined by parameter dirA
, which is either CUSPARSE_DIRECTION_ROW
or CUSPARSE_DIRECTION_COLUMN
. The matrix type must be CUSPARSE_MATRIX_TYPE_GENERAL
, and the fill mode and diagonal type are ignored.
It is expected that this function will be executed only once for a given matrix and a particular operation type.
This function requires the buffer size returned by bsrsm2_bufferSize()
. The address of pBuffer
must be multiple of 128 bytes. If not, CUSPARSE_STATUS_INVALID_VALUE
is returned.
Function bsrsm2_analysis()
reports a structural zero and computes the level information stored in opaque structure info
. The level information can extract more parallelism during a triangular solver. However bsrsm2_solve()
can be done without level information. To disable level information, the user needs to specify the policy of the triangular solver as CUSPARSE_SOLVE_POLICY_NO_LEVEL
.
Function bsrsm2_analysis()
always reports the first structural zero, even if the parameter policy
is CUSPARSE_SOLVE_POLICY_NO_LEVEL
. Besides, no structural zero is reported if CUSPARSE_DIAG_TYPE_UNIT
is specified, even if block A(j,j)
is missing for some j
. The user must call cusparseXbsrsm2_query_zero_pivot()
to know where the structural zero is.
If bsrsm2_analysis()
reports a structural zero, the solve will return a numerical zero in the same position as the structural zero but this result X
is meaningless.
This function requires temporary extra storage that is allocated internally
The routine supports asynchronous execution if the Stream Ordered Memory Allocator is available
The routine supports CUDA graph capture if the Stream Ordered Memory Allocator is available
Input
|
handle to the cuSPARSE library context. |
|
storage format of blocks, either |
|
the operation |
|
the operation |
|
number of block rows of matrix |
|
number of columns of matrix |
|
number of non-zero blocks of matrix |
|
the descriptor of matrix |
|
<type> array of |
|
integer array of |
|
integer array of |
|
block dimension of sparse matrix |
|
structure initialized using |
|
The supported policies are |
|
buffer allocated by the user; the size is return by |
Output
|
structure filled with information collected during the analysis phase (that should be passed to the solve phase unchanged). |
See cusparseStatus_t for the description of the return status
9.4. cusparse<t>bsrsm2_solve()
cusparseStatus_t
cusparseSbsrsm2_solve(cusparseHandle_t handle,
cusparseDirection_t dirA,
cusparseOperation_t transA,
cusparseOperation_t transX,
int mb,
int n,
int nnzb,
const float* alpha,
const cusparseMatDescr_t descrA,
const float* bsrSortedVal,
const int* bsrSortedRowPtr,
const int* bsrSortedColInd,
int blockDim,
bsrsm2Info_t info,
const float* B,
int ldb,
float* X,
int ldx,
cusparseSolvePolicy_t policy,
void* pBuffer)
cusparseStatus_t
cusparseDbsrsm2_solve(cusparseHandle_t handle,
cusparseDirection_t dirA,
cusparseOperation_t transA,
cusparseOperation_t transX,
int mb,
int n,
int nnzb,
const double* alpha,
const cusparseMatDescr_t descrA,
const double* bsrSortedVal,
const int* bsrSortedRowPtr,
const int* bsrSortedColInd,
int blockDim,
bsrsm2Info_t info,
const double* B,
int ldb,
double* X,
int ldx,
cusparseSolvePolicy_t policy,
void* pBuffer)
cusparseStatus_t
cusparseCbsrsm2_solve(cusparseHandle_t handle,
cusparseDirection_t dirA,
cusparseOperation_t transA,
cusparseOperation_t transX,
int mb,
int n,
int nnzb,
const cuComplex* alpha,
const cusparseMatDescr_t descrA,
const cuComplex* bsrSortedVal,
const int* bsrSortedRowPtr,
const int* bsrSortedColInd,
int blockDim,
bsrsm2Info_t info,
const cuComplex* B,
int ldb,
cuComplex* X,
int ldx,
cusparseSolvePolicy_t policy,
void* pBuffer)
cusparseStatus_t
cusparseZbsrsm2_solve(cusparseHandle_t handle,
cusparseDirection_t dirA,
cusparseOperation_t transA,
cusparseOperation_t transX,
int mb,
int n,
int nnzb,
const cuDoubleComplex* alpha,
const cusparseMatDescr_t descrA,
const cuDoubleComplex* bsrSortedVal,
const int* bsrSortedRowPtr,
const int* bsrSortedColInd,
int blockDim,
bsrsm2Info_t info,
const cuDoubleComplex* B,
int ldb,
cuDoubleComplex* X,
int ldx,
cusparseSolvePolicy_t policy,
void* pBuffer)
This function performs the solve phase of the solution of a sparse triangular linear system:
\(\text{op}(A) \ast \text{op(X)} = \alpha \ast \text{op(B)}\) |
A
is an (mb*blockDim)x(mb*blockDim)
sparse matrix that is defined in BSR storage format by the three arrays bsrValA
, bsrRowPtrA
, and bsrColIndA
); B
and X
are the right-hand-side and the solution matrices; \(\alpha\) is a scalar, and
\(\text{op}(A) = \begin{cases} A & \text{if transA == CUSPARSE_OPERATION_NON_TRANSPOSE} \\ A^{T} & \text{if transA == CUSPARSE_OPERATION_TRANSPOSE} \\ A^{H} & \text{if transA == CUSPARSE_OPERATION_CONJUGATE_TRANSPOSE} \\ \end{cases}\)
and
\(\text{op}(X) = \begin{cases} X & \text{if transX == CUSPARSE_OPERATION_NON_TRANSPOSE} \\ X^{T} & \text{if transX == CUSPARSE_OPERATION_TRANSPOSE} \\ X^{H} & \text{not supported} \\ \end{cases}\)
Only op(A)=A
is supported.
op(B)
and op(X)
must be performed in the same way. In other words, if op(B)=B
, op(X)=X
.
The block of BSR format is of size blockDim*blockDim
, stored as column-major or row-major as determined by parameter dirA
, which is either CUSPARSE_DIRECTION_ROW
or CUSPARSE_DIRECTION_COLUMN
. The matrix type must be CUSPARSE_MATRIX_TYPE_GENERAL
, and the fill mode and diagonal type are ignored. Function bsrsm02_solve()
can support an arbitrary blockDim
.
This function may be executed multiple times for a given matrix and a particular operation type.
This function requires the buffer size returned by bsrsm2_bufferSize()
. The address of pBuffer
must be multiple of 128 bytes. If it is not, CUSPARSE_STATUS_INVALID_VALUE
is returned.
Although bsrsm2_solve()
can be done without level information, the user still needs to be aware of consistency. If bsrsm2_analysis()
is called with policy CUSPARSE_SOLVE_POLICY_USE_LEVEL
, bsrsm2_solve()
can be run with or without levels. On the other hand, if bsrsm2_analysis()
is called with CUSPARSE_SOLVE_POLICY_NO_LEVEL
, bsrsm2_solve()
can only accept CUSPARSE_SOLVE_POLICY_NO_LEVEL
; otherwise, CUSPARSE_STATUS_INVALID_VALUE
is returned.
Function bsrsm02_solve()
has the same behavior as bsrsv02_solve()
, reporting the first numerical zero, including a structural zero. The user must call cusparseXbsrsm2_query_zero_pivot()
to know where the numerical zero is.
The motivation of transpose(X)
is to improve the memory access of matrix X
. The computational pattern of transpose(X)
with matrix X
in column-major order is equivalent to X
with matrix X
in row-major order.
In-place is supported and requires that B
and X
point to the same memory block, and ldb=ldx
.
The function supports the following properties if pBuffer != NULL
:
The routine requires no extra storage
The routine supports asynchronous execution
The routine supports CUDA graph capture
Input
|
handle to the cuSPARSE library context. |
|
storage format of blocks, either |
|
the operation |
|
the operation |
|
number of block rows of matrix |
|
number of columns of matrix |
|
number of non-zero blocks of matrix |
|
<type> scalar used for multiplication. |
|
the descriptor of matrix |
|
<type> array of |
|
integer array of |
|
integer array of |
|
block dimension of sparse matrix |
|
structure initialized using |
|
<type> right-hand-side array. |
|
leading dimension of |
|
leading dimension of |
|
the supported policies are |
|
buffer allocated by the user; the size is returned by |
Output
|
<type> solution array with leading dimensions |
See cusparseStatus_t for the description of the return status.
9.5. cusparseXbsrsm2_zeroPivot()
cusparseStatus_t
cusparseXbsrsm2_zeroPivot(cusparseHandle_t handle,
bsrsm2Info_t info,
int* position)
If the returned error code is CUSPARSE_STATUS_ZERO_PIVOT
, position=j
means A(j,j)
is either a structural zero or a numerical zero (singular block). Otherwise position=-1
.
The position
can be 0-base or 1-base, the same as the matrix.
Function cusparseXbsrsm2_zeroPivot()
is a blocking call. It calls cudaDeviceSynchronize()
to make sure all previous kernels are done.
The position
can be in the host memory or device memory. The user can set the proper mode with cusparseSetPointerMode()
.
The routine requires no extra storage
The routine supports asynchronous execution if the Stream Ordered Memory Allocator is available
The routine supports CUDA graph capture if the Stream Ordered Memory Allocator is available
Input
|
handle to the cuSPARSE library context. |
|
|
Output
|
if no structural or numerical zero, |
See cusparseStatus_t for the description of the return status.
10. cuSPARSE Extra Function Reference
This chapter describes the extra routines used to manipulate sparse matrices.
10.1. cusparse<t>csrgeam2()
cusparseStatus_t
cusparseScsrgeam2_bufferSizeExt(cusparseHandle_t handle,
int m,
int n,
const float* alpha,
const cusparseMatDescr_t descrA,
int nnzA,
const float* csrSortedValA,
const int* csrSortedRowPtrA,
const int* csrSortedColIndA,
const float* beta,
const cusparseMatDescr_t descrB,
int nnzB,
const float* csrSortedValB,
const int* csrSortedRowPtrB,
const int* csrSortedColIndB,
const cusparseMatDescr_t descrC,
const float* csrSortedValC,
const int* csrSortedRowPtrC,
const int* csrSortedColIndC,
size_t* pBufferSizeInBytes)
cusparseStatus_t
cusparseDcsrgeam2_bufferSizeExt(cusparseHandle_t handle,
int m,
int n,
const double* alpha,
const cusparseMatDescr_t descrA,
int nnzA,
const double* csrSortedValA,
const int* csrSortedRowPtrA,
const int* csrSortedColIndA,
const double* beta,
const cusparseMatDescr_t descrB,
int nnzB,
const double* csrSortedValB,
const int* csrSortedRowPtrB,
const int* csrSortedColIndB,
const cusparseMatDescr_t descrC,
const double* csrSortedValC,
const int* csrSortedRowPtrC,
const int* csrSortedColIndC,
size_t* pBufferSizeInBytes)
cusparseStatus_t
cusparseCcsrgeam2_bufferSizeExt(cusparseHandle_t handle,
int m,
int n,
const cuComplex* alpha,
const cusparseMatDescr_t descrA,
int nnzA,
const cuComplex* csrSortedValA,
const int* csrSortedRowPtrA,
const int* csrSortedColIndA,
const cuComplex* beta,
const cusparseMatDescr_t descrB,
int nnzB,
const cuComplex* csrSortedValB,
const int* csrSortedRowPtrB,
const int* csrSortedColIndB,
const cusparseMatDescr_t descrC,
const cuComplex* csrSortedValC,
const int* csrSortedRowPtrC,
const int* csrSortedColIndC,
size_t* pBufferSizeInBytes)
cusparseStatus_t
cusparseZcsrgeam2_bufferSizeExt(cusparseHandle_t handle,
int m,
int n,
const cuDoubleComplex* alpha,
const cusparseMatDescr_t descrA,
int nnzA,
const cuDoubleComplex* csrSortedValA,
const int* csrSortedRowPtrA,
const int* csrSortedColIndA,
const cuDoubleComplex* beta,
const cusparseMatDescr_t descrB,
int nnzB,
const cuDoubleComplex* csrSortedValB,
const int* csrSortedRowPtrB,
const int* csrSortedColIndB,
const cusparseMatDescr_t descrC,
const cuDoubleComplex* csrSortedValC,
const int* csrSortedRowPtrC,
const int* csrSortedColIndC,
size_t* pBufferSizeInBytes)
cusparseStatus_t
cusparseXcsrgeam2Nnz(cusparseHandle_t handle,
int m,
int n,
const cusparseMatDescr_t descrA,
int nnzA,
const int* csrSortedRowPtrA,
const int* csrSortedColIndA,
const cusparseMatDescr_t descrB,
int nnzB,
const int* csrSortedRowPtrB,
const int* csrSortedColIndB,
const cusparseMatDescr_t descrC,
int* csrSortedRowPtrC,
int* nnzTotalDevHostPtr,
void* workspace)
cusparseStatus_t
cusparseScsrgeam2(cusparseHandle_t handle,
int m,
int n,
const float* alpha,
const cusparseMatDescr_t descrA,
int nnzA,
const float* csrSortedValA,
const int* csrSortedRowPtrA,
const int* csrSortedColIndA,
const float* beta,
const cusparseMatDescr_t descrB,
int nnzB,
const float* csrSortedValB,
const int* csrSortedRowPtrB,
const int* csrSortedColIndB,
const cusparseMatDescr_t descrC,
float* csrSortedValC,
int* csrSortedRowPtrC,
int* csrSortedColIndC,
void* pBuffer)
cusparseStatus_t
cusparseDcsrgeam2(cusparseHandle_t handle,
int m,
int n,
const double* alpha,
const cusparseMatDescr_t descrA,
int nnzA,
const double* csrSortedValA,
const int* csrSortedRowPtrA,
const int* csrSortedColIndA,
const double* beta,
const cusparseMatDescr_t descrB,
int nnzB,
const double* csrSortedValB,
const int* csrSortedRowPtrB,
const int* csrSortedColIndB,
const cusparseMatDescr_t descrC,
double* csrSortedValC,
int* csrSortedRowPtrC,
int* csrSortedColIndC,
void* pBuffer)
cusparseStatus_t
cusparseCcsrgeam2(cusparseHandle_t handle,
int m,
int n,
const cuComplex* alpha,
const cusparseMatDescr_t descrA,
int nnzA,
const cuComplex* csrSortedValA,
const int* csrSortedRowPtrA,
const int* csrSortedColIndA,
const cuComplex* beta,
const cusparseMatDescr_t descrB,
int nnzB,
const cuComplex* csrSortedValB,
const int* csrSortedRowPtrB,
const int* csrSortedColIndB,
const cusparseMatDescr_t descrC,
cuComplex* csrSortedValC,
int* csrSortedRowPtrC,
int* csrSortedColIndC,
void* pBuffer)
cusparseStatus_t
cusparseZcsrgeam2(cusparseHandle_t handle,
int m,
int n,
const cuDoubleComplex* alpha,
const cusparseMatDescr_t descrA,
int nnzA,
const cuDoubleComplex* csrSortedValA,
const int* csrSortedRowPtrA,
const int* csrSortedColIndA,
const cuDoubleComplex* beta,
const cusparseMatDescr_t descrB,
int nnzB,
const cuDoubleComplex* csrSortedValB,
const int* csrSortedRowPtrB,
const int* csrSortedColIndB,
const cusparseMatDescr_t descrC,
cuDoubleComplex* csrSortedValC,
int* csrSortedRowPtrC,
int* csrSortedColIndC,
void* pBuffer)
This function performs following matrix-matrix operation
\(C = \alpha \ast A + \beta \ast B\) |
where A
, B
, and C
are m×n
sparse matrices (defined in CSR storage format by the three arrays csrValA|csrValB|csrValC
, csrRowPtrA|csrRowPtrB|csrRowPtrC
, and csrColIndA|csrColIndB|csrcolIndC
respectively), and \(\alpha\text{~and~}\beta\) are scalars. Since A
and B
have different sparsity patterns, cuSPARSE adopts a two-step approach to complete sparse matrix C
. In the first step, the user allocates csrRowPtrC
of m+1
elements and uses function cusparseXcsrgeam2Nnz()
to determine csrRowPtrC
and the total number of nonzero elements. In the second step, the user gathers nnzC
(number of nonzero elements of matrix C
) from either (nnzC=*nnzTotalDevHostPtr)
or (nnzC=csrRowPtrC(m)-csrRowPtrC(0))
and allocates csrValC, csrColIndC
of nnzC
elements respectively, then finally calls function cusparse[S|D|C|Z]csrgeam2()
to complete matrix C
.
The general procedure is as follows:
int baseC, nnzC;
/* alpha, nnzTotalDevHostPtr points to host memory */
size_t BufferSizeInBytes;
char *buffer = NULL;
int *nnzTotalDevHostPtr = &nnzC;
cusparseSetPointerMode(handle, CUSPARSE_POINTER_MODE_HOST);
cudaMalloc((void**)&csrRowPtrC, sizeof(int)*(m+1));
/* prepare buffer */
cusparseScsrgeam2_bufferSizeExt(handle, m, n,
alpha,
descrA, nnzA,
csrValA, csrRowPtrA, csrColIndA,
beta,
descrB, nnzB,
csrValB, csrRowPtrB, csrColIndB,
descrC,
csrValC, csrRowPtrC, csrColIndC
&bufferSizeInBytes
);
cudaMalloc((void**)&buffer, sizeof(char)*bufferSizeInBytes);
cusparseXcsrgeam2Nnz(handle, m, n,
descrA, nnzA, csrRowPtrA, csrColIndA,
descrB, nnzB, csrRowPtrB, csrColIndB,
descrC, csrRowPtrC, nnzTotalDevHostPtr,
buffer);
if (NULL != nnzTotalDevHostPtr){
nnzC = *nnzTotalDevHostPtr;
}else{
cudaMemcpy(&nnzC, csrRowPtrC+m, sizeof(int), cudaMemcpyDeviceToHost);
cudaMemcpy(&baseC, csrRowPtrC, sizeof(int), cudaMemcpyDeviceToHost);
nnzC -= baseC;
}
cudaMalloc((void**)&csrColIndC, sizeof(int)*nnzC);
cudaMalloc((void**)&csrValC, sizeof(float)*nnzC);
cusparseScsrgeam2(handle, m, n,
alpha,
descrA, nnzA,
csrValA, csrRowPtrA, csrColIndA,
beta,
descrB, nnzB,
csrValB, csrRowPtrB, csrColIndB,
descrC,
csrValC, csrRowPtrC, csrColIndC
buffer);
Several comments on csrgeam2()
:
The other three combinations, NT, TN, and TT, are not supported by cuSPARSE. In order to do any one of the three, the user should use the routine
csr2csc()
to convert \(A\) | \(B\) to \(A^{T}\) | \(B^{T}\) .Only
CUSPARSE_MATRIX_TYPE_GENERAL
is supported. If eitherA
orB
is symmetric or Hermitian, then the user must extend the matrix to a full one and reconfigure theMatrixType
field of the descriptor toCUSPARSE_MATRIX_TYPE_GENERAL
.If the sparsity pattern of matrix
C
is known, the user can skip the call to functioncusparseXcsrgeam2Nnz()
. For example, suppose that the user has an iterative algorithm which would updateA
andB
iteratively but keep the sparsity patterns. The user can call functioncusparseXcsrgeam2Nnz()
once to set up the sparsity pattern ofC
, then call functioncusparse[S|D|C|Z]geam()
only for each iteration.The pointers
alpha
andbeta
must be valid.When
alpha
orbeta
is zero, it is not considered a special case by cuSPARSE. The sparsity pattern ofC
is independent of the value ofalpha
andbeta
. If the user wants \(C = 0 \times A + 1 \times B^{T}\) , thencsr2csc()
is better thancsrgeam2()
.csrgeam2()
is the same ascsrgeam()
exceptcsrgeam2()
needs explicit buffer wherecsrgeam()
allocates the buffer internally.This function requires temporary extra storage that is allocated internally
The routine supports asynchronous execution if the Stream Ordered Memory Allocator is available
The routine supports CUDA graph capture if the Stream Ordered Memory Allocator is available
Input
|
handle to the cuSPARSE library context. |
|
number of rows of sparse matrix |
|
number of columns of sparse matrix |
|
<type> scalar used for multiplication. |
|
the descriptor of matrix |
|
number of nonzero elements of sparse matrix |
|
<type> array of |
|
integer array of |
|
integer array of |
|
<type> scalar used for multiplication. If |
|
the descriptor of matrix |
|
number of nonzero elements of sparse matrix |
|
<type> array of |
|
integer array of |
|
integer array of |
|
the descriptor of matrix |
Output
|
<type> array of |
|
integer array of |
|
integer array of |
|
total number of nonzero elements in device or host memory. It is equal to |
See cusparseStatus_t for the description of the return status
11. cuSPARSE Preconditioners Reference
This chapter describes the routines that implement different preconditioners.
11.1. Incomplete Cholesky Factorization: level 0
Different algorithms for ic0 are discussed in this section.
11.1.1. cusparse<t>csric02_bufferSize()
cusparseStatus_t
cusparseScsric02_bufferSize(cusparseHandle_t handle,
int m,
int nnz,
const cusparseMatDescr_t descrA,
float* csrValA,
const int* csrRowPtrA,
const int* csrColIndA,
csric02Info_t info,
int* pBufferSizeInBytes)
cusparseStatus_t
cusparseDcsric02_bufferSize(cusparseHandle_t handle,
int m,
int nnz,
const cusparseMatDescr_t descrA,
double* csrValA,
const int* csrRowPtrA,
const int* csrColIndA,
csric02Info_t info,
int* pBufferSizeInBytes)
cusparseStatus_t
cusparseCcsric02_bufferSize(cusparseHandle_t handle,
int m,
int nnz,
const cusparseMatDescr_t descrA,
cuComplex* csrValA,
const int* csrRowPtrA,
const int* csrColIndA,
csric02Info_t info,
int* pBufferSizeInBytes)
cusparseStatus_t
cusparseZcsric02_bufferSize(cusparseHandle_t handle,
int m,
int nnz,
const cusparseMatDescr_t descrA,
cuDoubleComplex* csrValA,
const int* csrRowPtrA,
const int* csrColIndA,
csric02Info_t info,
int* pBufferSizeInBytes)
This function returns size of buffer used in computing the incomplete-Cholesky factorization with \(0\) fill-in and no pivoting:
\(A \approx LL^{H}\) |
A
is an m×m
sparse matrix that is defined in CSR storage format by the three arrays csrValA
, csrRowPtrA
, and csrColIndA
.
The buffer size depends on dimension m
and nnz
, the number of nonzeros of the matrix. If the user changes the matrix, it is necessary to call csric02_bufferSize()
again to have the correct buffer size; otherwise, a segmentation fault may occur.
The routine requires no extra storage
The routine supports asynchronous execution
The routine supports CUDA graph capture
Input
|
handle to the cuSPARSE library context. |
|
number of rows and columns of matrix |
|
number of nonzeros of matrix |
|
the descriptor of matrix |
|
<type> array of |
|
integer array of |
|
integer array of |
Output
|
record internal states based on different algorithms |
|
number of bytes of the buffer used in |
See cusparseStatus_t for the description of the return status.
11.1.2. cusparse<t>csric02_analysis()
cusparseStatus_t
cusparseScsric02_analysis(cusparseHandle_t handle,
int m,
int nnz,
const cusparseMatDescr_t descrA,
const float* csrValA,
const int* csrRowPtrA,
const int* csrColIndA,
csric02Info_t info,
cusparseSolvePolicy_t policy,
void* pBuffer)
cusparseStatus_t
cusparseDcsric02_analysis(cusparseHandle_t handle,
int m,
int nnz,
const cusparseMatDescr_t descrA,
const double* csrValA,
const int* csrRowPtrA,
const int* csrColIndA,
csric02Info_t info,
cusparseSolvePolicy_t policy,
void* pBuffer)
cusparseStatus_t
cusparseCcsric02_analysis(cusparseHandle_t handle,
int m,
int nnz,
const cusparseMatDescr_t descrA,
const cuComplex* csrValA,
const int* csrRowPtrA,
const int* csrColIndA,
csric02Info_t info,
cusparseSolvePolicy_t policy,
void* pBuffer)
cusparseStatus_t
cusparseZcsric02_analysis(cusparseHandle_t handle,
int m,
int nnz,
const cusparseMatDescr_t descrA,
const cuDoubleComplex* csrValA,
const int* csrRowPtrA,
const int* csrColIndA,
csric02Info_t info,
cusparseSolvePolicy_t policy,
void* pBuffer)
This function performs the analysis phase of the incomplete-Cholesky factorization with \(0\) fill-in and no pivoting:
\(A \approx LL^{H}\) |
A
is an m×m
sparse matrix that is defined in CSR storage format by the three arrays csrValA
, csrRowPtrA
, and csrColIndA
.
This function requires a buffer size returned by csric02_bufferSize()
. The address of pBuffer
must be multiple of 128 bytes. If not, CUSPARSE_STATUS_INVALID_VALUE
is returned.
Function csric02_analysis()
reports a structural zero and computes level information stored in the opaque structure info
. The level information can extract more parallelism during incomplete Cholesky factorization. However csric02()
can be done without level information. To disable level information, the user must specify the policy of csric02_analysis()
and csric02()
as CUSPARSE_SOLVE_POLICY_NO_LEVEL
.
Function csric02_analysis()
always reports the first structural zero, even if the policy is CUSPARSE_SOLVE_POLICY_NO_LEVEL
. The user needs to call cusparseXcsric02_zeroPivot()
to know where the structural zero is.
It is the user’s choice whether to call csric02()
if csric02_analysis()
reports a structural zero. In this case, the user can still call csric02()
, which will return a numerical zero at the same position as the structural zero. However the result is meaningless.
This function requires temporary extra storage that is allocated internally
The routine supports asynchronous execution if the Stream Ordered Memory Allocator is available
The routine supports CUDA graph capture if the Stream Ordered Memory Allocator is available
Input
|
handle to the cuSPARSE library context. |
|
number of rows and columns of matrix |
|
number of nonzeros of matrix |
|
the descriptor of matrix |
|
<type> array of |
|
integer array of |
|
integer array of |
|
structure initialized using |
|
the supported policies are |
|
buffer allocated by the user; the size is returned by |
Output
|
number of bytes of the buffer used in |
See cusparseStatus_t for the description of the return status.
11.1.3. cusparse<t>csric02()
cusparseStatus_t
cusparseScsric02(cusparseHandle_t handle,
int m,
int nnz,
const cusparseMatDescr_t descrA,
float* csrValA_valM,
const int* csrRowPtrA,
const int* csrColIndA,
csric02Info_t info,
cusparseSolvePolicy_t policy,
void* pBuffer)
cusparseStatus_t
cusparseDcsric02(cusparseHandle_t handle,
int m,
int nnz,
const cusparseMatDescr_t descrA,
double* csrValA_valM,
const int* csrRowPtrA,
const int* csrColIndA,
csric02Info_t info,
cusparseSolvePolicy_t policy,
void* pBuffer)
cusparseStatus_t
cusparseCcsric02(cusparseHandle_t handle,
int m,
int nnz,
const cusparseMatDescr_t descrA,
cuComplex* csrValA_valM,
const int* csrRowPtrA,
const int* csrColIndA,
csric02Info_t info,
cusparseSolvePolicy_t policy,
void* pBuffer)
cusparseStatus_t
cusparseZcsric02(cusparseHandle_t handle,
int m,
int nnz,
const cusparseMatDescr_t descrA,
cuDoubleComplex* csrValA_valM,
const int* csrRowPtrA,
const int* csrColIndA,
csric02Info_t info,
cusparseSolvePolicy_t policy,
void* pBuffer)
This function performs the solve phase of the computing the incomplete-Cholesky factorization with \(0\) fill-in and no pivoting:
\(A \approx LL^{H}\) |
This function requires a buffer size returned by csric02_bufferSize()
. The address of pBuffer
must be a multiple of 128 bytes. If not, CUSPARSE_STATUS_INVALID_VALUE
is returned.
Although csric02()
can be done without level information, the user still needs to be aware of consistency. If csric02_analysis()
is called with policy CUSPARSE_SOLVE_POLICY_USE_LEVEL
, csric02()
can be run with or without levels. On the other hand, if csric02_analysis()
is called with CUSPARSE_SOLVE_POLICY_NO_LEVEL
, csric02()
can only accept CUSPARSE_SOLVE_POLICY_NO_LEVEL
; otherwise, CUSPARSE_STATUS_INVALID_VALUE
is returned.
Function csric02()
reports the first numerical zero, including a structural zero. The user must call cusparseXcsric02_zeroPivot()
to know where the numerical zero is.
Function csric02()
only takes the lower triangular part of matrix A
to perform factorization. The matrix type must be CUSPARSE_MATRIX_TYPE_GENERAL
, the fill mode and diagonal type are ignored, and the strictly upper triangular part is ignored and never touched. It does not matter if A
is Hermitian or not. In other words, from the point of view of csric02()
A
is Hermitian and only the lower triangular part is provided.
Note
In practice, a positive definite matrix may not have incomplete cholesky factorization. To the best of our knowledge, only matrix M
can guarantee the existence of incomplete cholesky factorization. If csric02()
failed cholesky factorization and reported a numerical zero, it is possible that incomplete cholesky factorization does not exist.
For example, suppose A
is a real m × m
matrix, the following code solves the precondition system M*y = x
where M
is the product of Cholesky factorization L
and its transpose.
\(M = LL^{H}\) |
// Suppose that A is m x m sparse matrix represented by CSR format,
// Assumption:
// - handle is already created by cusparseCreate(),
// - (d_csrRowPtr, d_csrColInd, d_csrVal) is CSR of A on device memory,
// - d_x is right hand side vector on device memory,
// - d_y is solution vector on device memory.
// - d_z is intermediate result on device memory.
cusparseMatDescr_t descr_M = 0;
cusparseMatDescr_t descr_L = 0;
csric02Info_t info_M = 0;
csrsv2Info_t info_L = 0;
csrsv2Info_t info_Lt = 0;
int pBufferSize_M;
int pBufferSize_L;
int pBufferSize_Lt;
int pBufferSize;
void *pBuffer = 0;
int structural_zero;
int numerical_zero;
const double alpha = 1.;
const cusparseSolvePolicy_t policy_M = CUSPARSE_SOLVE_POLICY_NO_LEVEL;
const cusparseSolvePolicy_t policy_L = CUSPARSE_SOLVE_POLICY_NO_LEVEL;
const cusparseSolvePolicy_t policy_Lt = CUSPARSE_SOLVE_POLICY_USE_LEVEL;
const cusparseOperation_t trans_L = CUSPARSE_OPERATION_NON_TRANSPOSE;
const cusparseOperation_t trans_Lt = CUSPARSE_OPERATION_TRANSPOSE;
// step 1: create a descriptor which contains
// - matrix M is base-1
// - matrix L is base-1
// - matrix L is lower triangular
// - matrix L has non-unit diagonal
cusparseCreateMatDescr(&descr_M);
cusparseSetMatIndexBase(descr_M, CUSPARSE_INDEX_BASE_ONE);
cusparseSetMatType(descr_M, CUSPARSE_MATRIX_TYPE_GENERAL);
cusparseCreateMatDescr(&descr_L);
cusparseSetMatIndexBase(descr_L, CUSPARSE_INDEX_BASE_ONE);
cusparseSetMatType(descr_L, CUSPARSE_MATRIX_TYPE_GENERAL);
cusparseSetMatFillMode(descr_L, CUSPARSE_FILL_MODE_LOWER);
cusparseSetMatDiagType(descr_L, CUSPARSE_DIAG_TYPE_NON_UNIT);
// step 2: create a empty info structure
// we need one info for csric02 and two info's for csrsv2
cusparseCreateCsric02Info(&info_M);
cusparseCreateCsrsv2Info(&info_L);
cusparseCreateCsrsv2Info(&info_Lt);
// step 3: query how much memory used in csric02 and csrsv2, and allocate the buffer
cusparseDcsric02_bufferSize(handle, m, nnz,
descr_M, d_csrVal, d_csrRowPtr, d_csrColInd, info_M, &bufferSize_M);
cusparseDcsrsv2_bufferSize(handle, trans_L, m, nnz,
descr_L, d_csrVal, d_csrRowPtr, d_csrColInd, info_L, &pBufferSize_L);
cusparseDcsrsv2_bufferSize(handle, trans_Lt, m, nnz,
descr_L, d_csrVal, d_csrRowPtr, d_csrColInd, info_Lt,&pBufferSize_Lt);
pBufferSize = max(bufferSize_M, max(pBufferSize_L, pBufferSize_Lt));
// pBuffer returned by cudaMalloc is automatically aligned to 128 bytes.
cudaMalloc((void**)&pBuffer, pBufferSize);
// step 4: perform analysis of incomplete Cholesky on M
// perform analysis of triangular solve on L
// perform analysis of triangular solve on L'
// The lower triangular part of M has the same sparsity pattern as L, so
// we can do analysis of csric02 and csrsv2 simultaneously.
cusparseDcsric02_analysis(handle, m, nnz, descr_M,
d_csrVal, d_csrRowPtr, d_csrColInd, info_M,
policy_M, pBuffer);
status = cusparseXcsric02_zeroPivot(handle, info_M, &structural_zero);
if (CUSPARSE_STATUS_ZERO_PIVOT == status){
printf("A(%d,%d) is missing\n", structural_zero, structural_zero);
}
cusparseDcsrsv2_analysis(handle, trans_L, m, nnz, descr_L,
d_csrVal, d_csrRowPtr, d_csrColInd,
info_L, policy_L, pBuffer);
cusparseDcsrsv2_analysis(handle, trans_Lt, m, nnz, descr_L,
d_csrVal, d_csrRowPtr, d_csrColInd,
info_Lt, policy_Lt, pBuffer);
// step 5: M = L * L'
cusparseDcsric02(handle, m, nnz, descr_M,
d_csrVal, d_csrRowPtr, d_csrColInd, info_M, policy_M, pBuffer);
status = cusparseXcsric02_zeroPivot(handle, info_M, &numerical_zero);
if (CUSPARSE_STATUS_ZERO_PIVOT == status){
printf("L(%d,%d) is zero\n", numerical_zero, numerical_zero);
}
// step 6: solve L*z = x
cusparseDcsrsv2_solve(handle, trans_L, m, nnz, &alpha, descr_L,
d_csrVal, d_csrRowPtr, d_csrColInd, info_L,
d_x, d_z, policy_L, pBuffer);
// step 7: solve L'*y = z
cusparseDcsrsv2_solve(handle, trans_Lt, m, nnz, &alpha, descr_L,
d_csrVal, d_csrRowPtr, d_csrColInd, info_Lt,
d_z, d_y, policy_Lt, pBuffer);
// step 6: free resources
cudaFree(pBuffer);
cusparseDestroyMatDescr(descr_M);
cusparseDestroyMatDescr(descr_L);
cusparseDestroyCsric02Info(info_M);
cusparseDestroyCsrsv2Info(info_L);
cusparseDestroyCsrsv2Info(info_Lt);
cusparseDestroy(handle);
The function supports the following properties if pBuffer != NULL
This function requires temporary extra storage that is allocated internally
The routine supports asynchronous execution if the Stream Ordered Memory Allocator is available
The routine supports CUDA graph capture if the Stream Ordered Memory Allocator is available
Input
|
handle to the cuSPARSE library context. |
|
number of rows and columns of matrix |
|
number of nonzeros of matrix |
|
the descriptor of matrix |
|
<type> array of |
|
integer array of |
|
integer array of |
|
structure with information collected during the analysis phase (that should have been passed to the solve phase unchanged). |
|
the supported policies are |
|
buffer allocated by the user; the size is returned by |
Output
|
<type> matrix containing the incomplete-Cholesky lower triangular factor. |
See cusparseStatus_t for the description of the return status.
11.1.4. cusparseXcsric02_zeroPivot()
cusparseStatus_t
cusparseXcsric02_zeroPivot(cusparseHandle_t handle,
csric02Info_t info,
int* position)
If the returned error code is CUSPARSE_STATUS_ZERO_PIVOT
, position=j
means A(j,j)
has either a structural zero or a numerical zero; otherwise, position=-1
.
The position
can be 0-based or 1-based, the same as the matrix.
Function cusparseXcsric02_zeroPivot()
is a blocking call. It calls cudaDeviceSynchronize()
to make sure all previous kernels are done.
The position
can be in the host memory or device memory. The user can set proper mode with cusparseSetPointerMode()
.
The routine requires no extra storage
The routine supports asynchronous execution if the Stream Ordered Memory Allocator is available
The routine supports CUDA graph capture if the Stream Ordered Memory Allocator is available
Input
|
handle to the cuSPARSE library context. |
|
|
Output
|
if no structural or numerical zero, |
See cusparseStatus_t for the description of the return status.
11.1.5. cusparse<t>bsric02_bufferSize()
cusparseStatus_t
cusparseSbsric02_bufferSize(cusparseHandle_t handle,
cusparseDirection_t dirA,
int mb,
int nnzb,
const cusparseMatDescr_t descrA,
float* bsrValA,
const int* bsrRowPtrA,
const int* bsrColIndA,
int blockDim,
bsric02Info_t info,
int* pBufferSizeInBytes)
cusparseStatus_t
cusparseDbsric02_bufferSize(cusparseHandle_t handle,
cusparseDirection_t dirA,
int mb,
int nnzb,
const cusparseMatDescr_t descrA,
double* bsrValA,
const int* bsrRowPtrA,
const int* bsrColIndA,
int blockDim,
bsric02Info_t info,
int* pBufferSizeInBytes)
cusparseStatus_t
cusparseCbsric02_bufferSize(cusparseHandle_t handle,
cusparseDirection_t dirA,
int mb,
int nnzb,
const cusparseMatDescr_t descrA,
cuComplex* bsrValA,
const int* bsrRowPtrA,
const int* bsrColIndA,
int blockDim,
bsric02Info_t info,
int* pBufferSizeInBytes)
cusparseStatus_t
cusparseZbsric02_bufferSize(cusparseHandle_t handle,
cusparseDirection_t dirA,
int mb,
int nnzb,
const cusparseMatDescr_t descrA,
cuDoubleComplex* bsrValA,
const int* bsrRowPtrA,
const int* bsrColIndA,
int blockDim,
bsric02Info_t info,
int* pBufferSizeInBytes)
This function returns the size of a buffer used in computing the incomplete-Cholesky factorization with 0 fill-in and no pivoting
\(A \approx LL^{H}\) |
A
is an (mb*blockDim)*(mb*blockDim)
sparse matrix that is defined in BSR storage format by the three arrays bsrValA
, bsrRowPtrA
, and bsrColIndA
.
The buffer size depends on the dimensions of mb
, blockDim
, and the number of nonzero blocks of the matrix nnzb
. If the user changes the matrix, it is necessary to call bsric02_bufferSize()
again to have the correct buffer size; otherwise, a segmentation fault may occur.
The routine requires no extra storage
The routine supports asynchronous execution
The routine supports CUDA graph capture
Input
|
handle to the cuSPARSE library context. |
|
storage format of blocks, either |
|
number of block rows and block columns of matrix |
|
number of nonzero blocks of matrix |
|
the descriptor of matrix |
|
<type> array of |
|
integer array of |
|
integer array of |
|
block dimension of sparse matrix A, larger than zero. |
Output
|
record internal states based on different algorithms. |
|
number of bytes of the buffer used in |
See cusparseStatus_t for the description of the return status.
11.1.6. cusparse<t>bsric02_analysis()
cusparseStatus_t
cusparseSbsric02_analysis(cusparseHandle_t handle,
cusparseDirection_t dirA,
int mb,
int nnzb,
const cusparseMatDescr_t descrA,
const float* bsrValA,
const int* bsrRowPtrA,
const int* bsrColIndA,
int blockDim,
bsric02Info_t info,
cusparseSolvePolicy_t policy,
void* pBuffer)
cusparseStatus_t
cusparseDbsric02_analysis(cusparseHandle_t handle,
cusparseDirection_t dirA,
int mb,
int nnzb,
const cusparseMatDescr_t descrA,
const double* bsrValA,
const int* bsrRowPtrA,
const int* bsrColIndA,
int blockDim,
bsric02Info_t info,
cusparseSolvePolicy_t policy,
void* pBuffer)
cusparseStatus_t
cusparseCbsric02_analysis(cusparseHandle_t handle,
cusparseDirection_t dirA,
int mb,
int nnzb,
const cusparseMatDescr_t descrA,
const cuComplex* bsrValA,
const int* bsrRowPtrA,
const int* bsrColIndA,
int blockDim,
bsric02Info_t info,
cusparseSolvePolicy_t policy,
void* pBuffer)
cusparseStatus_t
cusparseZbsric02_analysis(cusparseHandle_t handle,
cusparseDirection_t dirA,
int mb,
int nnzb,
const cusparseMatDescr_t descrA,
const cuDoubleComplex* bsrValA,
const int* bsrRowPtrA,
const int* bsrColIndA,
int blockDim,
bsric02Info_t info,
cusparseSolvePolicy_t policy,
void* pBuffer)
This function performs the analysis phase of the incomplete-Cholesky factorization with 0 fill-in and no pivoting
\(A \approx LL^{H}\) |
A
is an (mb*blockDim)x(mb*blockDim)
sparse matrix that is defined in BSR storage format by the three arrays bsrValA
, bsrRowPtrA
, and bsrColIndA
. The block in BSR format is of size blockDim*blockDim
, stored as column-major or row-major as determined by parameter dirA
, which is either CUSPARSE_DIRECTION_COLUMN
or CUSPARSE_DIRECTION_ROW
. The matrix type must be CUSPARSE_MATRIX_TYPE_GENERAL
, and the fill mode and diagonal type are ignored.
This function requires a buffer size returned by bsric02_bufferSize90
. The address of pBuffer
must be a multiple of 128 bytes. If it is not, CUSPARSE_STATUS_INVALID_VALUE
is returned.
Functionbsric02_analysis()
reports structural zero and computes level information stored in the opaque structure info
. The level information can extract more parallelism during incomplete Cholesky factorization. However bsric02()
can be done without level information. To disable level information, the user needs to specify the parameter policy
of bsric02[_analysis| ]
as CUSPARSE_SOLVE_POLICY_NO_LEVEL
.
Function bsric02_analysis
always reports the first structural zero, even when parameter policy
is CUSPARSE_SOLVE_POLICY_NO_LEVEL
. The user must call cusparseXbsric02_zeroPivot()
to know where the structural zero is.
It is the user’s choice whether to call bsric02()
if bsric02_analysis()
reports a structural zero. In this case, the user can still call bsric02()
, which returns a numerical zero in the same position as the structural zero. However the result is meaningless.
This function requires temporary extra storage that is allocated internally
The routine supports asynchronous execution if the Stream Ordered Memory Allocator is available
The routine supports CUDA graph capture if the Stream Ordered Memory Allocator is available
Input
|
handle to the cuSPARSE library context. |
|
storage format of blocks, either |
|
number of block rows and block columns of matrix |
|
number of nonzero blocks of matrix |
|
the descriptor of matrix |
|
<type> array of |
|
integer array of |
|
integer array of |
|
block dimension of sparse matrix A; must be larger than zero. |
|
structure initialized using |
|
the supported policies are |
|
buffer allocated by the user; the size is returned by |
Output
|
Structure filled with information collected during the analysis phase (that should be passed to the solve phase unchanged). |
See cusparseStatus_t for the description of the return status.
11.1.7. cusparse<t>bsric02()
cusparseStatus_t
cusparseSbsric02(cusparseHandle_t handle,
cusparseDirection_t dirA,
int mb,
int nnzb,
const cusparseMatDescr_t descrA,
float* bsrValA,
const int* bsrRowPtrA,
const int* bsrColIndA,
int blockDim,
bsric02Info_t info,
cusparseSolvePolicy_t policy,
void* pBuffer)
cusparseStatus_t
cusparseDbsric02(cusparseHandle_t handle,
cusparseDirection_t dirA,
int mb,
int nnzb,
const cusparseMatDescr_t descrA,
double* bsrValA,
const int* bsrRowPtrA,
const int* bsrColIndA,
int blockDim,
bsric02Info_t info,
cusparseSolvePolicy_t policy,
void* pBuffer)
cusparseStatus_t
cusparseCbsric02(cusparseHandle_t handle,
cusparseDirection_t dirA,
int mb,
int nnzb,
const cusparseMatDescr_t descrA,
cuComplex* bsrValA,
const int* bsrRowPtrA,
const int* bsrColIndA,
int blockDim,
bsric02Info_t info,
cusparseSolvePolicy_t policy,
void* pBuffer)
cusparseStatus_t
cusparseZbsric02(cusparseHandle_t handle,
cusparseDirection_t dirA,
int mb,
int nnzb,
const cusparseMatDescr_t descrA,
cuDoubleComplex* bsrValA,
const int* bsrRowPtrA,
const int* bsrColIndA,
int blockDim,
bsric02Info_t info,
cusparseSolvePolicy_t policy,
void* pBuffer)
This function performs the solve phase of the incomplete-Cholesky factorization with 0 fill-in and no pivoting
\(A \approx LL^{H}\) |
A
is an (mb*blockDim)×(mb*blockDim)
sparse matrix that is defined in BSR storage format by the three arrays bsrValA
, bsrRowPtrA
, and bsrColIndA
. The block in BSR format is of size blockDim*blockDim
, stored as column-major or row-major as determined by parameter dirA
, which is either CUSPARSE_DIRECTION_COLUMN
or CUSPARSE_DIRECTION_ROW
. The matrix type must be CUSPARSE_MATRIX_TYPE_GENERAL
, and the fill mode and diagonal type are ignored.
This function requires a buffer size returned by bsric02_bufferSize()
. The address of pBuffer
must be a multiple of 128 bytes. If it is not, CUSPARSE_STATUS_INVALID_VALUE
is returned.
Although bsric02()
can be done without level information, the user must be aware of consistency. If bsric02_analysis()
is called with policy CUSPARSE_SOLVE_POLICY_USE_LEVEL
, bsric02()
can be run with or without levels. On the other hand, if bsric02_analysis()
is called with CUSPARSE_SOLVE_POLICY_NO_LEVEL
, bsric02()
can only accept CUSPARSE_SOLVE_POLICY_NO_LEVEL
; otherwise, CUSPARSE_STATUS_INVALID_VALUE
is returned.
Function bsric02()
has the same behavior as csric02()
. That is, bsr2csr(bsric02(A)) = csric02(bsr2csr(A))
. The numerical zero of csric02()
means there exists some zero L(j,j)
. The numerical zero of bsric02()
means there exists some block Lj,j)
that is not invertible.
Function bsric02
reports the first numerical zero, including a structural zero. The user must call cusparseXbsric02_zeroPivot()
to know where the numerical zero is.
The bsric02()
function only takes the lower triangular part of matrix A
to perform factorization. The strictly upper triangular part is ignored and never touched. It does not matter if A
is Hermitian or not. In other words, from the point of view of bsric02()
, A
is Hermitian and only the lower triangular part is provided. Moreover, the imaginary part of diagonal elements of diagonal blocks is ignored.
For example, suppose A
is a real m-by-m matrix, where m=mb*blockDim
. The following code solves precondition system M*y = x
, where M
is the product of Cholesky factorization L
and its transpose.
\(M = LL^{H}\) |
// Suppose that A is m x m sparse matrix represented by BSR format,
// The number of block rows/columns is mb, and
// the number of nonzero blocks is nnzb.
// Assumption:
// - handle is already created by cusparseCreate(),
// - (d_bsrRowPtr, d_bsrColInd, d_bsrVal) is BSR of A on device memory,
// - d_x is right hand side vector on device memory,
// - d_y is solution vector on device memory.
// - d_z is intermediate result on device memory.
// - d_x, d_y and d_z are of size m.
cusparseMatDescr_t descr_M = 0;
cusparseMatDescr_t descr_L = 0;
bsric02Info_t info_M = 0;
bsrsv2Info_t info_L = 0;
bsrsv2Info_t info_Lt = 0;
int pBufferSize_M;
int pBufferSize_L;
int pBufferSize_Lt;
int pBufferSize;
void *pBuffer = 0;
int structural_zero;
int numerical_zero;
const double alpha = 1.;
const cusparseSolvePolicy_t policy_M = CUSPARSE_SOLVE_POLICY_NO_LEVEL;
const cusparseSolvePolicy_t policy_L = CUSPARSE_SOLVE_POLICY_NO_LEVEL;
const cusparseSolvePolicy_t policy_Lt = CUSPARSE_SOLVE_POLICY_USE_LEVEL;
const cusparseOperation_t trans_L = CUSPARSE_OPERATION_NON_TRANSPOSE;
const cusparseOperation_t trans_Lt = CUSPARSE_OPERATION_TRANSPOSE;
const cusparseDirection_t dir = CUSPARSE_DIRECTION_COLUMN;
// step 1: create a descriptor which contains
// - matrix M is base-1
// - matrix L is base-1
// - matrix L is lower triangular
// - matrix L has non-unit diagonal
cusparseCreateMatDescr(&descr_M);
cusparseSetMatIndexBase(descr_M, CUSPARSE_INDEX_BASE_ONE);
cusparseSetMatType(descr_M, CUSPARSE_MATRIX_TYPE_GENERAL);
cusparseCreateMatDescr(&descr_L);
cusparseSetMatIndexBase(descr_L, CUSPARSE_INDEX_BASE_ONE);
cusparseSetMatType(descr_L, CUSPARSE_MATRIX_TYPE_GENERAL);
cusparseSetMatFillMode(descr_L, CUSPARSE_FILL_MODE_LOWER);
cusparseSetMatDiagType(descr_L, CUSPARSE_DIAG_TYPE_NON_UNIT);
// step 2: create a empty info structure
// we need one info for bsric02 and two info's for bsrsv2
cusparseCreateBsric02Info(&info_M);
cusparseCreateBsrsv2Info(&info_L);
cusparseCreateBsrsv2Info(&info_Lt);
// step 3: query how much memory used in bsric02 and bsrsv2, and allocate the buffer
cusparseDbsric02_bufferSize(handle, dir, mb, nnzb,
descr_M, d_bsrVal, d_bsrRowPtr, d_bsrColInd, blockDim, info_M, &bufferSize_M);
cusparseDbsrsv2_bufferSize(handle, dir, trans_L, mb, nnzb,
descr_L, d_bsrVal, d_bsrRowPtr, d_bsrColInd, blockDim, info_L, &pBufferSize_L);
cusparseDbsrsv2_bufferSize(handle, dir, trans_Lt, mb, nnzb,
descr_L, d_bsrVal, d_bsrRowPtr, d_bsrColInd, blockDim, info_Lt, &pBufferSize_Lt);
pBufferSize = max(bufferSize_M, max(pBufferSize_L, pBufferSize_Lt));
// pBuffer returned by cudaMalloc is automatically aligned to 128 bytes.
cudaMalloc((void**)&pBuffer, pBufferSize);
// step 4: perform analysis of incomplete Cholesky on M
// perform analysis of triangular solve on L
// perform analysis of triangular solve on L'
// The lower triangular part of M has the same sparsity pattern as L, so
// we can do analysis of bsric02 and bsrsv2 simultaneously.
cusparseDbsric02_analysis(handle, dir, mb, nnzb, descr_M,
d_bsrVal, d_bsrRowPtr, d_bsrColInd, blockDim, info_M,
policy_M, pBuffer);
status = cusparseXbsric02_zeroPivot(handle, info_M, &structural_zero);
if (CUSPARSE_STATUS_ZERO_PIVOT == status){
printf("A(%d,%d) is missing\n", structural_zero, structural_zero);
}
cusparseDbsrsv2_analysis(handle, dir, trans_L, mb, nnzb, descr_L,
d_bsrVal, d_bsrRowPtr, d_bsrColInd, blockDim,
info_L, policy_L, pBuffer);
cusparseDbsrsv2_analysis(handle, dir, trans_Lt, mb, nnzb, descr_L,
d_bsrVal, d_bsrRowPtr, d_bsrColInd, blockDim,
info_Lt, policy_Lt, pBuffer);
// step 5: M = L * L'
cusparseDbsric02_solve(handle, dir, mb, nnzb, descr_M,
d_bsrVal, d_bsrRowPtr, d_bsrColInd, blockDim, info_M, policy_M, pBuffer);
status = cusparseXbsric02_zeroPivot(handle, info_M, &numerical_zero);
if (CUSPARSE_STATUS_ZERO_PIVOT == status){
printf("L(%d,%d) is not positive definite\n", numerical_zero, numerical_zero);
}
// step 6: solve L*z = x
cusparseDbsrsv2_solve(handle, dir, trans_L, mb, nnzb, &alpha, descr_L,
d_bsrVal, d_bsrRowPtr, d_bsrColInd, blockDim, info_L,
d_x, d_z, policy_L, pBuffer);
// step 7: solve L'*y = z
cusparseDbsrsv2_solve(handle, dir, trans_Lt, mb, nnzb, &alpha, descr_L,
d_bsrVal, d_bsrRowPtr, d_bsrColInd, blockDim, info_Lt,
d_z, d_y, policy_Lt, pBuffer);
// step 6: free resources
cudaFree(pBuffer);
cusparseDestroyMatDescr(descr_M);
cusparseDestroyMatDescr(descr_L);
cusparseDestroyBsric02Info(info_M);
cusparseDestroyBsrsv2Info(info_L);
cusparseDestroyBsrsv2Info(info_Lt);
cusparseDestroy(handle);
The function supports the following properties if pBuffer != NULL
The routine requires no extra storage
The routine supports asynchronous execution
The routine supports CUDA graph capture
Input
|
handle to the cuSPARSE library context. |
|
storage format of blocks, either |
|
number of block rows and block columns of matrix |
|
number of nonzero blocks of matrix |
|
the descriptor of matrix |
|
<type> array of |
|
integer array of |
|
integer array of |
|
block dimension of sparse matrix A, larger than zero. |
|
structure with information collected during the analysis phase (that should have been passed to the solve phase unchanged). |
|
the supported policies are |
|
buffer allocated by the user, the size is returned by |
Output
|
<type> matrix containing the incomplete-Cholesky lower triangular factor. |
See cusparseStatus_t for the description of the return status.
11.1.8. cusparseXbsric02_zeroPivot()
cusparseStatus_t
cusparseXbsric02_zeroPivot(cusparseHandle_t handle,
bsric02Info_t info,
int* position)
If the returned error code is CUSPARSE_STATUS_ZERO_PIVOT
, position=j
means A(j,j)
has either a structural zero or a numerical zero (the block is not positive definite). Otherwise position=-1
.
The position
can be 0-based or 1-based, the same as the matrix.
Function cusparseXbsric02_zeroPivot()
is a blocking call. It calls cudaDeviceSynchronize()
to make sure all previous kernels are done.
The position
can be in the host memory or device memory. The user can set the proper mode with cusparseSetPointerMode()
.
The routine requires no extra storage
The routine supports asynchronous execution if the Stream Ordered Memory Allocator is available
The routine supports CUDA graph capture if the Stream Ordered Memory Allocator is available
Input
|
handle to the cuSPARSE library context. |
|
|
Output
|
If no structural or numerical zero, |
See cusparseStatus_t for the description of the return status.
11.2. Incomplete LU Factorization: level 0
Different algorithms for ilu0 are discussed in this section.
11.2.1. cusparse<t>csrilu02_numericBoost()
cusparseStatus_t
cusparseScsrilu02_numericBoost(cusparseHandle_t handle,
csrilu02Info_t info,
int enable_boost,
double* tol,
float* boost_val)
cusparseStatus_t
cusparseDcsrilu02_numericBoost(cusparseHandle_t handle,
csrilu02Info_t info,
int enable_boost,
double* tol,
double* boost_val)
cusparseStatus_t
cusparseCcsrilu02_numericBoost(cusparseHandle_t handle,
csrilu02Info_t info,
int enable_boost,
double* tol,
cuComplex* boost_val)
cusparseStatus_t
cusparseZcsrilu02_numericBoost(cusparseHandle_t handle,
csrilu02Info_t info,
int enable_boost,
double* tol,
cuDoubleComplex* boost_val)
The user can use a boost value to replace a numerical value in incomplete LU factorization. The tol
is used to determine a numerical zero, and the boost_val
is used to replace a numerical zero. The behavior is
if tol >= fabs(A(j,j))
, then A(j,j)=boost_val
.
To enable a boost value, the user has to set parameter enable_boost
to 1 before calling csrilu02()
. To disable a boost value, the user can call csrilu02_numericBoost()
again with parameter enable_boost=0
.
If enable_boost=0
, tol
and boost_val
are ignored.
Both tol
and boost_val
can be in the host memory or device memory. The user can set the proper mode with cusparseSetPointerMode()
.
The routine requires no extra storage
The routine supports asynchronous execution
The routine supports CUDA graph capture
Input
|
handle to the cuSPARSE library context |
|
structure initialized using |
|
disable boost by |
|
tolerance to determine a numerical zero |
|
boost value to replace a numerical zero |
See cusparseStatus_t for the description of the return status.
11.2.2. cusparse<t>csrilu02_bufferSize()
cusparseStatus_t
cusparseScsrilu02_bufferSize(cusparseHandle_t handle,
int m,
int nnz,
const cusparseMatDescr_t descrA,
float* csrValA,
const int* csrRowPtrA,
const int* csrColIndA,
csrilu02Info_t info,
int* pBufferSizeInBytes)
cusparseStatus_t
cusparseDcsrilu02_bufferSize(cusparseHandle_t handle,
int m,
int nnz,
const cusparseMatDescr_t descrA,
double* csrValA,
const int* csrRowPtrA,
const int* csrColIndA,
csrilu02Info_t info,
int* pBufferSizeInBytes)
cusparseStatus_t
cusparseCcsrilu02_bufferSize(cusparseHandle_t handle,
int m,
int nnz,
const cusparseMatDescr_t descrA,
cuComplex* csrValA,
const int* csrRowPtrA,
const int* csrColIndA,
csrilu02Info_t info,
int* pBufferSizeInBytes)
cusparseStatus_t
cusparseZcsrilu02_bufferSize(cusparseHandle_t handle,
int m,
int nnz,
const cusparseMatDescr_t descrA,
cuDoubleComplex* csrValA,
const int* csrRowPtrA,
const int* csrColIndA,
csrilu02Info_t info,
int* pBufferSizeInBytes)
This function returns size of the buffer used in computing the incomplete-LU factorization with \(0\) fill-in and no pivoting:
\(A \approx LU\) |
A
is an m×m
sparse matrix that is defined in CSR storage format by the three arrays csrValA
, csrRowPtrA
, and csrColIndA
.
The buffer size depends on the dimension m
and nnz
, the number of nonzeros of the matrix. If the user changes the matrix, it is necessary to call csrilu02_bufferSize()
again to have the correct buffer size; otherwise, a segmentation fault may occur.
The routine requires no extra storage
The routine supports asynchronous execution
The routine supports CUDA graph capture
Input
|
handle to the cuSPARSE library context. |
|
number of rows and columns of matrix |
|
number of nonzeros of matrix |
|
the descriptor of matrix |
|
<type> array of |
|
integer array of |
|
integer array of |
Output
|
record internal states based on different algorithms |
|
number of bytes of the buffer used in |
See cusparseStatus_t for the description of the return status.
11.2.3. cusparse<t>csrilu02_analysis()
cusparseStatus_t
cusparseScsrilu02_analysis(cusparseHandle_t handle,
int m,
int nnz,
const cusparseMatDescr_t descrA,
const float* csrValA,
const int* csrRowPtrA,
const int* csrColIndA,
csrilu02Info_t info,
cusparseSolvePolicy_t policy,
void* pBuffer)
cusparseStatus_t
cusparseDcsrilu02_analysis(cusparseHandle_t handle,
int m,
int nnz,
const cusparseMatDescr_t descrA,
const double* csrValA,
const int* csrRowPtrA,
const int* csrColIndA,
csrilu02Info_t info,
cusparseSolvePolicy_t policy,
void* pBuffer)
cusparseStatus_t
cusparseCcsrilu02_analysis(cusparseHandle_t handle,
int m,
int nnz,
const cusparseMatDescr_t descrA,
const cuComplex* csrValA,
const int* csrRowPtrA,
const int* csrColIndA,
csrilu02Info_t info,
cusparseSolvePolicy_t policy,
void* pBuffer)
cusparseStatus_t
cusparseZcsrilu02_analysis(cusparseHandle_t handle,
int m,
int nnz,
const cusparseMatDescr_t descrA,
const cuDoubleComplex* csrValA,
const int* csrRowPtrA,
const int* csrColIndA,
csrilu02Info_t info,
cusparseSolvePolicy_t policy,
void* pBuffer)
This function performs the analysis phase of the incomplete-LU factorization with \(0\) fill-in and no pivoting:
\(A \approx LU\) |
A
is an m×m
sparse matrix that is defined in CSR storage format by the three arrays csrValA
, csrRowPtrA
, and csrColIndA
.
This function requires the buffer size returned by csrilu02_bufferSize()
. The address of pBuffer
must be a multiple of 128 bytes. If not, CUSPARSE_STATUS_INVALID_VALUE
is returned.
Function csrilu02_analysis()
reports a structural zero and computes level information stored in the opaque structure info
. The level information can extract more parallelism during incomplete LU factorization; however csrilu02()
can be done without level information. To disable level information, the user must specify the policy of csrilu02()
as CUSPARSE_SOLVE_POLICY_NO_LEVEL
.
It is the user’s choice whether to call csrilu02()
if csrilu02_analysis()
reports a structural zero. In this case, the user can still call csrilu02()
, which will return a numerical zero at the same position as the structural zero. However the result is meaningless.
This function requires temporary extra storage that is allocated internally
The routine supports asynchronous execution if the Stream Ordered Memory Allocator is available
The routine supports CUDA graph capture if the Stream Ordered Memory Allocator is available
Input
|
handle to the cuSPARSE library context. |
|
number of rows and columns of matrix |
|
number of nonzeros of matrix |
|
the descriptor of matrix |
|
<type> array of |
|
integer array of |
|
integer array of |
|
structure initialized using |
|
the supported policies are |
|
buffer allocated by the user, the size is returned by |
Output
|
Structure filled with information collected during the analysis phase (that should be passed to the solve phase unchanged). |
See cusparseStatus_t for the description of the return status.
11.2.4. cusparse<t>csrilu02()
cusparseStatus_t
cusparseScsrilu02(cusparseHandle_t handle,
int m,
int nnz,
const cusparseMatDescr_t descrA,
float* csrValA_valM,
const int* csrRowPtrA,
const int* csrColIndA,
csrilu02Info_t info,
cusparseSolvePolicy_t policy,
void* pBuffer)
cusparseStatus_t
cusparseDcsrilu02(cusparseHandle_t handle,
int m,
int nnz,
const cusparseMatDescr_t descrA,
double* csrValA_valM,
const int* csrRowPtrA,
const int* csrColIndA,
csrilu02Info_t info,
cusparseSolvePolicy_t policy,
void* pBuffer)
cusparseStatus_t
cusparseCcsrilu02(cusparseHandle_t handle,
int m,
int nnz,
const cusparseMatDescr_t descrA,
cuComplex* csrValA_valM,
const int* csrRowPtrA,
const int* csrColIndA,
csrilu02Info_t info,
cusparseSolvePolicy_t policy,
void* pBuffer)
cusparseStatus_t
cusparseZcsrilu02(cusparseHandle_t handle,
int m,
int nnz,
const cusparseMatDescr_t descrA,
cuDoubleComplex* csrValA_valM,
const int* csrRowPtrA,
const int* csrColIndA,
csrilu02Info_t info,
cusparseSolvePolicy_t policy,
void* pBuffer)
This function performs the solve phase of the incomplete-LU factorization with \(0\) fill-in and no pivoting:
\(A \approx LU\) |
A
is an m×m
sparse matrix that is defined in CSR storage format by the three arrays csrValA_valM
, csrRowPtrA
, and csrColIndA
.
This function requires a buffer size returned by csrilu02_bufferSize()
. The address of pBuffer
must be a multiple of 128 bytes. If not, CUSPARSE_STATUS_INVALID_VALUE
is returned.
The matrix type must be CUSPARSE_MATRIX_TYPE_GENERAL
. The fill mode and diagonal type are ignored.
Although csrilu02()
can be done without level information, the user still needs to be aware of consistency. If csrilu02_analysis()
is called with policy CUSPARSE_SOLVE_POLICY_USE_LEVEL
, csrilu02()
can be run with or without levels. On the other hand, if csrilu02_analysis()
is called with CUSPARSE_SOLVE_POLICY_NO_LEVEL
, csrilu02()
can only accept CUSPARSE_SOLVE_POLICY_NO_LEVEL
; otherwise, CUSPARSE_STATUS_INVALID_VALUE
is returned.
Function csrilu02()
reports the first numerical zero, including a structural zero. The user must call cusparseXcsrilu02_zeroPivot()
to know where the numerical zero is.
For example, suppose A
is a real m × m
matrix, the following code solves precondition system M*y = x
where M
is the product of LU factors L
and U
.
// Suppose that A is m x m sparse matrix represented by CSR format,
// Assumption:
// - handle is already created by cusparseCreate(),
// - (d_csrRowPtr, d_csrColInd, d_csrVal) is CSR of A on device memory,
// - d_x is right hand side vector on device memory,
// - d_y is solution vector on device memory.
// - d_z is intermediate result on device memory.
cusparseMatDescr_t descr_M = 0;
cusparseMatDescr_t descr_L = 0;
cusparseMatDescr_t descr_U = 0;
csrilu02Info_t info_M = 0;
csrsv2Info_t info_L = 0;
csrsv2Info_t info_U = 0;
int pBufferSize_M;
int pBufferSize_L;
int pBufferSize_U;
int pBufferSize;
void *pBuffer = 0;
int structural_zero;
int numerical_zero;
const double alpha = 1.;
const cusparseSolvePolicy_t policy_M = CUSPARSE_SOLVE_POLICY_NO_LEVEL;
const cusparseSolvePolicy_t policy_L = CUSPARSE_SOLVE_POLICY_NO_LEVEL;
const cusparseSolvePolicy_t policy_U = CUSPARSE_SOLVE_POLICY_USE_LEVEL;
const cusparseOperation_t trans_L = CUSPARSE_OPERATION_NON_TRANSPOSE;
const cusparseOperation_t trans_U = CUSPARSE_OPERATION_NON_TRANSPOSE;
// step 1: create a descriptor which contains
// - matrix M is base-1
// - matrix L is base-1
// - matrix L is lower triangular
// - matrix L has unit diagonal
// - matrix U is base-1
// - matrix U is upper triangular
// - matrix U has non-unit diagonal
cusparseCreateMatDescr(&descr_M);
cusparseSetMatIndexBase(descr_M, CUSPARSE_INDEX_BASE_ONE);
cusparseSetMatType(descr_M, CUSPARSE_MATRIX_TYPE_GENERAL);
cusparseCreateMatDescr(&descr_L);
cusparseSetMatIndexBase(descr_L, CUSPARSE_INDEX_BASE_ONE);
cusparseSetMatType(descr_L, CUSPARSE_MATRIX_TYPE_GENERAL);
cusparseSetMatFillMode(descr_L, CUSPARSE_FILL_MODE_LOWER);
cusparseSetMatDiagType(descr_L, CUSPARSE_DIAG_TYPE_UNIT);
cusparseCreateMatDescr(&descr_U);
cusparseSetMatIndexBase(descr_U, CUSPARSE_INDEX_BASE_ONE);
cusparseSetMatType(descr_U, CUSPARSE_MATRIX_TYPE_GENERAL);
cusparseSetMatFillMode(descr_U, CUSPARSE_FILL_MODE_UPPER);
cusparseSetMatDiagType(descr_U, CUSPARSE_DIAG_TYPE_NON_UNIT);
// step 2: create a empty info structure
// we need one info for csrilu02 and two info's for csrsv2
cusparseCreateCsrilu02Info(&info_M);
cusparseCreateCsrsv2Info(&info_L);
cusparseCreateCsrsv2Info(&info_U);
// step 3: query how much memory used in csrilu02 and csrsv2, and allocate the buffer
cusparseDcsrilu02_bufferSize(handle, m, nnz,
descr_M, d_csrVal, d_csrRowPtr, d_csrColInd, info_M, &pBufferSize_M);
cusparseDcsrsv2_bufferSize(handle, trans_L, m, nnz,
descr_L, d_csrVal, d_csrRowPtr, d_csrColInd, info_L, &pBufferSize_L);
cusparseDcsrsv2_bufferSize(handle, trans_U, m, nnz,
descr_U, d_csrVal, d_csrRowPtr, d_csrColInd, info_U, &pBufferSize_U);
pBufferSize = max(pBufferSize_M, max(pBufferSize_L, pBufferSize_U));
// pBuffer returned by cudaMalloc is automatically aligned to 128 bytes.
cudaMalloc((void**)&pBuffer, pBufferSize);
// step 4: perform analysis of incomplete Cholesky on M
// perform analysis of triangular solve on L
// perform analysis of triangular solve on U
// The lower(upper) triangular part of M has the same sparsity pattern as L(U),
// we can do analysis of csrilu0 and csrsv2 simultaneously.
cusparseDcsrilu02_analysis(handle, m, nnz, descr_M,
d_csrVal, d_csrRowPtr, d_csrColInd, info_M,
policy_M, pBuffer);
status = cusparseXcsrilu02_zeroPivot(handle, info_M, &structural_zero);
if (CUSPARSE_STATUS_ZERO_PIVOT == status){
printf("A(%d,%d) is missing\n", structural_zero, structural_zero);
}
cusparseDcsrsv2_analysis(handle, trans_L, m, nnz, descr_L,
d_csrVal, d_csrRowPtr, d_csrColInd,
info_L, policy_L, pBuffer);
cusparseDcsrsv2_analysis(handle, trans_U, m, nnz, descr_U,
d_csrVal, d_csrRowPtr, d_csrColInd,
info_U, policy_U, pBuffer);
// step 5: M = L * U
cusparseDcsrilu02(handle, m, nnz, descr_M,
d_csrVal, d_csrRowPtr, d_csrColInd, info_M, policy_M, pBuffer);
status = cusparseXcsrilu02_zeroPivot(handle, info_M, &numerical_zero);
if (CUSPARSE_STATUS_ZERO_PIVOT == status){
printf("U(%d,%d) is zero\n", numerical_zero, numerical_zero);
}
// step 6: solve L*z = x
cusparseDcsrsv2_solve(handle, trans_L, m, nnz, &alpha, descr_L,
d_csrVal, d_csrRowPtr, d_csrColInd, info_L,
d_x, d_z, policy_L, pBuffer);
// step 7: solve U*y = z
cusparseDcsrsv2_solve(handle, trans_U, m, nnz, &alpha, descr_U,
d_csrVal, d_csrRowPtr, d_csrColInd, info_U,
d_z, d_y, policy_U, pBuffer);
// step 6: free resources
cudaFree(pBuffer);
cusparseDestroyMatDescr(descr_M);
cusparseDestroyMatDescr(descr_L);
cusparseDestroyMatDescr(descr_U);
cusparseDestroyCsrilu02Info(info_M);
cusparseDestroyCsrsv2Info(info_L);
cusparseDestroyCsrsv2Info(info_U);
cusparseDestroy(handle);
The function supports the following properties if pBuffer != NULL
The routine requires no extra storage
The routine supports asynchronous execution
The routine supports CUDA graph capture
Input
|
handle to the cuSPARSE library context. |
|
number of rows and columns of matrix |
|
number of nonzeros of matrix |
|
the descriptor of matrix |
|
<type> array of |
|
integer array of |
|
integer array of |
|
structure with information collected during the analysis phase (that should have been passed to the solve phase unchanged). |
|
the supported policies are |
|
buffer allocated by the user; the size is returned by |
Output
|
<type> matrix containing the incomplete-LU lower and upper triangular factors. |
See cusparseStatus_t for the description of the return status.
11.2.5. cusparseXcsrilu02_zeroPivot()
cusparseStatus_t
cusparseXcsrilu02_zeroPivot(cusparseHandle_t handle,
csrilu02Info_t info,
int* position)
If the returned error code is CUSPARSE_STATUS_ZERO_PIVOT
, position=j
means A(j,j)
has either a structural zero or a numerical zero; otherwise, position=-1
.
The position
can be 0-based or 1-based, the same as the matrix.
Function cusparseXcsrilu02_zeroPivot()
is a blocking call. It calls cudaDeviceSynchronize(
) to make sure all previous kernels are done.
The position
can be in the host memory or device memory. The user can set proper mode with cusparseSetPointerMode()
.
The routine requires no extra storage
The routine supports asynchronous execution if the Stream Ordered Memory Allocator is available
The routine supports CUDA graph capture if the Stream Ordered Memory Allocator is available
Input
|
Handle to the cuSPARSE library context. |
|
|
Output
|
If no structural or numerical zero, |
See cusparseStatus_t for the description of the return status.
11.2.6. cusparse<t>bsrilu02_numericBoost()
cusparseStatus_t
cusparseSbsrilu02_numericBoost(cusparseHandle_t handle,
bsrilu02Info_t info,
int enable_boost,
double* tol,
float* boost_val)
cusparseStatus_t
cusparseDbsrilu02_numericBoost(cusparseHandle_t handle,
bsrilu02Info_t info,
int enable_boost,
double* tol,
double* boost_val)
cusparseStatus_t
cusparseCbsrilu02_numericBoost(cusparseHandle_t handle,
bsrilu02Info_t info,
int enable_boost,
double* tol,
cuComplex* boost_val)
cusparseStatus_t
cusparseZbsrilu02_numericBoost(cusparseHandle_t handle,
bsrilu02Info_t info,
int enable_boost,
double* tol,
cuDoubleComplex* boost_val)
The user can use a boost value to replace a numerical value in incomplete LU factorization. Parameter tol
is used to determine a numerical zero, and boost_val
is used to replace a numerical zero. The behavior is as follows:
if tol >= fabs(A(j,j))
, then reset each diagonal element of block A(j,j)
by boost_val
.
To enable a boost value, the user sets parameter enable_boost
to 1 before calling bsrilu02()
. To disable the boost value, the user can call bsrilu02_numericBoost()
with parameter enable_boost=0
.
If enable_boost=0
, tol
and boost_val
are ignored.
Both tol
and boost_val
can be in host memory or device memory. The user can set the proper mode with cusparseSetPointerMode()
.
The routine requires no extra storage
The routine supports asynchronous execution
The routine supports CUDA graph capture
Input
|
handle to the cuSPARSE library context. |
|
structure initialized using |
|
disable boost by setting |
|
tolerance to determine a numerical zero. |
|
boost value to replace a numerical zero. |
See cusparseStatus_t for the description of the return status.
11.2.7. cusparse<t>bsrilu02_bufferSize()
cusparseStatus_t
cusparseSbsrilu02_bufferSize(cusparseHandle_t handle,
cusparseDirection_t dirA,
int mb,
int nnzb,
const cusparseMatDescr_t descrA,
float *bsrValA,
const int *bsrRowPtrA,
const int *bsrColIndA,
int blockDim,
bsrilu02Info_t info,
int *pBufferSizeInBytes);
cusparseStatus_t
cusparseDbsrilu02_bufferSize(cusparseHandle_t handle,
cusparseDirection_t dirA,
int mb,
int nnzb,
const cusparseMatDescr_t descrA,
double *bsrValA,
const int *bsrRowPtrA,
const int *bsrColIndA,
int blockDim,
bsrilu02Info_t info,
int *pBufferSizeInBytes);
cusparseStatus_t
cusparseCbsrilu02_bufferSize(cusparseHandle_t handle,
cusparseDirection_t dirA,
int mb,
int nnzb,
const cusparseMatDescr_t descrA,
cuComplex *bsrValA,
const int *bsrRowPtrA,
const int *bsrColIndA,
int blockDim,
bsrilu02Info_t info,
int *pBufferSizeInBytes);
cusparseStatus_t
cusparseZbsrilu02_bufferSize(cusparseHandle_t handle,
cusparseDirection_t dirA,
int mb,
int nnzb,
const cusparseMatDescr_t descrA,
cuDoubleComplex *bsrValA,
const int *bsrRowPtrA,
const int *bsrColIndA,
int blockDim,
bsrilu02Info_t info,
int *pBufferSizeInBytes);
This function returns the size of the buffer used in computing the incomplete-LU factorization with 0 fill-in and no pivoting.
\(A \approx LU\) |
A
is an (mb*blockDim)*(mb*blockDim)
sparse matrix that is defined in BSR storage format by the three arrays bsrValA
, bsrRowPtrA
, and bsrColIndA
.
The buffer size depends on the dimensions of mb
, blockDim
, and the number of nonzero blocks of the matrix nnzb
. If the user changes the matrix, it is necessary to call bsrilu02_bufferSize()
again to have the correct buffer size; otherwise, a segmentation fault may occur.
Input
|
handle to the cuSPARSE library context. |
|
storage format of blocks, either |
|
number of block rows and columns of matrix |
|
number of nonzero blocks of matrix |
|
the descriptor of matrix |
|
<type> array of |
|
integer array of |
|
integer array of |
|
block dimension of sparse matrix A, larger than zero. |
Output
|
record internal states based on different algorithms. |
|
number of bytes of the buffer used in |
Status Returned
|
the operation completed successfully. |
|
the library was not initialized. |
|
the resources could not be allocated. |
|
invalid parameters were passed ( |
|
the device only supports compute capability 2.0 and above. |
|
an internal operation failed. |
|
the matrix type is not supported. |
11.2.8. cusparse<t>bsrilu02_analysis()
cusparseStatus_t
cusparseSbsrilu02_analysis(cusparseHandle_t handle,
cusparseDirection_t dirA,
int mb,
int nnzb,
const cusparseMatDescr_t descrA,
float* bsrValA,
const int* bsrRowPtrA,
const int* bsrColIndA,
int blockDim,
bsrilu02Info_t info,
cusparseSolvePolicy_t policy,
void* pBuffer)
cusparseStatus_t
cusparseDbsrilu02_analysis(cusparseHandle_t handle,
cusparseDirection_t dirA,
int mb,
int nnzb,
const cusparseMatDescr_t descrA,
double* bsrValA,
const int* bsrRowPtrA,
const int* bsrColIndA,
int blockDim,
bsrilu02Info_t info,
cusparseSolvePolicy_t policy,
void* pBuffer)
cusparseStatus_t
cusparseCbsrilu02_analysis(cusparseHandle_t handle,
cusparseDirection_t dirA,
int mb,
int nnzb,
const cusparseMatDescr_t descrA,
cuComplex* bsrValA,
const int* bsrRowPtrA,
const int* bsrColIndA,
int blockDim,
bsrilu02Info_t info,
cusparseSolvePolicy_t policy,
void* pBuffer)
cusparseStatus_t
cusparseZbsrilu02_analysis(cusparseHandle_t handle,
cusparseDirection_t dirA,
int mb,
int nnzb,
const cusparseMatDescr_t descrA,
cuDoubleComplex* bsrValA,
const int* bsrRowPtrA,
const int* bsrColIndA,
int blockDim,
bsrilu02Info_t info,
cusparseSolvePolicy_t policy,
void* pBuffer)
This function performs the analysis phase of the incomplete-LU factorization with 0 fill-in and no pivoting.
\(A \approx LU\) |
A
is an (mb*blockDim)×(mb*blockDim)
sparse matrix that is defined in BSR storage format by the three arrays bsrValA
, bsrRowPtrA
, and bsrColIndA
. The block in BSR format is of size blockDim*blockDim
, stored as column-major or row-major as determined by parameter dirA
, which is either CUSPARSE_DIRECTION_COLUMN
or CUSPARSE_DIRECTION_ROW
. The matrix type must be CUSPARSE_MATRIX_TYPE_GENERAL
, and the fill mode and diagonal type are ignored.
This function requires a buffer size returned by bsrilu02_bufferSize()
. The address of pBuffer
must be multiple of 128 bytes. If it is not, CUSPARSE_STATUS_INVALID_VALUE
is returned.
Function bsrilu02_analysis()
reports a structural zero and computes level information stored in the opaque structure info
. The level information can extract more parallelism during incomplete LU factorization. However bsrilu02()
can be done without level information. To disable level information, the user needs to specify the parameter policy
of bsrilu02[_analysis| ]
as CUSPARSE_SOLVE_POLICY_NO_LEVEL
.
Function bsrilu02_analysis()
always reports the first structural zero, even with parameter policy
is CUSPARSE_SOLVE_POLICY_NO_LEVEL
. The user must call cusparseXbsrilu02_zeroPivot()
to know where the structural zero is.
It is the user’s choice whether to call bsrilu02()
if bsrilu02_analysis()
reports a structural zero. In this case, the user can still call bsrilu02()
, which will return a numerical zero at the same position as the structural zero. However the result is meaningless.
This function requires temporary extra storage that is allocated internally
The routine supports asynchronous execution if the Stream Ordered Memory Allocator is available
The routine supports CUDA graph capture if the Stream Ordered Memory Allocator is available
Input
|
handle to the cuSPARSE library context. |
|
storage format of blocks, either |
|
number of block rows and block columns of matrix |
|
number of nonzero blocks of matrix |
|
the descriptor of matrix |
|
<type> array of |
|
integer array of |
|
integer array of |
|
block dimension of sparse matrix A, larger than zero. |
|
structure initialized using |
|
the supported policies are |
|
buffer allocated by the user, the size is returned by |
Output
|
structure filled with information collected during the analysis phase (that should be passed to the solve phase unchanged) |
See cusparseStatus_t for the description of the return status.
11.2.9. cusparse<t>bsrilu02()
cusparseStatus_t
cusparseSbsrilu02(cusparseHandle_t handle,
cusparseDirection_t dirA,
int mb,
int nnzb,
const cusparseMatDescr_t descry,
float* bsrValA,
const int* bsrRowPtrA,
const int* bsrColIndA,
int blockDim,
bsrilu02Info_t info,
cusparseSolvePolicy_t policy,
void* pBuffer)
cusparseStatus_t
cusparseDbsrilu02(cusparseHandle_t handle,
cusparseDirection_t dirA,
int mb,
int nnzb,
const cusparseMatDescr_t descry,
double* bsrValA,
const int* bsrRowPtrA,
const int* bsrColIndA,
int blockDim,
bsrilu02Info_t info,
cusparseSolvePolicy_t policy,
void* pBuffer)
cusparseStatus_t
cusparseCbsrilu02(cusparseHandle_t handle,
cusparseDirection_t dirA,
int mb,
int nnzb,
const cusparseMatDescr_t descry,
cuComplex* bsrValA,
const int* bsrRowPtrA,
const int* bsrColIndA,
int blockDim,
bsrilu02Info_t info,
cusparseSolvePolicy_t policy,
void* pBuffer)
cusparseStatus_t
cusparseZbsrilu02(cusparseHandle_t handle,
cusparseDirection_t dirA,
int mb,
int nnzb,
const cusparseMatDescr_t descry,
cuDoubleComplex* bsrValA,
const int* bsrRowPtrA,
const int* bsrColIndA,
int blockDim,
bsrilu02Info_t info,
cusparseSolvePolicy_t policy,
void* pBuffer)
This function performs the solve phase of the incomplete-LU factorization with 0 fill-in and no pivoting.
\(A \approx LU\) |
A
is an (mb*blockDim)×(mb*blockDim)
sparse matrix that is defined in BSR storage format by the three arrays bsrValA
, bsrRowPtrA
, and bsrColIndA
. The block in BSR format is of size blockDim*blockDim
, stored as column-major or row-major determined by parameter dirA
, which is either CUSPARSE_DIRECTION_COLUMN
or CUSPARSE_DIRECTION_ROW
. The matrix type must be CUSPARSE_MATRIX_TYPE_GENERAL
, and the fill mode and diagonal type are ignored. Function bsrilu02()
supports an arbitrary blockDim
.
This function requires a buffer size returned by bsrilu02_bufferSize()
. The address of pBuffer
must be a multiple of 128 bytes. If it is not, CUSPARSE_STATUS_INVALID_VALUE
is returned.
Although bsrilu02()
can be used without level information, the user must be aware of consistency. If bsrilu02_analysis()
is called with policy CUSPARSE_SOLVE_POLICY_USE_LEVEL
, bsrilu02()
can be run with or without levels. On the other hand, if bsrilu02_analysis()
is called with CUSPARSE_SOLVE_POLICY_NO_LEVEL
, bsrilu02()
can only accept CUSPARSE_SOLVE_POLICY_NO_LEVEL
; otherwise, CUSPARSE_STATUS_INVALID_VALUE
is returned.
Function bsrilu02()
has the same behavior as csrilu02()
. That is, bsr2csr(bsrilu02(A)) = csrilu02(bsr2csr(A))
. The numerical zero of csrilu02()
means there exists some zero U(j,j)
. The numerical zero of bsrilu02()
means there exists some block U(j,j)
that is not invertible.
Function bsrilu02
reports the first numerical zero, including a structural zero. The user must call cusparseXbsrilu02_zeroPivot()
to know where the numerical zero is.
For example, suppose A
is a real m-by-m matrix where m=mb*blockDim
. The following code solves precondition system M*y = x
, where M
is the product of LU factors L
and U
.
// Suppose that A is m x m sparse matrix represented by BSR format,
// The number of block rows/columns is mb, and
// the number of nonzero blocks is nnzb.
// Assumption:
// - handle is already created by cusparseCreate(),
// - (d_bsrRowPtr, d_bsrColInd, d_bsrVal) is BSR of A on device memory,
// - d_x is right hand side vector on device memory.
// - d_y is solution vector on device memory.
// - d_z is intermediate result on device memory.
// - d_x, d_y and d_z are of size m.
cusparseMatDescr_t descr_M = 0;
cusparseMatDescr_t descr_L = 0;
cusparseMatDescr_t descr_U = 0;
bsrilu02Info_t info_M = 0;
bsrsv2Info_t info_L = 0;
bsrsv2Info_t info_U = 0;
int pBufferSize_M;
int pBufferSize_L;
int pBufferSize_U;
int pBufferSize;
void *pBuffer = 0;
int structural_zero;
int numerical_zero;
const double alpha = 1.;
const cusparseSolvePolicy_t policy_M = CUSPARSE_SOLVE_POLICY_NO_LEVEL;
const cusparseSolvePolicy_t policy_L = CUSPARSE_SOLVE_POLICY_NO_LEVEL;
const cusparseSolvePolicy_t policy_U = CUSPARSE_SOLVE_POLICY_USE_LEVEL;
const cusparseOperation_t trans_L = CUSPARSE_OPERATION_NON_TRANSPOSE;
const cusparseOperation_t trans_U = CUSPARSE_OPERATION_NON_TRANSPOSE;
const cusparseDirection_t dir = CUSPARSE_DIRECTION_COLUMN;
// step 1: create a descriptor which contains
// - matrix M is base-1
// - matrix L is base-1
// - matrix L is lower triangular
// - matrix L has unit diagonal
// - matrix U is base-1
// - matrix U is upper triangular
// - matrix U has non-unit diagonal
cusparseCreateMatDescr(&descr_M);
cusparseSetMatIndexBase(descr_M, CUSPARSE_INDEX_BASE_ONE);
cusparseSetMatType(descr_M, CUSPARSE_MATRIX_TYPE_GENERAL);
cusparseCreateMatDescr(&descr_L);
cusparseSetMatIndexBase(descr_L, CUSPARSE_INDEX_BASE_ONE);
cusparseSetMatType(descr_L, CUSPARSE_MATRIX_TYPE_GENERAL);
cusparseSetMatFillMode(descr_L, CUSPARSE_FILL_MODE_LOWER);
cusparseSetMatDiagType(descr_L, CUSPARSE_DIAG_TYPE_UNIT);
cusparseCreateMatDescr(&descr_U);
cusparseSetMatIndexBase(descr_U, CUSPARSE_INDEX_BASE_ONE);
cusparseSetMatType(descr_U, CUSPARSE_MATRIX_TYPE_GENERAL);
cusparseSetMatFillMode(descr_U, CUSPARSE_FILL_MODE_UPPER);
cusparseSetMatDiagType(descr_U, CUSPARSE_DIAG_TYPE_NON_UNIT);
// step 2: create a empty info structure
// we need one info for bsrilu02 and two info's for bsrsv2
cusparseCreateBsrilu02Info(&info_M);
cusparseCreateBsrsv2Info(&info_L);
cusparseCreateBsrsv2Info(&info_U);
// step 3: query how much memory used in bsrilu02 and bsrsv2, and allocate the buffer
cusparseDbsrilu02_bufferSize(handle, dir, mb, nnzb,
descr_M, d_bsrVal, d_bsrRowPtr, d_bsrColInd, blockDim, info_M, &pBufferSize_M);
cusparseDbsrsv2_bufferSize(handle, dir, trans_L, mb, nnzb,
descr_L, d_bsrVal, d_bsrRowPtr, d_bsrColInd, blockDim, info_L, &pBufferSize_L);
cusparseDbsrsv2_bufferSize(handle, dir, trans_U, mb, nnzb,
descr_U, d_bsrVal, d_bsrRowPtr, d_bsrColInd, blockDim, info_U, &pBufferSize_U);
pBufferSize = max(pBufferSize_M, max(pBufferSize_L, pBufferSize_U));
// pBuffer returned by cudaMalloc is automatically aligned to 128 bytes.
cudaMalloc((void**)&pBuffer, pBufferSize);
// step 4: perform analysis of incomplete LU factorization on M
// perform analysis of triangular solve on L
// perform analysis of triangular solve on U
// The lower(upper) triangular part of M has the same sparsity pattern as L(U),
// we can do analysis of bsrilu0 and bsrsv2 simultaneously.
cusparseDbsrilu02_analysis(handle, dir, mb, nnzb, descr_M,
d_bsrVal, d_bsrRowPtr, d_bsrColInd, blockDim, info_M,
policy_M, pBuffer);
status = cusparseXbsrilu02_zeroPivot(handle, info_M, &structural_zero);
if (CUSPARSE_STATUS_ZERO_PIVOT == statuss){
printf("A(%d,%d) is missing\n", structural_zero, structural_zero);
}
cusparseDbsrsv2_analysis(handle, dir, trans_L, mb, nnzb, descr_L,
d_bsrVal, d_bsrRowPtr, d_bsrColInd, blockDim,
info_L, policy_L, pBuffer);
cusparseDbsrsv2_analysis(handle, dir, trans_U, mb, nnzb, descr_U,
d_bsrVal, d_bsrRowPtr, d_bsrColInd, blockDim,
info_U, policy_U, pBuffer);
// step 5: M = L * U
cusparseDbsrilu02(handle, dir, mb, nnzb, descr_M,
d_bsrVal, d_bsrRowPtr, d_bsrColInd, blockDim, info_M, policy_M, pBuffer);
status = cusparseXbsrilu02_zeroPivot(handle, info_M, &numerical_zero);
if (CUSPARSE_STATUS_ZERO_PIVOT == statuss){
printf("block U(%d,%d) is not invertible\n", numerical_zero, numerical_zero);
}
// step 6: solve L*z = x
cusparseDbsrsv2_solve(handle, dir, trans_L, mb, nnzb, &alpha, descr_L,
d_bsrVal, d_bsrRowPtr, d_bsrColInd, blockDim, info_L,
d_x, d_z, policy_L, pBuffer);
// step 7: solve U*y = z
cusparseDbsrsv2_solve(handle, dir, trans_U, mb, nnzb, &alpha, descr_U,
d_bsrVal, d_bsrRowPtr, d_bsrColInd, blockDim, info_U,
d_z, d_y, policy_U, pBuffer);
// step 6: free resources
cudaFree(pBuffer);
cusparseDestroyMatDescr(descr_M);
cusparseDestroyMatDescr(descr_L);
cusparseDestroyMatDescr(descr_U);
cusparseDestroyBsrilu02Info(info_M);
cusparseDestroyBsrsv2Info(info_L);
cusparseDestroyBsrsv2Info(info_U);
cusparseDestroy(handle);
The function supports the following properties if pBuffer != NULL
The routine requires no extra storage
The routine supports asynchronous execution
The routine supports CUDA graph capture
Input
|
handle to the cuSPARSE library context. |
|
storage format of blocks: either |
|
number of block rows and block columns of matrix |
|
number of nonzero blocks of matrix |
|
the descriptor of matrix |
|
<type> array of |
|
integer array of |
|
integer array of |
|
block dimension of sparse matrix A; must be larger than zero. |
|
structure with information collected during the analysis phase (that should have been passed to the solve phase unchanged). |
|
the supported policies are |
|
buffer allocated by the user; the size is returned by |
Output
|
<type> matrix containing the incomplete-LU lower and upper triangular factors |
See cusparseStatus_t for the description of the return status.
11.2.10. cusparseXbsrilu02_zeroPivot()
cusparseStatus_t
cusparseXbsrilu02_zeroPivot(cusparseHandle_t handle,
bsrilu02Info_t info,
int* position)
If the returned error code is CUSPARSE_STATUS_ZERO_PIVOT
, position=j
means A(j,j)
has either a structural zero or a numerical zero (the block is not invertible). Otherwise position=-1
.
The position
can be 0-based or 1-based, the same as the matrix.
Function cusparseXbsrilu02_zeroPivot()
is a blocking call. It calls cudaDeviceSynchronize()
to make sure all previous kernels are done.
The position
can be in the host memory or device memory. The user can set proper the mode with cusparseSetPointerMode()
.
The routine requires no extra storage
The routine supports asynchronous execution if the Stream Ordered Memory Allocator is available
The routine supports CUDA graph capture if the Stream Ordered Memory Allocator is available
Input
|
handle to the cuSPARSE library context. |
|
|
Output
|
if no structural or numerical zero, |
See cusparseStatus_t for the description of the return status.
11.3. Tridiagonal Solve
Different algorithms for tridiagonal solve are discussed in this section.
11.3.1. cusparse<t>gtsv2_buffSizeExt()
cusparseStatus_t
cusparseSgtsv2_bufferSizeExt(cusparseHandle_t handle,
int m,
int n,
const float* dl,
const float* d,
const float* du,
const float* B,
int ldb,
size_t* bufferSizeInBytes)
cusparseStatus_t
cusparseDgtsv2_bufferSizeExt(cusparseHandle_t handle,
int m,
int n,
const double* dl,
const double* d,
const double* du,
const double* B,
int ldb,
size_t* bufferSizeInBytes)
cusparseStatus_t
cusparseCgtsv2_bufferSizeExt(cusparseHandle_t handle,
int m,
int n,
const cuComplex* dl,
const cuComplex* d,
const cuComplex* du,
const cuComplex* B,
int ldb,
size_t* bufferSizeInBytes)
cusparseStatus_t
cusparseZgtsv2_bufferSizeExt(cusparseHandle_t handle,
int m,
int n,
const cuDoubleComplex* dl,
const cuDoubleComplex* d,
const cuDoubleComplex* du,
const cuDoubleComplex* B,
int ldb,
size_t* bufferSizeInBytes)
This function returns the size of the buffer used in gtsv2
which computes the solution of a tridiagonal linear system with multiple right-hand sides.
\(A \ast X = B\) |
The coefficient matrix A
of each of these tri-diagonal linear system is defined with three vectors corresponding to its lower (dl
), main (d
), and upper (du
) matrix diagonals; the right-hand sides are stored in the dense matrix B
. Notice that solution X
overwrites right-hand-side matrix B
on exit.
The routine requires no extra storage
The routine supports asynchronous execution
The routine supports CUDA graph capture
Input
|
handle to the cuSPARSE library context. |
|
the size of the linear system (must be ≥ 3). |
|
number of right-hand sides, columns of matrix |
|
<type> dense array containing the lower diagonal of the tri-diagonal linear system. The first element of each lower diagonal must be zero. |
|
<type> dense array containing the main diagonal of the tri-diagonal linear system. |
|
<type> dense array containing the upper diagonal of the tri-diagonal linear system. The last element of each upper diagonal must be zero. |
|
<type> dense right-hand-side array of dimensions |
|
leading dimension of |
Output
|
number of bytes of the buffer used in the |
See cusparseStatus_t for the description of the return status.
11.3.2. cusparse<t>gtsv2()
cusparseStatus_t
cusparseSgtsv2(cusparseHandle_t handle,
int m,
int n,
const float* dl,
const float* d,
const float* du,
float* B,
int ldb,
void pBuffer)
cusparseStatus_t
cusparseDgtsv2(cusparseHandle_t handle,
int m,
int n,
const double* dl,
const double* d,
const double* du,
double* B,
int ldb,
void pBuffer)
cusparseStatus_t
cusparseCgtsv2(cusparseHandle_t handle,
int m,
int n,
const cuComplex* dl,
const cuComplex* d,
const cuComplex* du,
cuComplex* B,
int ldb,
void pBuffer)
cusparseStatus_t
cusparseZgtsv2(cusparseHandle_t handle,
int m,
int n,
const cuDoubleComplex* dl,
const cuDoubleComplex* d,
const cuDoubleComplex* du,
cuDoubleComplex* B,
int ldb,
void pBuffer)
This function computes the solution of a tridiagonal linear system with multiple right-hand sides:
\(A \ast X = B\) |
The coefficient matrix A
of each of these tri-diagonal linear system is defined with three vectors corresponding to its lower (dl
), main (d
), and upper (du
) matrix diagonals; the right-hand sides are stored in the dense matrix B
. Notice that solution X
overwrites right-hand-side matrix B
on exit.
Assuming A
is of size m
and base-1, dl
, d
and du
are defined by the following formula:
dl(i) := A(i, i-1)
for i=1,2,...,m
The first element of dl is out-of-bound (dl(1) := A(1,0)
), so dl(1) = 0
.
d(i) = A(i,i)
for i=1,2,...,m
du(i) = A(i,i+1)
for i=1,2,...,m
The last element of du is out-of-bound (du(m) := A(m,m+1)
), so du(m) = 0
.
The routine does perform pivoting, which usually results in more accurate and more stable results than cusparse<t>gtsv_nopivot()
or cusparse<t>gtsv2_nopivot()
at the expense of some execution time.
This function requires a buffer size returned by gtsv2_bufferSizeExt()
. The address of pBuffer
must be multiple of 128 bytes. If it is not, CUSPARSE_STATUS_INVALID_VALUE
is returned.
The routine requires no extra storage
The routine supports asynchronous execution
The routine supports CUDA graph capture
Input
|
handle to the cuSPARSE library context. |
|
the size of the linear system (must be ≥ 3). |
|
number of right-hand sides, columns of matrix |
|
<type> dense array containing the lower diagonal of the tri-diagonal linear system. The first element of each lower diagonal must be zero. |
|
<type> dense array containing the main diagonal of the tri-diagonal linear system. |
|
<type> dense array containing the upper diagonal of the tri-diagonal linear system. The last element of each upper diagonal must be zero. |
|
<type> dense right-hand-side array of dimensions |
|
leading dimension of |
|
buffer allocated by the user, the size is return by |
Output
|
<type> dense solution array of dimensions |
See cusparseStatus_t for the description of the return status.
11.3.3. cusparse<t>gtsv2_nopivot_bufferSizeExt()
cusparseStatus_t
cusparseSgtsv2_nopivot_bufferSizeExt(cusparseHandle_t handle,
int m,
int n,
const float* dl,
const float* d,
const float* du,
const float* B,
int ldb,
size_t* bufferSizeInBytes)
cusparseStatus_t
cusparseDgtsv2_nopivot_bufferSizeExt(cusparseHandle_t handle,
int m,
int n,
const double* dl,
const double* d,
const double* du,
const double* B,
int ldb,
size_t* bufferSizeInBytes)
cusparseStatus_t
cusparseCgtsv2_nopivot_bufferSizeExt(cusparseHandle_t handle,
int m,
int n,
const cuComplex* dl,
const cuComplex* d,
const cuComplex* du,
const cuComplex* B,
int ldb,
size_t* bufferSizeInBytes)
cusparseStatus_t
cusparseZgtsv2_nopivot_bufferSizeExt(cusparseHandle_t handle,
int m,
int n,
const cuDoubleComplex* dl,
const cuDoubleComplex* d,
const cuDoubleComplex* du,
const cuDoubleComplex* B,
int ldb,
size_t* bufferSizeInBytes)
This function returns the size of the buffer used in gtsv2_nopivot
which computes the solution of a tridiagonal linear system with multiple right-hand sides.
\(A \ast X = B\) |
The coefficient matrix A
of each of these tri-diagonal linear system is defined with three vectors corresponding to its lower (dl
), main (d
), and upper (du
) matrix diagonals; the right-hand sides are stored in the dense matrix B
. Notice that solution X
overwrites right-hand-side matrix B
on exit.
The routine requires no extra storage
The routine supports asynchronous execution
The routine supports CUDA graph capture
Input
|
handle to the cuSPARSE library context. |
|
the size of the linear system (must be ≥ 3). |
|
number of right-hand sides, columns of matrix |
|
<type> dense array containing the lower diagonal of the tri-diagonal linear system. The first element of each lower diagonal must be zero. |
|
<type> dense array containing the main diagonal of the tri-diagonal linear system. |
|
<type> dense array containing the upper diagonal of the tri-diagonal linear system. The last element of each upper diagonal must be zero. |
|
<type> dense right-hand-side array of dimensions |
|
leading dimension of |
Output
|
number of bytes of the buffer used in the |
See cusparseStatus_t for the description of the return status.
11.3.4. cusparse<t>gtsv2_nopivot()
cusparseStatus_t
cusparseSgtsv2_nopivot(cusparseHandle_t handle,
int m,
int n,
const float* dl,
const float* d,
const float* du,
float* B,
int ldb,
void* pBuffer)
cusparseStatus_t
cusparseDgtsv2_nopivot(cusparseHandle_t handle,
int m,
int n,
const double* dl,
const double* d,
const double* du,
double* B,
int ldb,
void* pBuffer)
cusparseStatus_t
cusparseCgtsv2_nopivot(cusparseHandle_t handle,
int m,
int n,
const cuComplex* dl,
const cuComplex* d,
const cuComplex* du,
cuComplex* B,
int ldb,
void* pBuffer)
cusparseStatus_t
cusparseZgtsv2_nopivot(cusparseHandle_t handle,
int m,
int n,
const cuDoubleComplex* dl,
const cuDoubleComplex* d,
const cuDoubleComplex* du,
cuDoubleComplex* B,
int ldb,
void* pBuffer)
This function computes the solution of a tridiagonal linear system with multiple right-hand sides:
\(A \ast X = B\) |
The coefficient matrix A
of each of these tri-diagonal linear system is defined with three vectors corresponding to its lower (dl
), main (d
), and upper (du
) matrix diagonals; the right-hand sides are stored in the dense matrix B
. Notice that solution X
overwrites right-hand-side matrix B
on exit.
The routine does not perform any pivoting and uses a combination of the Cyclic Reduction (CR) and the Parallel Cyclic Reduction (PCR) algorithms to find the solution. It achieves better performance when m
is a power of 2.
This function requires a buffer size returned by gtsv2_nopivot_bufferSizeExt()
. The address of pBuffer
must be multiple of 128 bytes. If it is not, CUSPARSE_STATUS_INVALID_VALUE
is returned.
The routine requires no extra storage
The routine supports asynchronous execution
The routine supports CUDA graph capture
Input
|
handle to the cuSPARSE library context. |
|
the size of the linear system (must be ≥ 3). |
|
number of right-hand sides, columns of matrix |
|
<type> dense array containing the lower diagonal of the tri-diagonal linear system. The first element of each lower diagonal must be zero. |
|
<type> dense array containing the main diagonal of the tri-diagonal linear system. |
|
<type> dense array containing the upper diagonal of the tri-diagonal linear system. The last element of each upper diagonal must be zero. |
|
<type> dense right-hand-side array of dimensions |
|
leading dimension of |
|
buffer allocated by the user, the size is return by |
Output
|
<type> dense solution array of dimensions |
See cusparseStatus_t for the description of the return status.
11.4. Batched Tridiagonal Solve
Different algorithms for batched tridiagonal solve are discussed in this section.
11.4.1. cusparse<t>gtsv2StridedBatch_bufferSizeExt()
cusparseStatus_t
cusparseSgtsv2StridedBatch_bufferSizeExt(cusparseHandle_t handle,
int m,
const float* dl,
const float* d,
const float* du,
const float* x,
int batchCount,
int batchStride,
size_t* bufferSizeInBytes)
cusparseStatus_t
cusparseDgtsv2StridedBatch_bufferSizeExt(cusparseHandle_t handle,
int m,
const double* dl,
const double* d,
const double* du,
const double* x,
int batchCount,
int batchStride,
size_t* bufferSizeInBytes)
cusparseStatus_t
cusparseCgtsv2StridedBatch_bufferSizeExt(cusparseHandle_t handle,
int m,
const cuComplex* dl,
const cuComplex* d,
const cuComplex* du,
const cuComplex* x,
int batchCount,
int batchStride,
size_t* bufferSizeInBytes)
cusparseStatus_t
cusparseZgtsv2StridedBatch_bufferSizeExt(cusparseHandle_t handle,
int m,
const cuDoubleComplex* dl,
const cuDoubleComplex* d,
const cuDoubleComplex* du,
const cuDoubleComplex* x,
int batchCount,
int batchStride,
size_t* bufferSizeInBytes)
This function returns the size of the buffer used in gtsv2StridedBatch
which computes the solution of multiple tridiagonal linear systems for i=0,…,batchCount
:
\(A^{(i)} \ast \text{y}^{(i)} = \text{x}^{(i)}\) |
The coefficient matrix A
of each of these tri-diagonal linear system is defined with three vectors corresponding to its lower (dl
), main (d
), and upper (du
) matrix diagonals; the right-hand sides are stored in the dense matrix X
. Notice that solution Y
overwrites right-hand-side matrix X
on exit. The different matrices are assumed to be of the same size and are stored with a fixed batchStride
in memory.
The routine requires no extra storage
The routine supports asynchronous execution
The routine supports CUDA graph capture
Input
|
handle to the cuSPARSE library context. |
|
the size of the linear system (must be ≥ 3). |
|
<type> dense array containing the lower diagonal of the tri-diagonal linear system. The lower diagonal \(dl^{(i)}\) that corresponds to the ith linear system starts at location |
|
<type> dense array containing the main diagonal of the tri-diagonal linear system. The main diagonal \(d^{(i)}\) that corresponds to the ith linear system starts at location |
|
<type> dense array containing the upper diagonal of the tri-diagonal linear system. The upper diagonal \(du^{(i)}\) that corresponds to the ith linear system starts at location |
|
<type> dense array that contains the right-hand-side of the tri-diagonal linear system. The right-hand-side \(x^{(i)}\) that corresponds to the ith linear system starts at location |
|
number of systems to solve. |
|
stride (number of elements) that separates the vectors of every system (must be at least |
Output
|
number of bytes of the buffer used in the |
See cusparseStatus_t for the description of the return status.
11.4.2. cusparse<t>gtsv2StridedBatch()
cusparseStatus_t
cusparseSgtsv2StridedBatch(cusparseHandle_t handle,
int m,
const float* dl,
const float* d,
const float* du,
float* x,
int batchCount,
int batchStride,
void* pBuffer)
cusparseStatus_t
cusparseDgtsv2StridedBatch(cusparseHandle_t handle,
int m,
const double* dl,
const double* d,
const double* du,
double* x,
int batchCount,
int batchStride,
void* pBuffer)
cusparseStatus_t
cusparseCgtsv2StridedBatch(cusparseHandle_t handle,
int m,
const cuComplex* dl,
const cuComplex* d,
const cuComplex* du,
cuComplex* x,
int batchCount,
int batchStride,
void* pBuffer)
cusparseStatus_t
cusparseZgtsv2StridedBatch(cusparseHandle_t handle,
int m,
const cuDoubleComplex* dl,
const cuDoubleComplex* d,
const cuDoubleComplex* du,
cuDoubleComplex* x,
int batchCount,
int batchStride,
void* pBuffer)
This function computes the solution of multiple tridiagonal linear systems for i=0,…,batchCount
:
\(A^{(i)} \ast \text{y}^{(i)} = \text{x}^{(i)}\) |
The coefficient matrix A
of each of these tri-diagonal linear system is defined with three vectors corresponding to its lower (dl
), main (d
), and upper (du
) matrix diagonals; the right-hand sides are stored in the dense matrix X
. Notice that solution Y
overwrites right-hand-side matrix X
on exit. The different matrices are assumed to be of the same size and are stored with a fixed batchStride
in memory.
The routine does not perform any pivoting and uses a combination of the Cyclic Reduction (CR) and the Parallel Cyclic Reduction (PCR) algorithms to find the solution. It achieves better performance when m
is a power of 2.
This function requires a buffer size returned by gtsv2StridedBatch_bufferSizeExt()
. The address of pBuffer
must be multiple of 128 bytes. If it is not, CUSPARSE_STATUS_INVALID_VALUE
is returned.
The routine requires no extra storage
The routine supports asynchronous execution
The routine supports CUDA graph capture
Input
|
handle to the cuSPARSE library context. |
|
the size of the linear system (must be ≥ 3). |
|
<type> dense array containing the lower diagonal of the tri-diagonal linear system. The lower diagonal \(dl^{(i)}\) that corresponds to the ith linear system starts at location |
|
<type> dense array containing the main diagonal of the tri-diagonal linear system. The main diagonal \(d^{(i)}\) that corresponds to the ith linear system starts at location |
|
<type> dense array containing the upper diagonal of the tri-diagonal linear system. The upper diagonal \(du^{(i)}\) that corresponds to the ith linear system starts at location |
|
<type> dense array that contains the right-hand-side of the tri-diagonal linear system. The right-hand-side \(x^{(i)}\) that corresponds to the ith linear system starts at location |
|
number of systems to solve. |
|
stride (number of elements) that separates the vectors of every system (must be at least |
|
buffer allocated by the user, the size is return by |
Output
|
<type> dense array that contains the solution of the tri-diagonal linear system. The solution \(x^{(i)}\) that corresponds to the ith linear system starts at location |
See cusparseStatus_t for the description of the return status.
11.4.3. cusparse<t>gtsvInterleavedBatch()
cusparseStatus_t
cusparseSgtsvInterleavedBatch_bufferSizeExt(cusparseHandle_t handle,
int algo,
int m,
const float* dl,
const float* d,
const float* du,
const float* x,
int batchCount,
size_t* pBufferSizeInBytes)
cusparseStatus_t
cusparseDgtsvInterleavedBatch_bufferSizeExt(cusparseHandle_t handle,
int algo,
int m,
const double* dl,
const double* d,
const double* du,
const double* x,
int batchCount,
size_t* pBufferSizeInBytes)
cusparseStatus_t
cusparseCgtsvInterleavedBatch_bufferSizeExt(cusparseHandle_t handle,
int algo,
int m,
const cuComplex* dl,
const cuComplex* d,
const cuComplex* du,
const cuComplex* x,
int batchCount,
size_t* pBufferSizeInBytes)
cusparseStatus_t
cusparseZgtsvInterleavedBatch_bufferSizeExt(cusparseHandle_t handle,
int algo,
int m,
const cuDoubleComplex* dl,
const cuDoubleComplex* d,
const cuDoubleComplex* du,
const cuDoubleComplex* x,
int batchCount,
size_t* pBufferSizeInBytes)
cusparseStatus_t
cusparseSgtsvInterleavedBatch(cusparseHandle_t handle,
int algo,
int m,
float* dl,
float* d,
float* du,
float* x,
int batchCount,
void* pBuffer)
cusparseStatus_t
cusparseDgtsvInterleavedBatch(cusparseHandle_t handle,
int algo,
int m,
double* dl,
double* d,
double* du,
double* x,
int batchCount,
void* pBuffer)
cusparseStatus_t
cusparseCgtsvInterleavedBatch(cusparseHandle_t handle,
int algo,
int m,
cuComplex* dl,
cuComplex* d,
cuComplex* du,
cuComplex* x,
int batchCount,
void* pBuffer)
cusparseStatus_t
cusparseZgtsvInterleavedBatch(cusparseHandle_t handle,
int algo,
int m,
cuDoubleComplex* dl,
cuDoubleComplex* d,
cuDoubleComplex* du,
cuDoubleComplex* x,
int batchCount,
void* pBuffer)
This function computes the solution of multiple tridiagonal linear systems for i=0,…,batchCount
:
\(A^{(i)} \ast \text{x}^{(i)} = \text{b}^{(i)}\) |
The coefficient matrix A
of each of these tri-diagonal linear system is defined with three vectors corresponding to its lower (dl
), main (d
), and upper (du
) matrix diagonals; the right-hand sides are stored in the dense matrix B
. Notice that solution X
overwrites right-hand-side matrix B
on exit.
Assuming A
is of size m
and base-1, dl
, d
and du
are defined by the following formula:
dl(i) := A(i, i-1)
for i=1,2,...,m
The first element of dl is out-of-bound (dl(1) := A(1,0)
), so dl(1) = 0
.
d(i) = A(i,i)
for i=1,2,...,m
du(i) = A(i,i+1)
for i=1,2,...,m
The last element of du is out-of-bound (du(m) := A(m,m+1)
), so du(m) = 0
.
The data layout is different from gtsvStridedBatch
which aggregates all matrices one after another. Instead, gtsvInterleavedBatch
gathers different matrices of the same element in a continous manner. If dl
is regarded as a 2-D array of size m-by-batchCount
, dl(:,j)
to store j-th
matrix. gtsvStridedBatch
uses column-major while gtsvInterleavedBatch
uses row-major.
The routine provides three different algorithms, selected by parameter algo
. The first algorithm is cuThomas
provided by Barcelona Supercomputing Center
. The second algorithm is LU with partial pivoting and last algorithm is QR. From stability perspective, cuThomas is not numerically stable because it does not have pivoting. LU with partial pivoting and QR are stable. From performance perspective, LU with partial pivoting and QR is about 10% to 20% slower than cuThomas.
This function requires a buffer size returned by gtsvInterleavedBatch_bufferSizeExt()
. The address of pBuffer
must be multiple of 128 bytes. If it is not, CUSPARSE_STATUS_INVALID_VALUE
is returned.
If the user prepares aggregate format, one can use cublasXgeam
to get interleaved format. However such transformation takes time comparable to solver itself. To reach best performance, the user must prepare interleaved format explicitly.
This function requires temporary extra storage that is allocated internally
The routine supports asynchronous execution if the Stream Ordered Memory Allocator is available
The routine supports CUDA graph capture if the Stream Ordered Memory Allocator is available
Input
|
handle to the cuSPARSE library context. |
|
algo = 0: cuThomas (unstable algorithm); algo = 1: LU with pivoting (stable algorithm); algo = 2: QR (stable algorithm) |
|
the size of the linear system. |
|
<type> dense array containing the lower diagonal of the tri-diagonal linear system. The first element of each lower diagonal must be zero. |
|
<type> dense array containing the main diagonal of the tri-diagonal linear system. |
|
<type> dense array containing the upper diagonal of the tri-diagonal linear system. The last element of each upper diagonal must be zero. |
|
<type> dense right-hand-side array of dimensions |
|
buffer allocated by the user, the size is return by |
Output
|
<type> dense solution array of dimensions |
See cusparseStatus_t for the description of the return status.
11.5. Batched Pentadiagonal Solve
Different algorithms for batched pentadiagonal solve are discussed in this section.
11.5.1. cusparse<t>gpsvInterleavedBatch()
cusparseStatus_t
cusparseSgpsvInterleavedBatch_bufferSizeExt(cusparseHandle_t handle,
int algo,
int m,
const float* ds,
const float* dl,
const float* d,
const float* du,
const float* dw,
const float* x,
int batchCount,
size_t* pBufferSizeInBytes)
cusparseStatus_t
cusparseDgpsvInterleavedBatch_bufferSizeExt(cusparseHandle_t handle,
int algo,
int m,
const double* ds,
const double* dl,
const double* d,
const double* du,
const double* dw,
const double* x,
int batchCount,
size_t* pBufferSizeInBytes)
cusparseStatus_t
cusparseCgpsvInterleavedBatch_bufferSizeExt(cusparseHandle_t handle,
int algo,
int m,
const cuComplex* ds,
const cuComplex* dl,
const cuComplex* d,
const cuComplex* du,
const cuComplex* dw,
const cuComplex* x,
int batchCount,
size_t* pBufferSizeInBytes)
cusparseStatus_t
cusparseZgpsvInterleavedBatch_bufferSizeExt(cusparseHandle_t handle,
int algo,
int m,
const cuDoubleComplex* ds,
const cuDoubleComplex* dl,
const cuDoubleComplex* d,
const cuDoubleComplex* du,
const cuDoubleComplex* dw,
const cuDoubleComplex* x,
int batchCount,
size_t* pBufferSizeInBytes)
cusparseStatus_t
cusparseSgpsvInterleavedBatch(cusparseHandle_t handle,
int algo,
int m,
float* ds,
float* dl,
float* d,
float* du,
float* dw,
float* x,
int batchCount,
void* pBuffer)
cusparseStatus_t
cusparseDgpsvInterleavedBatch(cusparseHandle_t handle,
int algo,
int m,
double* ds,
double* dl,
double* d,
double* du,
double* dw,
double* x,
int batchCount,
void* pBuffer)
cusparseStatus_t
cusparseCgpsvInterleavedBatch(cusparseHandle_t handle,
int algo,
int m,
cuComplex* ds,
cuComplex* dl,
cuComplex* d,
cuComplex* du,
cuComplex* dw,
cuComplex* x,
int batchCount,
void* pBuffer)
cusparseStatus_t
cusparseZgpsvInterleavedBatch(cusparseHandle_t handle,
int algo,
int m,
cuDoubleComplex* ds,
cuDoubleComplex* dl,
cuDoubleComplex* d,
cuDoubleComplex* du,
cuDoubleComplex* dw,
cuDoubleComplex* x,
int batchCount,
void* pBuffer)
This function computes the solution of multiple penta-diagonal linear systems for i=0,…,batchCount
:
\(A^{(i)} \ast \text{x}^{(i)} = \text{b}^{(i)}\) |
The coefficient matrix A
of each of these penta-diagonal linear system is defined with five vectors corresponding to its lower (ds, dl
), main (d
), and upper (du, dw
) matrix diagonals; the right-hand sides are stored in the dense matrix B
. Notice that solution X
overwrites right-hand-side matrix B
on exit.
Assuming A
is of size m
and base-1, ds
, dl
, d
, du
and dw
are defined by the following formula:
ds(i) := A(i, i-2)
for i=1,2,...,m
The first two elements of ds is out-of-bound (ds(1) := A(1,-1)
, ds(2) := A(2,0)
), so ds(1) = 0
and ds(2) = 0
.
dl(i) := A(i, i-1)
for i=1,2,...,m
The first element of dl is out-of-bound (dl(1) := A(1,0)
), so dl(1) = 0
.
d(i) = A(i,i)
for i=1,2,...,m
du(i) = A(i,i+1)
for i=1,2,...,m
The last element of du is out-of-bound (du(m) := A(m,m+1)
), so du(m) = 0
.
dw(i) = A(i,i+2)
for i=1,2,...,m
The last two elements of dw is out-of-bound (dw(m-1) := A(m-1,m+1)
, dw(m) := A(m,m+2)
), so dw(m-1) = 0
and dw(m) = 0
.
The data layout is the same as gtsvStridedBatch
.
The routine is numerically stable because it uses QR to solve the linear system.
This function requires a buffer size returned by gpsvInterleavedBatch_bufferSizeExt()
. The address of pBuffer
must be multiple of 128 bytes. If it is not, CUSPARSE_STATUS_INVALID_VALUE
is returned.
The function supports the following properties if pBuffer != NULL
The routine requires no extra storage
The routine supports asynchronous execution
The routine supports CUDA graph capture
Input
|
handle to the cuSPARSE library context. |
|
only support algo = 0 (QR) |
|
the size of the linear system. |
|
<type> dense array containing the lower diagonal (distance 2 to the diagonal) of the penta-diagonal linear system. The first two elements must be zero. |
|
<type> dense array containing the lower diagonal (distance 1 to the diagonal) of the penta-diagonal linear system. The first element must be zero. |
|
<type> dense array containing the main diagonal of the penta-diagonal linear system. |
|
<type> dense array containing the upper diagonal (distance 1 to the diagonal) of the penta-diagonal linear system. The last element must be zero. |
|
<type> dense array containing the upper diagonal (distance 2 to the diagonal) of the penta-diagonal linear system. The last two elements must be zero. |
|
<type> dense right-hand-side array of dimensions |
|
buffer allocated by the user, the size is return by |
Output
|
<type> dense solution array of dimensions |
See cusparseStatus_t for the description of the return status.
Please visit cuSPARSE Library Samples - cusparseSgpsvInterleavedBatch for a code example.
12. cuSPARSE Reorderings Reference
This chapter describes the reordering routines used to manipulate sparse matrices.
12.1. cusparse<t>csrcolor()
cusparseStatus_t
cusparseScsrcolor(cusparseHandle_t handle,
int m,
int nnz,
const cusparseMatDescr_t descrA,
const float* csrValA,
const int* csrRowPtrA,
const int* csrColIndA,
const float* fractionToColor,
int* ncolors,
int* coloring,
int* reordering,
cusparseColorInfo_t info)
cusparseStatus_t
cusparseDcsrcolor(cusparseHandle_t handle,
int m,
int nnz,
const cusparseMatDescr_t descrA,
const double* csrValA,
const int* csrRowPtrA,
const int* csrColIndA,
const double* fractionToColor,
int* ncolors,
int* coloring,
int* reordering,
cusparseColorInfo_t info)
cusparseStatus_t
cusparseCcsrcolor(cusparseHandle_t handle,
int m,
int nnz,
const cusparseMatDescr_t descrA,
const cuComplex* csrValA,
const int* csrRowPtrA,
const int* csrColIndA,
const cuComplex* fractionToColor,
int* ncolors,
int* coloring,
int* reordering,
cusparseColorInfo_t info)
cusparseStatus_t
cusparseZcsrcolor(cusparseHandle_t handle,
int m,
int nnz,
const cusparseMatDescr_t descrA,
const cuDoubleComplex* csrValA,
const int* csrRowPtrA,
const int* csrColIndA,
const cuDoubleComplex* fractionToColor,
int* ncolors,
int* coloring,
int* reordering,
cusparseColorInfo_t info)
This function performs the coloring of the adjacency graph associated with the matrix A stored in CSR format. The coloring is an assignment of colors (integer numbers) to nodes, such that neighboring nodes have distinct colors. An approximate coloring algorithm is used in this routine, and is stopped when a certain percentage of nodes has been colored. The rest of the nodes are assigned distinct colors (an increasing sequence of integers numbers, starting from the last integer used previously). The last two auxiliary routines can be used to extract the resulting number of colors, their assignment and the associated reordering. The reordering is such that nodes that have been assigned the same color are reordered to be next to each other.
The matrix A passed to this routine, must be stored as a general matrix and have a symmetric sparsity pattern. If the matrix is nonsymmetric the user should pass A+A^T as a parameter to this routine.
This function requires temporary extra storage that is allocated internally
The routine supports asynchronous execution if the Stream Ordered Memory Allocator is available
The routine supports CUDA graph capture if the Stream Ordered Memory Allocator is available
Input
|
handle to the cuSPARSE library context. |
|
number of rows of matrix |
|
number of nonzero elements of matrix |
|
the descriptor of matrix |
|
<type> array of |
|
integer array of |
|
integer array of |
|
fraction of nodes to be colored, which should be in the interval [0.0,1.0], for example 0.8 implies that 80 percent of nodes will be colored. |
|
structure with information to be passed to the coloring. |
Output
|
The number of distinct colors used (at most the size of the matrix, but likely much smaller). |
|
The resulting coloring permutation |
|
The resulting reordering permutation (untouched if NULL) |
See cusparseStatus_t for the description of the return status.
13. cuSPARSE Format Conversion Reference
This chapter describes the conversion routines between different sparse and dense storage formats.
coosort
, csrsort
, cscsort
, and csru2csr
are sorting routines without malloc inside, the following table estimates the buffer size.
|
|
|
|
|
125M |
|
|
100M |
|
|
71M for ‘d’ and 55M for ‘z’ |
13.1. cusparse<t>bsr2csr()
cusparseStatus_t
cusparseSbsr2csr(cusparseHandle_t handle,
cusparseDirection_t dir,
int mb,
int nb,
const cusparseMatDescr_t descrA,
const float* bsrValA,
const int* bsrRowPtrA,
const int* bsrColIndA,
int blockDim,
const cusparseMatDescr_t descrC,
float* csrValC,
int* csrRowPtrC,
int* csrColIndC)
cusparseStatus_t
cusparseDbsr2csr(cusparseHandle_t handle,
cusparseDirection_t dir,
int mb,
int nb,
const cusparseMatDescr_t descrA,
const double* bsrValA,
const int* bsrRowPtrA,
const int* bsrColIndA,
int blockDim,
const cusparseMatDescr_t descrC,
double* csrValC,
int* csrRowPtrC,
int* csrColIndC)
cusparseStatus_t
cusparseCbsr2csr(cusparseHandle_t handle,
cusparseDirection_t dir,
int mb,
int nb,
const cusparseMatDescr_t descrA,
const cuComplex* bsrValA,
const int* bsrRowPtrA,
const int* bsrColIndA,
int blockDim,
const cusparseMatDescr_t descrC,
cuComplex* csrValC,
int* csrRowPtrC,
int* csrColIndC)
cusparseStatus_t
cusparseZbsr2csr(cusparseHandle_t handle,
cusparseDirection_t dir,
int mb,
int nb,
const cusparseMatDescr_t descrA,
const cuDoubleComplex* bsrValA,
const int* bsrRowPtrA,
const int* bsrColIndA,
int blockDim,
const cusparseMatDescr_t descrC,
cuDoubleComplex* csrValC,
int* csrRowPtrC,
int* csrColIndC)
This function converts a sparse matrix in BSR format that is defined by the three arrays bsrValA
, bsrRowPtrA
, and bsrColIndA
) into a sparse matrix in CSR format that is defined by arrays csrValC
, csrRowPtrC
, and csrColIndC
.
Let m(=mb*blockDim)
be the number of rows of A
and n(=nb*blockDim)
be number of columns of A
, then A
and C
are m*n
sparse matrices. The BSR format of A
contains nnzb(=bsrRowPtrA[mb] - bsrRowPtrA[0])
nonzero blocks, whereas the sparse matrix A
contains nnz(=nnzb*blockDim*blockDim)
elements. The user must allocate enough space for arrays csrRowPtrC
, csrColIndC
, and csrValC
. The requirements are as follows:
csrRowPtrC
of m+1
elements
csrValC
of nnz
elements
csrColIndC
of nnz
elements
The general procedure is as follows:
// Given BSR format (bsrRowPtrA, bsrcolIndA, bsrValA) and
// blocks of BSR format are stored in column-major order.
cusparseDirection_t dir = CUSPARSE_DIRECTION_COLUMN;
int m = mb*blockDim;
int nnzb = bsrRowPtrA[mb] - bsrRowPtrA[0]; // number of blocks
int nnz = nnzb * blockDim * blockDim; // number of elements
cudaMalloc((void**)&csrRowPtrC, sizeof(int)*(m+1));
cudaMalloc((void**)&csrColIndC, sizeof(int)*nnz);
cudaMalloc((void**)&csrValC, sizeof(float)*nnz);
cusparseSbsr2csr(handle, dir, mb, nb,
descrA,
bsrValA, bsrRowPtrA, bsrColIndA,
blockDim,
descrC,
csrValC, csrRowPtrC, csrColIndC);
The routine requires no extra storage
The routine supports asynchronous execution if
blockDim != 1
or the Stream Ordered Memory Allocator is availableThe routine supports CUDA graph capture if
blockDim != 1
or the Stream Ordered Memory Allocator is available
Input
|
handle to the cuSPARSE library context. |
|
storage format of blocks, either |
|
number of block rows of sparse matrix |
|
number of block columns of sparse matrix |
|
the descriptor of matrix |
|
<type> array of |
|
integer array of |
|
integer array of |
|
block dimension of sparse matrix |
|
the descriptor of matrix |
Output
|
<type> array of |
|
integer array of |
|
integer array of |
See cusparseStatus_t for the description of the return status.
13.2. cusparse<t>gebsr2gebsc()
cusparseStatus_t
cusparseSgebsr2gebsc_bufferSize(cusparseHandle_t handle,
int mb,
int nb,
int nnzb,
const float* bsrVal,
const int* bsrRowPtr,
const int* bsrColInd,
int rowBlockDim,
int colBlockDim,
int* pBufferSize)
cusparseStatus_t
cusparseDgebsr2gebsc_bufferSize(cusparseHandle_t handle,
int mb,
int nb,
int nnzb,
const double* bsrVal,
const int* bsrRowPtr,
const int* bsrColInd,
int rowBlockDim,
int colBlockDim,
int* pBufferSize)
cusparseStatus_t
cusparseCgebsr2gebsc_bufferSize(cusparseHandle_t handle,
int mb,
int nb,
int nnzb,
const cuComplex* bsrVal,
const int* bsrRowPtr,
const int* bsrColInd,
int rowBlockDim,
int colBlockDim,
int* pBufferSize)
cusparseStatus_t
cusparseZgebsr2gebsc_bufferSize(cusparseHandle_t handle,
int mb,
int nb,
int nnzb,
const cuDoubleComplex* bsrVal,
const int* bsrRowPtr,
const int* bsrColInd,
int rowBlockDim,
int colBlockDim,
int* pBufferSize)
cusparseStatus_t
cusparseSgebsr2gebsc(cusparseHandle_t handle,
int mb,
int nb,
int nnzb,
const float* bsrVal,
const int* bsrRowPtr,
const int* bsrColInd,
int rowBlockDim,
int colBlockDim,
float* bscVal,
int* bscRowInd,
int* bscColPtr,
cusparseAction_t copyValues,
cusparseIndexBase_t baseIdx,
void* pBuffer)
cusparseStatus_t
cusparseDgebsr2gebsc(cusparseHandle_t handle,
int mb,
int nb,
int nnzb,
const double* bsrVal,
const int* bsrRowPtr,
const int* bsrColInd,
int rowBlockDim,
int colBlockDim,
double* bscVal,
int* bscRowInd,
int* bscColPtr,
cusparseAction_t copyValues,
cusparseIndexBase_t baseIdx,
void* pBuffer)
cusparseStatus_t
cusparseCgebsr2gebsc(cusparseHandle_t handle,
int mb,
int nb,
int nnzb,
const cuComplex* bsrVal,
const int* bsrRowPtr,
const int* bsrColInd,
int rowBlockDim,
int colBlockDim,
cuComplex* bscVal,
int* bscRowInd,
int* bscColPtr,
cusparseAction_t copyValues,
cusparseIndexBase_t baseIdx,
void* pBuffer)
cusparseStatus_t
cusparseZgebsr2gebsc(cusparseHandle_t handle,
int mb,
int nb,
int nnzb,
const cuDoubleComplex* bsrVal,
const int* bsrRowPtr,
const int* bsrColInd,
int rowBlockDim,
int colBlockDim,
cuDoubleComplex* bscVal,
int* bscRowInd,
int* bscColPtr,
cusparseAction_t copyValues,
cusparseIndexBase_t baseIdx,
void* pBuffer)
This function can be seen as the same as csr2csc()
when each block of size rowBlockDim*colBlockDim
is regarded as a scalar.
This sparsity pattern of the result matrix can also be seen as the transpose of the original sparse matrix, but the memory layout of a block does not change.
The user must call gebsr2gebsc_bufferSize()
to determine the size of the buffer required by gebsr2gebsc()
, allocate the buffer, and pass the buffer pointer to gebsr2gebsc()
.
The routine requires no extra storage if
pBuffer != NULL
The routine supports asynchronous execution if the Stream Ordered Memory Allocator is available
The routine supports CUDA graph capture if the Stream Ordered Memory Allocator is available
Input
|
handle to the cuSPARSE library context. |
|
number of block rows of sparse matrix |
|
number of block columns of sparse matrix |
|
number of nonzero blocks of matrix |
|
<type> array of |
|
integer array of |
|
integer array of |
|
number of rows within a block of |
|
number of columns within a block of |
|
|
|
|
|
host pointer containing number of bytes of the buffer used in |
|
buffer allocated by the user; the size is return by |
Output
|
<type> array of |
|
integer array of |
|
integer array of |
See cusparseStatus_t for the description of the return status.
13.3. cusparse<t>gebsr2gebsr()
cusparseStatus_t
cusparseSgebsr2gebsr_bufferSize(cusparseHandle_t handle,
cusparseDirection_t dir,
int mb,
int nb,
int nnzb,
const cusparseMatDescr_t descrA,
const float* bsrValA,
const int* bsrRowPtrA,
const int* bsrColIndA,
int rowBlockDimA,
int colBlockDimA,
int rowBlockDimC,
int colBlockDimC,
int* pBufferSize)
cusparseStatus_t
cusparseDgebsr2gebsr_bufferSize(cusparseHandle_t handle,
cusparseDirection_t dir,
int mb,
int nb,
int nnzb,
const cusparseMatDescr_t descrA,
const double* bsrValA,
const int* bsrRowPtrA,
const int* bsrColIndA,
int rowBlockDimA,
int colBlockDimA,
int rowBlockDimC,
int colBlockDimC,
int* pBufferSize)
cusparseStatus_t
cusparseCgebsr2gebsr_bufferSize(cusparseHandle_t handle,
cusparseDirection_t dir,
int mb,
int nb,
int nnzb,
const cusparseMatDescr_t descrA,
const cuComplex* bsrValA,
const int* bsrRowPtrA,
const int* bsrColIndA,
int rowBlockDimA,
int colBlockDimA,
int rowBlockDimC,
int colBlockDimC,
int* pBufferSize)
cusparseStatus_t
cusparseZgebsr2gebsr_bufferSize(cusparseHandle_t handle,
cusparseDirection_t dir,
int mb,
int nb,
int nnzb,
const cusparseMatDescr_t descrA,
const cuDoubleComplex* bsrValA,
const int* bsrRowPtrA,
const int* bsrColIndA,
int rowBlockDimA,
int colBlockDimA,
int rowBlockDimC,
int colBlockDimC,
int* pBufferSize)
cusparseStatus_t
cusparseXgebsr2gebsrNnz(cusparseHandle_t handle,
cusparseDirection_t dir,
int mb,
int nb,
int nnzb,
const cusparseMatDescr_t descrA,
const int* bsrRowPtrA,
const int* bsrColIndA,
int rowBlockDimA,
int colBlockDimA,
const cusparseMatDescr_t descrC,
int* bsrRowPtrC,
int rowBlockDimC,
int colBlockDimC,
int* nnzTotalDevHostPtr,
void* pBuffer)
cusparseStatus_t
cusparseSgebsr2gebsr(cusparseHandle_t handle,
cusparseDirection_t dir,
int mb,
int nb,
int nnzb,
const cusparseMatDescr_t descrA,
const float* bsrValA,
const int* bsrRowPtrA,
const int* bsrColIndA,
int rowBlockDimA,
int colBlockDimA,
const cusparseMatDescr_t descrC,
float* bsrValC,
int* bsrRowPtrC,
int* bsrColIndC,
int rowBlockDimC,
int colBlockDimC,
void* pBuffer)
cusparseStatus_t
cusparseDgebsr2gebsr(cusparseHandle_t handle,
cusparseDirection_t dir,
int mb,
int nb,
int nnzb,
const cusparseMatDescr_t descrA,
const double* bsrValA,
const int* bsrRowPtrA,
const int* bsrColIndA,
int rowBlockDimA,
int colBlockDimA,
const cusparseMatDescr_t descrC,
double* bsrValC,
int* bsrRowPtrC,
int* bsrColIndC,
int rowBlockDimC,
int colBlockDimC,
void* pBuffer)
cusparseStatus_t
cusparseCgebsr2gebsr(cusparseHandle_t handle,
cusparseDirection_t dir,
int mb,
int nb,
int nnzb,
const cusparseMatDescr_t descrA,
const cuComplex* bsrValA,
const int* bsrRowPtrA,
const int* bsrColIndA,
int rowBlockDimA,
int colBlockDimA,
const cusparseMatDescr_t descrC,
cuComplex* bsrValC,
int* bsrRowPtrC,
int* bsrColIndC,
int rowBlockDimC,
int colBlockDimC,
void* pBuffer)
cusparseStatus_t
cusparseZgebsr2gebsr(cusparseHandle_t handle,
cusparseDirection_t dir,
int mb,
int nb,
int nnzb,
const cusparseMatDescr_t descrA,
const cuDoubleComplex* bsrValA,
const int* bsrRowPtrA,
const int* bsrColIndA,
int rowBlockDimA,
int colBlockDimA,
const cusparseMatDescr_t descrC,
cuDoubleComplex* bsrValC,
int* bsrRowPtrC,
int* bsrColIndC,
int rowBlockDimC,
int colBlockDimC,
void* pBuffer)
This function converts a sparse matrix in general BSR format that is defined by the three arrays bsrValA
, bsrRowPtrA
, and bsrColIndA
into a sparse matrix in another general BSR format that is defined by arrays bsrValC
, bsrRowPtrC
, and bsrColIndC
.
If rowBlockDimA=1
and colBlockDimA=1
, cusparse[S|D|C|Z]gebsr2gebsr()
is the same as cusparse[S|D|C|Z]csr2gebsr()
.
If rowBlockDimC=1
and colBlockDimC=1
, cusparse[S|D|C|Z]gebsr2gebsr()
is the same as cusparse[S|D|C|Z]gebsr2csr()
.
A
is an m*n
sparse matrix where m(=mb*rowBlockDim)
is the number of rows of A
, and n(=nb*colBlockDim)
is the number of columns of A
. The general BSR format of A
contains nnzb(=bsrRowPtrA[mb] - bsrRowPtrA[0])
nonzero blocks. The matrix C
is also general BSR format with a different block size, rowBlockDimC*colBlockDimC
. If m
is not a multiple of rowBlockDimC
, or n
is not a multiple of colBlockDimC
, zeros are filled in. The number of block rows of C
is mc(=(m+rowBlockDimC-1)/rowBlockDimC)
. The number of block rows of C
is nc(=(n+colBlockDimC-1)/colBlockDimC)
. The number of nonzero blocks of C
is nnzc
.
The implementation adopts a two-step approach to do the conversion. First, the user allocates bsrRowPtrC
of mc+1
elements and uses function cusparseXgebsr2gebsrNnz()
to determine the number of nonzero block columns per block row of matrix C
. Second, the user gathers nnzc
(number of non-zero block columns of matrix C
) from either (nnzc=*nnzTotalDevHostPtr)
or (nnzc=bsrRowPtrC[mc]-bsrRowPtrC[0])
and allocates bsrValC
of nnzc*rowBlockDimC*colBlockDimC
elements and bsrColIndC
of nnzc
integers. Finally the function cusparse[S|D|C|Z]gebsr2gebsr()
is called to complete the conversion.
The user must call gebsr2gebsr_bufferSize()
to know the size of the buffer required by gebsr2gebsr()
, allocate the buffer, and pass the buffer pointer to gebsr2gebsr()
.
The general procedure is as follows:
// Given general BSR format (bsrRowPtrA, bsrColIndA, bsrValA) and
// blocks of BSR format are stored in column-major order.
cusparseDirection_t dir = CUSPARSE_DIRECTION_COLUMN;
int base, nnzc;
int m = mb*rowBlockDimA;
int n = nb*colBlockDimA;
int mc = (m+rowBlockDimC-1)/rowBlockDimC;
int nc = (n+colBlockDimC-1)/colBlockDimC;
int bufferSize;
void *pBuffer;
cusparseSgebsr2gebsr_bufferSize(handle, dir, mb, nb, nnzb,
descrA, bsrValA, bsrRowPtrA, bsrColIndA,
rowBlockDimA, colBlockDimA,
rowBlockDimC, colBlockDimC,
&bufferSize);
cudaMalloc((void**)&pBuffer, bufferSize);
cudaMalloc((void**)&bsrRowPtrC, sizeof(int)*(mc+1));
// nnzTotalDevHostPtr points to host memory
int *nnzTotalDevHostPtr = &nnzc;
cusparseXgebsr2gebsrNnz(handle, dir, mb, nb, nnzb,
descrA, bsrRowPtrA, bsrColIndA,
rowBlockDimA, colBlockDimA,
descrC, bsrRowPtrC,
rowBlockDimC, colBlockDimC,
nnzTotalDevHostPtr,
pBuffer);
if (NULL != nnzTotalDevHostPtr){
nnzc = *nnzTotalDevHostPtr;
}else{
cudaMemcpy(&nnzc, bsrRowPtrC+mc, sizeof(int), cudaMemcpyDeviceToHost);
cudaMemcpy(&base, bsrRowPtrC, sizeof(int), cudaMemcpyDeviceToHost);
nnzc -= base;
}
cudaMalloc((void**)&bsrColIndC, sizeof(int)*nnzc);
cudaMalloc((void**)&bsrValC, sizeof(float)*(rowBlockDimC*colBlockDimC)*nnzc);
cusparseSgebsr2gebsr(handle, dir, mb, nb, nnzb,
descrA, bsrValA, bsrRowPtrA, bsrColIndA,
rowBlockDimA, colBlockDimA,
descrC, bsrValC, bsrRowPtrC, bsrColIndC,
rowBlockDimC, colBlockDimC,
pBuffer);
The routines require no extra storage if
pBuffer != NULL
The routine supports asynchronous execution if the Stream Ordered Memory Allocator is available
The routines do not support CUDA graph capture
Input
|
handle to the cuSPARSE library context. |
|
storage format of blocks, either |
|
number of block rows of sparse matrix |
|
number of block columns of sparse matrix |
|
number of nonzero blocks of matrix |
|
the descriptor of matrix |
|
<type> array of |
|
integer array of |
|
integer array of |
|
number of rows within a block of |
|
number of columns within a block of |
|
the descriptor of matrix |
|
number of rows within a block of |
|
number of columns within a block of |
|
host pointer containing number of bytes of the buffer used in |
|
buffer allocated by the user; the size is return by |
Output
|
<type> array of |
|
integer array of |
|
integer array of |
|
total number of nonzero blocks of |
See cusparseStatus_t for the description of the return status.
13.4. cusparse<t>gebsr2csr()
cusparseStatus_t
cusparseSgebsr2csr(cusparseHandle_t handle,
cusparseDirection_t dir,
int mb,
int nb,
const cusparseMatDescr_t descrA,
const float* bsrValA,
const int* bsrRowPtrA,
const int* bsrColIndA,
int rowBlockDim,
int colBlockDim,
const cusparseMatDescr_t descrC,
float* csrValC,
int* csrRowPtrC,
int* csrColIndC)
cusparseStatus_t
cusparseDgebsr2csr(cusparseHandle_t handle,
cusparseDirection_t dir,
int mb,
int nb,
const cusparseMatDescr_t descrA,
const double* bsrValA,
const int* bsrRowPtrA,
const int* bsrColIndA,
int rowBlockDim,
int colBlockDim,
const cusparseMatDescr_t descrC,
double* csrValC,
int* csrRowPtrC,
int* csrColIndC)
cusparseStatus_t
cusparseCgebsr2csr(cusparseHandle_t handle,
cusparseDirection_t dir,
int mb,
int nb,
const cusparseMatDescr_t descrA,
const cuComplex* bsrValA,
const int* bsrRowPtrA,
const int* bsrColIndA,
int rowBlockDim,
int colBlockDim,
const cusparseMatDescr_t descrC,
cuComplex* csrValC,
int* csrRowPtrC,
int* csrColIndC)
cusparseStatus_t
cusparseZgebsr2csr(cusparseHandle_t handle,
cusparseDirection_t dir,
int mb,
int nb,
const cusparseMatDescr_t descrA,
const cuDoubleComplex* bsrValA,
const int* bsrRowPtrA,
const int* bsrColIndA,
int rowBlockDim,
int colBlockDim,
const cusparseMatDescr_t descrC,
cuDoubleComplex* csrValC,
int* csrRowPtrC,
int* csrColIndC)
This function converts a sparse matrix in general BSR format that is defined by the three arrays bsrValA
, bsrRowPtrA
, and bsrColIndA
into a sparse matrix in CSR format that is defined by arrays csrValC
, csrRowPtrC
, and csrColIndC
.
Let m(=mb*rowBlockDim)
be number of rows of A
and n(=nb*colBlockDim)
be number of columns of A
, then A
and C
are m*n
sparse matrices. The general BSR format of A
contains nnzb(=bsrRowPtrA[mb] - bsrRowPtrA[0])
non-zero blocks, whereas sparse matrix A
contains nnz(=nnzb*rowBlockDim*colBlockDim)
elements. The user must allocate enough space for arrays csrRowPtrC
, csrColIndC
, and csrValC
. The requirements are as follows:
csrRowPtrC
of m+1
elements
csrValC
of nnz
elements
csrColIndC
of nnz
elements
The general procedure is as follows:
// Given general BSR format (bsrRowPtrA, bsrColIndA, bsrValA) and
// blocks of BSR format are stored in column-major order.
cusparseDirection_t dir = CUSPARSE_DIRECTION_COLUMN;
int m = mb*rowBlockDim;
int n = nb*colBlockDim;
int nnzb = bsrRowPtrA[mb] - bsrRowPtrA[0]; // number of blocks
int nnz = nnzb * rowBlockDim * colBlockDim; // number of elements
cudaMalloc((void**)&csrRowPtrC, sizeof(int)*(m+1));
cudaMalloc((void**)&csrColIndC, sizeof(int)*nnz);
cudaMalloc((void**)&csrValC, sizeof(float)*nnz);
cusparseSgebsr2csr(handle, dir, mb, nb,
descrA,
bsrValA, bsrRowPtrA, bsrColIndA,
rowBlockDim, colBlockDim,
descrC,
csrValC, csrRowPtrC, csrColIndC);
The routine requires no extra storage
The routine supports asynchronous execution
The routine supports CUDA graph capture
Input
|
handle to the cuSPARSE library context. |
|
storage format of blocks, either |
|
number of block rows of sparse matrix |
|
number of block columns of sparse matrix |
|
the descriptor of matrix |
|
<type> array of |
|
integer array of |
|
integer array of |
|
number of rows within a block of |
|
number of columns within a block of |
|
the descriptor of matrix |
Output
|
<type> array of |
|
integer array of |
|
integer array of |
See cusparseStatus_t for the description of the return status.
13.5. cusparse<t>csr2gebsr()
cusparseStatus_t
cusparseScsr2gebsr_bufferSize(cusparseHandle_t handle,
cusparseDirection_t dir,
int m,
int n,
const cusparseMatDescr_t descrA,
const float* csrValA,
const int* csrRowPtrA,
const int* csrColIndA,
int rowBlockDim,
int colBlockDim,
int* pBufferSize)
cusparseStatus_t
cusparseDcsr2gebsr_bufferSize(cusparseHandle_t handle,
cusparseDirection_t dir,
int m,
int n,
const cusparseMatDescr_t descrA,
const double* csrValA,
const int* csrRowPtrA,
const int* csrColIndA,
int rowBlockDim,
int colBlockDim,
int* pBufferSize)
cusparseStatus_t
cusparseCcsr2gebsr_bufferSize(cusparseHandle_t handle,
cusparseDirection_t dir,
int m,
int n,
const cusparseMatDescr_t descrA,
const cuComplex* csrValA,
const int* csrRowPtrA,
const int* csrColIndA,
int rowBlockDim,
int colBlockDim,
int* pBufferSize)
cusparseStatus_t
cusparseZcsr2gebsr_bufferSize(cusparseHandle_t handle,
cusparseDirection_t dir,
int m,
int n,
const cusparseMatDescr_t descrA,
const cuDoubleComplex* csrValA,
const int* csrRowPtrA,
const int* csrColIndA,
int rowBlockDim,
int colBlockDim,
int* pBufferSize)
cusparseStatus_t
cusparseXcsr2gebsrNnz(cusparseHandle_t handle,
cusparseDirection_t dir,
int m,
int n,
const cusparseMatDescr_t descrA,
const int* csrRowPtrA,
const int* csrColIndA,
const cusparseMatDescr_t descrC,
int* bsrRowPtrC,
int rowBlockDim,
int colBlockDim,
int* nnzTotalDevHostPtr,
void* pBuffer)
cusparseStatus_t
cusparseScsr2gebsr(cusparseHandle_t handle,
cusparseDirection_t dir,
int m,
int n,
const cusparseMatDescr_t descrA,
const float* csrValA,
const int* csrRowPtrA,
const int* csrColIndA,
const cusparseMatDescr_t descrC,
float* bsrValC,
int* bsrRowPtrC,
int* bsrColIndC,
int rowBlockDim,
int colBlockDim,
void* pBuffer)
cusparseStatus_t
cusparseDcsr2gebsr(cusparseHandle_t handle,
cusparseDirection_t dir,
int m,
int n,
const cusparseMatDescr_t descrA,
const double* csrValA,
const int* csrRowPtrA,
const int* csrColIndA,
const cusparseMatDescr_t descrC,
double* bsrValC,
int* bsrRowPtrC,
int* bsrColIndC,
int rowBlockDim,
int colBlockDim,
void* pBuffer)
cusparseStatus_t
cusparseCcsr2gebsr(cusparseHandle_t handle,
cusparseDirection_t dir,
int m,
int n,
const cusparseMatDescr_t descrA,
const cuComplex* csrValA,
const int* csrRowPtrA,
const int* csrColIndA,
const cusparseMatDescr_t descrC,
cuComplex* bsrValC,
int* bsrRowPtrC,
int* bsrColIndC,
int rowBlockDim,
int colBlockDim,
void* pBuffer)
cusparseStatus_t
cusparseZcsr2gebsr(cusparseHandle_t handle,
cusparseDirection_t dir,
int m,
int n,
const cusparseMatDescr_t descrA,
const cuDoubleComplex* csrValA,
const int* csrRowPtrA,
const int* csrColIndA,
const cusparseMatDescr_t descrC,
cuDoubleComplex* bsrValC,
int* bsrRowPtrC,
int* bsrColIndC,
int rowBlockDim,
int colBlockDim,
void* pBuffer)
This function converts a sparse matrix A
in CSR format (that is defined by arrays csrValA
, csrRowPtrA
, and csrColIndA
) into a sparse matrix C
in general BSR format (that is defined by the three arrays bsrValC
, bsrRowPtrC
, and bsrColIndC
).
The matrix A is a m*n
sparse matrix and matrix C
is a (mb*rowBlockDim)*(nb*colBlockDim)
sparse matrix, where mb(=(m+rowBlockDim-1)/rowBlockDim)
is the number of block rows of C
, and nb(=(n+colBlockDim-1)/colBlockDim)
is the number of block columns of C
.
The block of C
is of size rowBlockDim*colBlockDim
. If m
is not multiple of rowBlockDim
or n
is not multiple of colBlockDim
, zeros are filled in.
The implementation adopts a two-step approach to do the conversion. First, the user allocates bsrRowPtrC
of mb+1
elements and uses function cusparseXcsr2gebsrNnz()
to determine the number of nonzero block columns per block row. Second, the user gathers nnzb
(number of nonzero block columns of matrix C
) from either (nnzb=*nnzTotalDevHostPtr)
or (nnzb=bsrRowPtrC[mb]-bsrRowPtrC[0])
and allocates bsrValC
of nnzb*rowBlockDim*colBlockDim
elements and bsrColIndC
of nnzb
integers. Finally function cusparse[S|D|C|Z]csr2gebsr()
is called to complete the conversion.
The user must obtain the size of the buffer required by csr2gebsr()
by calling csr2gebsr_bufferSize()
, allocate the buffer, and pass the buffer pointer to csr2gebsr()
.
The general procedure is as follows:
// Given CSR format (csrRowPtrA, csrColIndA, csrValA) and
// blocks of BSR format are stored in column-major order.
cusparseDirection_t dir = CUSPARSE_DIRECTION_COLUMN;
int base, nnzb;
int mb = (m + rowBlockDim-1)/rowBlockDim;
int nb = (n + colBlockDim-1)/colBlockDim;
int bufferSize;
void *pBuffer;
cusparseScsr2gebsr_bufferSize(handle, dir, m, n,
descrA, csrValA, csrRowPtrA, csrColIndA,
rowBlockDim, colBlockDim,
&bufferSize);
cudaMalloc((void**)&pBuffer, bufferSize);
cudaMalloc((void**)&bsrRowPtrC, sizeof(int) *(mb+1));
// nnzTotalDevHostPtr points to host memory
int *nnzTotalDevHostPtr = &nnzb;
cusparseXcsr2gebsrNnz(handle, dir, m, n,
descrA, csrRowPtrA, csrColIndA,
descrC, bsrRowPtrC, rowBlockDim, colBlockDim,
nnzTotalDevHostPtr,
pBuffer);
if (NULL != nnzTotalDevHostPtr){
nnzb = *nnzTotalDevHostPtr;
}else{
cudaMemcpy(&nnzb, bsrRowPtrC+mb, sizeof(int), cudaMemcpyDeviceToHost);
cudaMemcpy(&base, bsrRowPtrC, sizeof(int), cudaMemcpyDeviceToHost);
nnzb -= base;
}
cudaMalloc((void**)&bsrColIndC, sizeof(int)*nnzb);
cudaMalloc((void**)&bsrValC, sizeof(float)*(rowBlockDim*colBlockDim)*nnzb);
cusparseScsr2gebsr(handle, dir, m, n,
descrA,
csrValA, csrRowPtrA, csrColIndA,
descrC,
bsrValC, bsrRowPtrC, bsrColIndC,
rowBlockDim, colBlockDim,
pBuffer);
The routine cusparseXcsr2gebsrNnz()
has the following properties:
The routine requires no extra storage
The routine supports asynchronous execution if the Stream Ordered Memory Allocator is available
The routine supports CUDA graph capture if the Stream Ordered Memory Allocator is available
The routine cusparse<t>csr2gebsr()
has the following properties:
The routine requires no extra storage if
pBuffer != NULL
The routine supports asynchronous execution
The routine supports CUDA graph capture
Input
|
handle to the cuSPARSE library context. |
|
storage format of blocks, either |
|
number of rows of sparse matrix |
|
number of columns of sparse matrix |
|
the descriptor of matrix |
|
<type> array of |
|
integer array of |
|
integer array of |
|
the descriptor of matrix |
|
number of rows within a block of |
|
number of columns within a block of |
|
buffer allocated by the user, the size is return by |
Output
|
<type> array of |
|
integer array of |
|
integer array of |
|
total number of nonzero blocks of matrix |
See cusparseStatus_t for the description of the return status.
13.6. cusparse<t>coo2csr()
cusparseStatus_t
cusparseXcoo2csr(cusparseHandle_t handle,
const int* cooRowInd,
int nnz,
int m,
int* csrRowPtr,
cusparseIndexBase_t idxBase)
This function converts the array containing the uncompressed row indices (corresponding to COO format) into an array of compressed row pointers (corresponding to CSR format).
It can also be used to convert the array containing the uncompressed column indices (corresponding to COO format) into an array of column pointers (corresponding to CSC format).
The routine requires no extra storage
The routine supports asynchronous execution
The routine supports CUDA graph capture
Input
|
handle to the cuSPARSE library context. |
|
integer array of |
|
number of non-zeros of the sparse matrix (that is also the length of array |
|
number of rows of matrix |
|
|
Output
|
integer array of |
See cusparseStatus_t for the description of the return status.
13.7. cusparse<t>csr2coo()
cusparseStatus_t
cusparseXcsr2coo(cusparseHandle_t handle,
const int* csrRowPtr,
int nnz,
int m,
int* cooRowInd,
cusparseIndexBase_t idxBase)
This function converts the array containing the compressed row pointers (corresponding to CSR format) into an array of uncompressed row indices (corresponding to COO format).
It can also be used to convert the array containing the compressed column indices (corresponding to CSC format) into an array of uncompressed column indices (corresponding to COO format).
The routine requires no extra storage
The routine supports asynchronous execution
The routine supports CUDA graph capture
Input
|
handle to the cuSPARSE library context. |
|
integer array of |
|
number of nonzeros of the sparse matrix (that is also the length of array |
|
number of rows of matrix |
|
|
Output
|
integer array of |
See cusparseStatus_t for the description of the return status.
13.8. cusparseCsr2cscEx2()
cusparseStatus_t
cusparseCsr2cscEx2_bufferSize(cusparseHandle_t handle,
int m,
int n,
int nnz,
const void* csrVal,
const int* csrRowPtr,
const int* csrColInd,
void* cscVal,
int* cscColPtr,
int* cscRowInd,
cudaDataType valType,
cusparseAction_t copyValues,
cusparseIndexBase_t idxBase,
cusparseCsr2CscAlg_t alg,
size_t* bufferSize)
cusparseStatus_t
cusparseCsr2cscEx2(cusparseHandle_t handle,
int m,
int n,
int nnz,
const void* csrVal,
const int* csrRowPtr,
const int* csrColInd,
void* cscVal,
int* cscColPtr,
int* cscRowInd,
cudaDataType valType,
cusparseAction_t copyValues,
cusparseIndexBase_t idxBase,
cusparseCsr2CscAlg_t alg,
void* buffer)
This function converts a sparse matrix in CSR format (that is defined by the three arrays csrVal
, csrRowPtr
, and csrColInd
) into a sparse matrix in CSC format (that is defined by arrays cscVal
, cscRowInd
, and cscColPtr
). The resulting matrix can also be seen as the transpose of the original sparse matrix. Notice that this routine can also be used to convert a matrix in CSC format into a matrix in CSR format.
The routine requires extra storage proportional to the number of nonzero values nnz
. It provides in output always the same matrix.
It is executed asynchronously with respect to the host, and it may return control to the application on the host before the result is ready.
The function cusparseCsr2cscEx2_bufferSize()
returns the size of the workspace needed by cusparseCsr2cscEx2()
. User needs to allocate a buffer of this size and give that buffer to cusparseCsr2cscEx2()
as an argument.
If nnz == 0
, then csrColInd
, csrVal
, cscVal
, and cscRowInd
could have NULL
value. In this case, cscColPtr
is set to idxBase
for all values.
If m == 0
or n == 0
, the pointers are not checked and the routine returns CUSPARSE_STATUS_SUCCESS
.
Input
|
Handle to the cuSPARSE library context |
|
Number of rows of the CSR input matrix; number of columns of the CSC ouput matrix |
|
Number of columns of the CSR input matrix; number of rows of the CSC ouput matrix |
|
Number of nonzero elements of the CSR and CSC matrices |
|
Value array of size |
|
Integer array of size |
|
Integer array of size |
|
Value array of size |
|
Integer array of size |
|
Integer array of size |
|
Value type for both CSR and CSC matrices |
|
|
|
Index base |
|
Algorithm implementation. see |
|
Number of bytes of workspace needed by |
|
Pointer to workspace buffer |
cusparseCsr2cscEx2()
supports the following data types:
|
---|
|
|
|
|
|
|
|
|
|
The routine requires no extra storage
The routine supports asynchronous execution
The routine supports CUDA graph capture
See cusparseStatus_t for the description of the return status.
13.9. cusparse<t>nnz()
cusparseStatus_t
cusparseSnnz(cusparseHandle_t handle,
cusparseDirection_t dirA,
int m,
int n,
const cusparseMatDescr_t descrA,
const float* A,
int lda,
int* nnzPerRowColumn,
int* nnzTotalDevHostPtr)
cusparseStatus_t
cusparseDnnz(cusparseHandle_t handle,
cusparseDirection_t dirA,
int m,
int n,
const cusparseMatDescr_t descrA,
const double* A,
int lda,
int* nnzPerRowColumn,
int* nnzTotalDevHostPtr)
cusparseStatus_t
cusparseCnnz(cusparseHandle_t handle,
cusparseDirection_t dirA,
int m,
int n,
const cusparseMatDescr_t descrA,
const cuComplex* A,
int lda,
int* nnzPerRowColumn,
int* nnzTotalDevHostPtr)
cusparseStatus_t
cusparseZnnz(cusparseHandle_t handle,
cusparseDirection_t dirA,
int m,
int n,
const cusparseMatDescr_t descrA,
const cuDoubleComplex* A,
int lda,
int* nnzPerRowColumn,
int* nnzTotalDevHostPtr)
This function computes the number of nonzero elements per row or column and the total number of nonzero elements in a dense matrix.
This function requires temporary extra storage that is allocated internally
The routine supports asynchronous execution if the Stream Ordered Memory Allocator is available
The routine supports CUDA graph capture if the Stream Ordered Memory Allocator is available
Input
|
handle to the cuSPARSE library context. |
|
direction that specifies whether to count nonzero elements by |
|
number of rows of matrix |
|
number of columns of matrix |
|
the descriptor of matrix |
|
array of dimensions |
|
leading dimension of dense array |
Output
|
array of size |
|
total number of nonzero elements in device or host memory. |
See cusparseStatus_t for the description of the return status.
13.10. cusparseCreateIdentityPermutation()
cusparseStatus_t
cusparseCreateIdentityPermutation(cusparseHandle_t handle,
int n,
int* p);
This function creates an identity map. The output parameter p
represents such map by p = 0:1:(n-1)
.
This function is typically used with coosort
, csrsort
, cscsort
.
The routine requires no extra storage
The routine supports asynchronous execution
The routine supports CUDA graph capture
Input
|
|
|
|
|
handle to the cuSPARSE library context. |
|
|
size of the map. |
Output
|
|
|
|
|
integer array of dimensions |
See cusparseStatus_t for the description of the return status.
13.11. cusparseXcoosort()
cusparseStatus_t
cusparseXcoosort_bufferSizeExt(cusparseHandle_t handle,
int m,
int n,
int nnz,
const int* cooRows,
const int* cooCols,
size_t* pBufferSizeInBytes)
cusparseStatus_t
cusparseXcoosortByRow(cusparseHandle_t handle,
int m,
int n,
int nnz,
int* cooRows,
int* cooCols,
int* P,
void* pBuffer)
cusparseStatus_t
cusparseXcoosortByColumn(cusparseHandle_t handle,
int m,
int n,
int nnz,
int* cooRows,
int* cooCols,
int* P,
void* pBuffer);
This function sorts COO format. The sorting is in-place. Also the user can sort by row or sort by column.
A
is an m×n
sparse matrix that is defined in COO storage format by the three arrays cooVals
, cooRows
, and cooCols
.
There is no assumption for the base index of the matrix. coosort
uses stable sort on signed integer, so the value of cooRows
or cooCols
can be negative.
This function coosort()
requires buffer size returned by coosort_bufferSizeExt()
. The address of pBuffer
must be multiple of 128 bytes. If not, CUSPARSE_STATUS_INVALID_VALUE
is returned.
The parameter P
is both input and output. If the user wants to compute sorted cooVal
, P
must be set as 0:1:(nnz-1) before coosort()
, and after coosort()
, new sorted value array satisfies cooVal_sorted = cooVal(P)
.
Remark: the dimension m
and n
are not used. If the user does not know the value of m
or n
, just passes a value positive. This usually happens if the user only reads a COO array first and needs to decide the dimension m
or n
later.
The routine requires no extra storage if
pBuffer != NULL
The routine supports asynchronous execution if the Stream Ordered Memory Allocator is available
The routine supports CUDA graph capture if the Stream Ordered Memory Allocator is available
Input
|
|
|
|
|
handle to the cuSPARSE library context. |
|
|
number of rows of matrix |
|
|
number of columns of matrix |
|
|
number of nonzero elements of matrix |
|
|
integer array of |
|
|
integer array of |
|
|
integer array of |
|
|
buffer allocated by the user; the size is returned by |
Output
|
|
|
|
|
integer array of |
|
|
integer array of |
|
|
integer array of |
|
|
number of bytes of the buffer. |
See cusparseStatus_t for the description of the return status
Please visit cuSPARSE Library Samples - cusparseXcoosortByRow for a code example.
13.12. cusparseXcsrsort()
cusparseStatus_t
cusparseXcsrsort_bufferSizeExt(cusparseHandle_t handle,
int m,
int n,
int nnz,
const int* csrRowPtr,
const int* csrColInd,
size_t* pBufferSizeInBytes)
cusparseStatus_t
cusparseXcsrsort(cusparseHandle_t handle,
int m,
int n,
int nnz,
const cusparseMatDescr_t descrA,
const int* csrRowPtr,
int* csrColInd,
int* P,
void* pBuffer)
This function sorts CSR format. The stable sorting is in-place.
The matrix type is regarded as CUSPARSE_MATRIX_TYPE_GENERAL
implicitly. In other words, any symmetric property is ignored.
This function csrsort()
requires buffer size returned by csrsort_bufferSizeExt()
. The address of pBuffer
must be multiple of 128 bytes. If not, CUSPARSE_STATUS_INVALID_VALUE
is returned.
The parameter P
is both input and output. If the user wants to compute sorted csrVal
, P
must be set as 0:1:(nnz-1) before csrsort()
, and after csrsort()
, new sorted value array satisfies csrVal_sorted = csrVal(P)
.
The general procedure is as follows:
// A is a 3x3 sparse matrix, base-0
// | 1 2 3 |
// A = | 4 5 6 |
// | 7 8 9 |
const int m = 3;
const int n = 3;
const int nnz = 9;
csrRowPtr[m+1] = { 0, 3, 6, 9}; // on device
csrColInd[nnz] = { 2, 1, 0, 0, 2,1, 1, 2, 0}; // on device
csrVal[nnz] = { 3, 2, 1, 4, 6, 5, 8, 9, 7}; // on device
size_t pBufferSizeInBytes = 0;
void *pBuffer = NULL;
int *P = NULL;
// step 1: allocate buffer
cusparseXcsrsort_bufferSizeExt(handle, m, n, nnz, csrRowPtr, csrColInd, &pBufferSizeInBytes);
cudaMalloc( &pBuffer, sizeof(char)* pBufferSizeInBytes);
// step 2: setup permutation vector P to identity
cudaMalloc( (void**)&P, sizeof(int)*nnz);
cusparseCreateIdentityPermutation(handle, nnz, P);
// step 3: sort CSR format
cusparseXcsrsort(handle, m, n, nnz, descrA, csrRowPtr, csrColInd, P, pBuffer);
// step 4: gather sorted csrVal
cusparseDgthr(handle, nnz, csrVal, csrVal_sorted, P, CUSPARSE_INDEX_BASE_ZERO);
The routine requires no extra storage if
pBuffer != NULL
The routine supports asynchronous execution if the Stream Ordered Memory Allocator is available
The routine supports CUDA graph capture if the Stream Ordered Memory Allocator is available
Input
|
|
|
|
|
handle to the cuSPARSE library context. |
|
|
number of rows of matrix |
|
|
number of columns of matrix |
|
|
number of nonzero elements of matrix |
|
|
integer array of |
|
|
integer array of |
|
|
integer array of |
|
|
buffer allocated by the user; the size is returned by |
Output
|
|
|
|
|
integer array of |
|
|
integer array of |
|
|
number of bytes of the buffer. |
See cusparseStatus_t for the description of the return status.
13.13. cusparseXcscsort()
cusparseStatus_t
cusparseXcscsort_bufferSizeExt(cusparseHandle_t handle,
int m,
int n,
int nnz,
const int* cscColPtr,
const int* cscRowInd,
size_t* pBufferSizeInBytes)
cusparseStatus_t
cusparseXcscsort(cusparseHandle_t handle,
int m,
int n,
int nnz,
const cusparseMatDescr_t descrA,
const int* cscColPtr,
int* cscRowInd,
int* P,
void* pBuffer)
This function sorts CSC format. The stable sorting is in-place.
The matrix type is regarded as CUSPARSE_MATRIX_TYPE_GENERAL
implicitly. In other words, any symmetric property is ignored.
This function cscsort()
requires buffer size returned by cscsort_bufferSizeExt()
. The address of pBuffer
must be multiple of 128 bytes. If not, CUSPARSE_STATUS_INVALID_VALUE
is returned.
The parameter P
is both input and output. If the user wants to compute sorted cscVal
, P
must be set as 0:1:(nnz-1) before cscsort()
, and after cscsort()
, new sorted value array satisfies cscVal_sorted = cscVal(P)
.
The general procedure is as follows:
// A is a 3x3 sparse matrix, base-0
// | 1 2 |
// A = | 4 0 |
// | 0 8 |
const int m = 3;
const int n = 2;
const int nnz = 4;
cscColPtr[n+1] = { 0, 2, 4}; // on device
cscRowInd[nnz] = { 1, 0, 2, 0}; // on device
cscVal[nnz] = { 4.0, 1.0, 8.0, 2.0 }; // on device
size_t pBufferSizeInBytes = 0;
void *pBuffer = NULL;
int *P = NULL;
// step 1: allocate buffer
cusparseXcscsort_bufferSizeExt(handle, m, n, nnz, cscColPtr, cscRowInd, &pBufferSizeInBytes);
cudaMalloc( &pBuffer, sizeof(char)* pBufferSizeInBytes);
// step 2: setup permutation vector P to identity
cudaMalloc( (void**)&P, sizeof(int)*nnz);
cusparseCreateIdentityPermutation(handle, nnz, P);
// step 3: sort CSC format
cusparseXcscsort(handle, m, n, nnz, descrA, cscColPtr, cscRowInd, P, pBuffer);
// step 4: gather sorted cscVal
cusparseDgthr(handle, nnz, cscVal, cscVal_sorted, P, CUSPARSE_INDEX_BASE_ZERO);
The routine requires no extra storage if
pBuffer != NULL
The routine supports asynchronous execution if the Stream Ordered Memory Allocator is available
The routine supports CUDA graph capture if the Stream Ordered Memory Allocator is available
Input
|
|
|
|
|
handle to the cuSPARSE library context. |
|
|
number of rows of matrix |
|
|
number of columns of matrix |
|
|
number of nonzero elements of matrix |
|
|
integer array of |
|
|
integer array of |
|
|
integer array of |
|
|
buffer allocated by the user; the size is returned by |
Output
|
|
|
|
|
integer array of |
|
|
integer array of |
|
|
number of bytes of the buffer. |
See cusparseStatus_t for the description of the return status.
13.14. cusparseXcsru2csr()
cusparseStatus_t
cusparseCreateCsru2csrInfo(csru2csrInfo_t *info);
cusparseStatus_t
cusparseDestroyCsru2csrInfo(csru2csrInfo_t info);
cusparseStatus_t
cusparseScsru2csr_bufferSizeExt(cusparseHandle_t handle,
int m,
int n,
int nnz,
float* csrVal,
const int* csrRowPtr,
int* csrColInd,
csru2csrInfo_t info,
size_t* pBufferSizeInBytes)
cusparseStatus_t
cusparseDcsru2csr_bufferSizeExt(cusparseHandle_t handle,
int m,
int n,
int nnz,
double* csrVal,
const int* csrRowPtr,
int* csrColInd,
csru2csrInfo_t info,
size_t* pBufferSizeInBytes)
cusparseStatus_t
cusparseCcsru2csr_bufferSizeExt(cusparseHandle_t handle,
int m,
int n,
int nnz,
cuComplex* csrVal,
const int* csrRowPtr,
int* csrColInd,
csru2csrInfo_t info,
size_t* pBufferSizeInBytes)
cusparseStatus_t
cusparseZcsru2csr_bufferSizeExt(cusparseHandle_t handle,
int m,
int n,
int nnz,
cuDoubleComplex* csrVal,
const int* csrRowPtr,
int* csrColInd,
csru2csrInfo_t info,
size_t* pBufferSizeInBytes)
cusparseStatus_t
cusparseScsru2csr(cusparseHandle_t handle,
int m,
int n,
int nnz,
const cusparseMatDescr_t descrA,
float* csrVal,
const int* csrRowPtr,
int* csrColInd,
csru2csrInfo_t info,
void* pBuffer)
cusparseStatus_t
cusparseDcsru2csr(cusparseHandle_t handle,
int m,
int n,
int nnz,
const cusparseMatDescr_t descrA,
double* csrVal,
const int* csrRowPtr,
int* csrColInd,
csru2csrInfo_t info,
void* pBuffer)
cusparseStatus_t
cusparseCcsru2csr(cusparseHandle_t handle,
int m,
int n,
int nnz,
const cusparseMatDescr_t descrA,
cuComplex* csrVal,
const int* csrRowPtr,
int* csrColInd,
csru2csrInfo_t info,
void* pBuffer)
cusparseStatus_t
cusparseZcsru2csr(cusparseHandle_t handle,
int m,
int n,
int nnz,
const cusparseMatDescr_t descrA,
cuDoubleComplex* csrVal,
const int* csrRowPtr,
int* csrColInd,
csru2csrInfo_t info,
void* pBuffer)
cusparseStatus_t
cusparseScsr2csru(cusparseHandle_t handle,
int m,
int n,
int nnz,
const cusparseMatDescr_t descrA,
float* csrVal,
const int* csrRowPtr,
int* csrColInd,
csru2csrInfo_t info,
void* pBuffer)
cusparseStatus_t
cusparseDcsr2csru(cusparseHandle_t handle,
int m,
int n,
int nnz,
const cusparseMatDescr_t descrA,
double* csrVal,
const int* csrRowPtr,
int* csrColInd,
csru2csrInfo_t info,
void* pBuffer)
cusparseStatus_t
cusparseCcsr2csru(cusparseHandle_t handle,
int m,
int n,
int nnz,
const cusparseMatDescr_t descrA,
cuComplex* csrVal,
const int* csrRowPtr,
int* csrColInd,
csru2csrInfo_t info,
void* pBuffer)
cusparseStatus_t
cusparseZcsr2csru(cusparseHandle_t handle,
int m,
int n,
int nnz,
const cusparseMatDescr_t descrA,
cuDoubleComplex* csrVal,
const int* csrRowPtr,
int* csrColInd,
csru2csrInfo_t info,
void* pBuffer)
This function transfers unsorted CSR format to CSR format, and vice versa. The operation is in-place.
This function is a wrapper of csrsort
and gthr
. The usecase is the following scenario.
If the user has a matrix A
of CSR format which is unsorted, and implements his own code (which can be CPU or GPU kernel) based on this special order (for example, diagonal first, then lower triangle, then upper triangle), and wants to convert it to CSR format when calling CUSPARSE library, and then convert it back when doing something else on his/her kernel. For example, suppose the user wants to solve a linear system Ax=b
by the following iterative scheme
\(x^{(k+1)} = x^{(k)} + L^{(-1)}*(b - Ax^{(k)})\) |
The code heavily uses SpMv and triangular solve. Assume that the user has an in-house design of SpMV (Sparse Matrix-Vector multiplication) based on special order of A
. However the user wants to use CUSAPRSE library for triangular solver. Then the following code can work.
do step 1: compute residual vector r = b - A x (k) by in-house SpMV step 2: B := sort(A), and L is lower triangular part of B (only sort A once and keep the permutation vector) step 3: solve z = L (-1) * ( b - A x (k) ) by cusparseXcsrsv step 4: add correction x (k+1) = x (k) + z step 5: A := unsort(B) (use permutation vector to get back the unsorted CSR) until convergence |
The requirements of step 2 and step 5 are
In-place operation.
The permutation vector
P
is hidden in an opaque structure.No
cudaMalloc
inside the conversion routine. Instead, the user has to provide the buffer explicitly.The conversion between unsorted CSR and sorted CSR may needs several times, but the function only generates the permutation vector
P
once.The function is based on
csrsort
,gather
andscatter
operations.
The operation is called csru2csr
, which means unsorted CSR to sorted CSR. Also we provide the inverse operation, called csr2csru
.
In order to keep the permutation vector invisible, we need an opaque structure called csru2csrInfo
. Then two functions (cusparseCreateCsru2csrInfo
, cusparseDestroyCsru2csrInfo
) are used to initialize and to destroy the opaque structure.
cusparse[S|D|C|Z]csru2csr_bufferSizeExt
returns the size of the buffer. The permutation vector P
is also allcated inside csru2csrInfo
. The lifetime of the permutation vector is the same as the lifetime of csru2csrInfo
.
cusparse[S|D|C|Z]csru2csr
performs forward transformation from unsorted CSR to sorted CSR. First call uses csrsort to generate the permutation vector P
, and subsequent call uses P
to do transformation.
cusparse[S|D|C|Z]csr2csru
performs backward transformation from sorted CSR to unsorted CSR. P
is used to get unsorted form back.
The routine cusparse<t>csru2csr()
has the following properties:
The routine requires no extra storage if
pBuffer != NULL
The routine supports asynchronous execution if the Stream Ordered Memory Allocator is available
The routine supports CUDA graph capture if the Stream Ordered Memory Allocator is available
The routine cusparse<t>csr2csru()
has the following properties if pBuffer != NULL
:
The routine requires no extra storage
The routine supports asynchronous execution
The routine supports CUDA graph capture
The following tables describe parameters of csr2csru_bufferSizeExt
and csr2csru
.
Input
|
|
|
|
|
handle to the cuSPARSE library context. |
|
|
number of rows of matrix |
|
|
number of columns of matrix |
|
|
number of nonzero elements of matrix |
|
|
the descriptor of matrix |
|
|
<type> array of nnz unsorted nonzero elements of matrix |
|
|
integer array of |
|
|
integer array of |
|
|
opaque structure initialized using |
|
|
buffer allocated by the user; the size is returned by |
Output
|
|
|
|
|
<type> array of nnz sorted nonzero elements of matrix |
|
|
integer array of |
|
|
number of bytes of the buffer. |
See cusparseStatus_t for the description of the return status.
13.15. cusparseXpruneDense2csr()
cusparseStatus_t
cusparseHpruneDense2csr_bufferSizeExt(cusparseHandle_t handle,
int m,
int n,
const __half* A,
int lda,
const __half* threshold,
const cusparseMatDescr_t descrC,
const __half* csrValC,
const int* csrRowPtrC,
const int* csrColIndC,
size_t* pBufferSizeInBytes)
cusparseStatus_t
cusparseSpruneDense2csr_bufferSizeExt(cusparseHandle_t handle,
int m,
int n,
const float* A,
int lda,
const float* threshold,
const cusparseMatDescr_t descrC,
const float* csrValC,
const int* csrRowPtrC,
const int* csrColIndC,
size_t* pBufferSizeInBytes)
cusparseStatus_t
cusparseDpruneDense2csr_bufferSizeExt(cusparseHandle_t handle,
int m,
int n,
const double* A,
int lda,
const double* threshold,
const cusparseMatDescr_t descrC,
const double* csrValC,
const int* csrRowPtrC,
const int* csrColIndC,
size_t* pBufferSizeInBytes)
cusparseStatus_t
cusparseHpruneDense2csrNnz(cusparseHandle_t handle,
int m,
int n,
const __half* A,
int lda,
const __half* threshold,
const cusparseMatDescr_t descrC,
int* csrRowPtrC,
int* nnzTotalDevHostPtr,
void* pBuffer)
cusparseStatus_t
cusparseSpruneDense2csrNnz(cusparseHandle_t handle,
int m,
int n,
const float* A,
int lda,
const float* threshold,
const cusparseMatDescr_t descrC,
int* csrRowPtrC,
int* nnzTotalDevHostPtr,
void* pBuffer)
cusparseStatus_t
cusparseDpruneDense2csrNnz(cusparseHandle_t handle,
int m,
int n,
const double* A,
int lda,
const double* threshold,
const cusparseMatDescr_t descrC,
int* csrRowPtrC,
int* nnzTotalDevHostPtr,
void* pBuffer)
cusparseStatus_t
cusparseHpruneDense2csr(cusparseHandle_t handle,
int m,
int n,
const __half* A,
int lda,
const __half* threshold,
const cusparseMatDescr_t descrC,
__half* csrValC,
const int* csrRowPtrC,
int* csrColIndC,
void* pBuffer)
cusparseStatus_t
cusparseSpruneDense2csr(cusparseHandle_t handle,
int m,
int n,
const float* A,
int lda,
const float* threshold,
const cusparseMatDescr_t descrC,
float* csrValC,
const int* csrRowPtrC,
int* csrColIndC,
void* pBuffer)
cusparseStatus_t
cusparseDpruneDense2csr(cusparseHandle_t handle,
int m,
int n,
const double* A,
int lda,
const double* threshold,
const cusparseMatDescr_t descrC,
double* csrValC,
const int* csrRowPtrC,
int* csrColIndC,
void* pBuffer)
This function prunes a dense matrix to a sparse matrix with CSR format.
Given a dense matrix A
and a non-negative value threshold
, the function returns a sparse matrix C
, defined by
\(\begin{matrix} {{C(i,j)} = {A(i,j)}} & \text{if\ |A(i,j)|\ >\ threshold} \\ \end{matrix}\) |
The implementation adopts a two-step approach to do the conversion. First, the user allocates csrRowPtrC
of m+1
elements and uses function pruneDense2csrNnz()
to determine the number of nonzeros columns per row. Second, the user gathers nnzC
(number of nonzeros of matrix C
) from either (nnzC=*nnzTotalDevHostPtr)
or (nnzC=csrRowPtrC[m]-csrRowPtrC[0])
and allocates csrValC
of nnzC
elements and csrColIndC
of nnzC
integers. Finally function pruneDense2csr()
is called to complete the conversion.
The user must obtain the size of the buffer required by pruneDense2csr()
by calling pruneDense2csr_bufferSizeExt()
, allocate the buffer, and pass the buffer pointer to pruneDense2csr()
.
Examples of prune chapter provides a simple example of pruneDense2csr()
.
The routine cusparse<t>pruneDense2csrNnz()
has the following properties:
This function requires temporary extra storage that is allocated internally
The routine supports asynchronous execution if the Stream Ordered Memory Allocator is available
The routine supports CUDA graph capture if the Stream Ordered Memory Allocator is available
The routine cusparse<t>DpruneDense2csr()
has the following properties:
The routine requires no extra storage
The routine supports asynchronous execution
The routine supports CUDA graph capture
Input
|
|
|
|
|
handle to the cuSPARSE library context. |
|
|
number of rows of matrix |
|
|
number of columns of matrix |
|
|
array of dimension (lda, n). |
|
|
leading dimension of |
|
|
a value to drop the entries of A. |
|
|
the descriptor of matrix |
|
|
buffer allocated by the user; the size is returned by |
Output
|
|
|
|
|
total number of nonzero of matrix |
|
|
<type> array of |
|
|
integer array of |
|
|
integer array of |
|
|
number of bytes of the buffer. |
See cusparseStatus_t for the description of the return status.
13.16. cusparseXpruneCsr2csr()
cusparseStatus_t
cusparseHpruneCsr2csr_bufferSizeExt(cusparseHandle_t handle,
int m,
int n,
int nnzA,
const cusparseMatDescr_t descrA,
const __half* csrValA,
const int* csrRowPtrA,
const int* csrColIndA,
const __half* threshold,
const cusparseMatDescr_t descrC,
const __half* csrValC,
const int* csrRowPtrC,
const int* csrColIndC,
size_t* pBufferSizeInBytes)
cusparseStatus_t
cusparseSpruneCsr2csr_bufferSizeExt(cusparseHandle_t handle,
int m,
int n,
int nnzA,
const cusparseMatDescr_t descrA,
const float* csrValA,
const int* csrRowPtrA,
const int* csrColIndA,
const float* threshold,
const cusparseMatDescr_t descrC,
const float* csrValC,
const int* csrRowPtrC,
const int* csrColIndC,
size_t* pBufferSizeInBytes)
cusparseStatus_t
cusparseDpruneCsr2csr_bufferSizeExt(cusparseHandle_t handle,
int m,
int n,
int nnzA,
const cusparseMatDescr_t descrA,
const double* csrValA,
const int* csrRowPtrA,
const int* csrColIndA,
const double* threshold,
const cusparseMatDescr_t descrC,
const double* csrValC,
const int* csrRowPtrC,
const int* csrColIndC,
size_t* pBufferSizeInBytes)
cusparseStatus_t
cusparseHpruneCsr2csrNnz(cusparseHandle_t handle,
int m,
int n,
int nnzA,
const cusparseMatDescr_t descrA,
const __half* csrValA,
const int* csrRowPtrA,
const int* csrColIndA,
const __half* threshold,
const cusparseMatDescr_t descrC,
int* csrRowPtrC,
int* nnzTotalDevHostPtr,
void* pBuffer)
cusparseStatus_t
cusparseSpruneCsr2csrNnz(cusparseHandle_t handle,
int m,
int n,
int nnzA,
const cusparseMatDescr_t descrA,
const float* csrValA,
const int* csrRowPtrA,
const int* csrColIndA,
const float* threshold,
const cusparseMatDescr_t descrC,
int* csrRowPtrC,
int* nnzTotalDevHostPtr,
void* pBuffer)
cusparseStatus_t
cusparseDpruneCsr2csrNnz(cusparseHandle_t handle,
int m,
int n,
int nnzA,
const cusparseMatDescr_t descrA,
const double* csrValA,
const int* csrRowPtrA,
const int* csrColIndA,
const double* threshold,
const cusparseMatDescr_t descrC,
int* csrRowPtrC,
int* nnzTotalDevHostPtr,
void* pBuffer)
cusparseStatus_t
cusparseHpruneCsr2csr(cusparseHandle_t handle,
int m,
int n,
int nnzA,
const cusparseMatDescr_t descrA,
const __half* csrValA,
const int* csrRowPtrA,
const int* csrColIndA,
const __half* threshold,
const cusparseMatDescr_t descrC,
__half* csrValC,
const int* csrRowPtrC,
int* csrColIndC,
void* pBuffer)
cusparseStatus_t
cusparseSpruneCsr2csr(cusparseHandle_t handle,
int m,
int n,
int nnzA,
const cusparseMatDescr_t descrA,
const float* csrValA,
const int* csrRowPtrA,
const int* csrColIndA,
const float* threshold,
const cusparseMatDescr_t descrC,
float* csrValC,
const int* csrRowPtrC,
int* csrColIndC,
void* pBuffer)
cusparseStatus_t
cusparseDpruneCsr2csr(cusparseHandle_t handle,
int m,
int n,
int nnzA,
const cusparseMatDescr_t descrA,
const double* csrValA,
const int* csrRowPtrA,
const int* csrColIndA,
const double* threshold,
const cusparseMatDescr_t descrC,
double* csrValC,
const int* csrRowPtrC,
int* csrColIndC,
void* pBuffer)
This function prunes a sparse matrix to a sparse matrix with CSR format.
Given a sparse matrix A
and a non-negative value threshold
, the function returns a sparse matrix C
, defined by
\(\begin{matrix} {{C(i,j)} = {A(i,j)}} & \text{if\ |A(i,j)|\ >\ threshold} \\ \end{matrix}\) |
The implementation adopts a two-step approach to do the conversion. First, the user allocates csrRowPtrC
of m+1
elements and uses function pruneCsr2csrNnz()
to determine the number of nonzeros columns per row. Second, the user gathers nnzC
(number of nonzeros of matrix C
) from either (nnzC=*nnzTotalDevHostPtr)
or (nnzC=csrRowPtrC[m]-csrRowPtrC[0])
and allocates csrValC
of nnzC
elements and csrColIndC
of nnzC
integers. Finally function pruneCsr2csr()
is called to complete the conversion.
The user must obtain the size of the buffer required by pruneCsr2csr()
by calling pruneCsr2csr_bufferSizeExt()
, allocate the buffer, and pass the buffer pointer to pruneCsr2csr()
.
Examples of prune chapter provides a simple example of pruneCsr2csr()
.
The routine cusparse<t>pruneCsr2csrNnz()
has the following properties:
This function requires temporary extra storage that is allocated internally
The routine supports asynchronous execution if the Stream Ordered Memory Allocator is available
The routine supports CUDA graph capture if the Stream Ordered Memory Allocator is available
The routine cusparse<t>pruneCsr2csr()
has the following properties:
The routine requires no extra storage
The routine supports asynchronous execution
The routine supports CUDA graph capture
Input
|
|
|
|
|
handle to the cuSPARSE library context. |
|
|
number of rows of matrix |
|
|
number of columns of matrix |
|
|
number of nonzeros of matrix |
|
|
the descriptor of matrix |
|
|
<type> array of |
|
|
integer array of |
|
|
integer array of |
|
|
a value to drop the entries of A. |
|
|
the descriptor of matrix |
|
|
buffer allocated by the user; the size is returned by |
Output
|
|
|
|
|
total number of nonzero of matrix |
|
|
<type> array of |
|
|
integer array of |
|
|
integer array of |
|
|
number of bytes of the buffer. |
See cusparseStatus_t for the description of the return status.
13.17. cusparseXpruneDense2csrPercentage()
cusparseStatus_t
cusparseHpruneDense2csrByPercentage_bufferSizeExt(cusparseHandle_t handle,
int m,
int n,
const __half* A,
int lda,
float percentage,
const cusparseMatDescr_t descrC,
const __half* csrValC,
const int* csrRowPtrC,
const int* csrColIndC,
pruneInfo_t info,
size_t* pBufferSizeInBytes)
cusparseStatus_t
cusparseSpruneDense2csrByPercentage_bufferSizeExt(cusparseHandle_t handle,
int m,
int n,
const float* A,
int lda,
float percentage,
const cusparseMatDescr_t descrC,
const float* csrValC,
const int* csrRowPtrC,
const int* csrColIndC,
pruneInfo_t info,
size_t* pBufferSizeInBytes)
cusparseStatus_t
cusparseDpruneDense2csrByPercentage_bufferSizeExt(cusparseHandle_t handle,
int m,
int n,
const double* A,
int lda,
float percentage,
const cusparseMatDescr_t descrC,
const double* csrValC,
const int* csrRowPtrC,
const int* csrColIndC,
pruneInfo_t info,
size_t* pBufferSizeInBytes)
cusparseStatus_t
cusparseHpruneDense2csrNnzByPercentage(cusparseHandle_t handle,
int m,
int n,
const __half* A,
int lda,
float percentage,
const cusparseMatDescr_t descrC,
int* csrRowPtrC,
int* nnzTotalDevHostPtr,
pruneInfo_t info,
void* pBuffer)
cusparseStatus_t
cusparseSpruneDense2csrNnzByPercentage(cusparseHandle_t handle,
int m,
int n,
const float* A,
int lda,
float percentage,
const cusparseMatDescr_t descrC,
int* csrRowPtrC,
int* nnzTotalDevHostPtr,
pruneInfo_t info,
void* pBuffer)
cusparseStatus_t
cusparseDpruneDense2csrNnzByPercentage(cusparseHandle_t handle,
int m,
int n,
const double* A,
int lda,
float percentage,
const cusparseMatDescr_t descrC,
int* csrRowPtrC,
int* nnzTotalDevHostPtr,
pruneInfo_t info,
void* pBuffer)
cusparseStatus_t
cusparseHpruneDense2csrByPercentage(cusparseHandle_t handle,
int m,
int n,
const __half* A,
int lda,
float percentage,
const cusparseMatDescr_t descrC,
__half* csrValC,
const int* csrRowPtrC,
int* csrColIndC,
pruneInfo_t info,
void* pBuffer)
cusparseStatus_t
cusparseSpruneDense2csrByPercentage(cusparseHandle_t handle,
int m,
int n,
const float* A,
int lda,
float percentage,
const cusparseMatDescr_t descrC,
float* csrValC,
const int* csrRowPtrC,
int* csrColIndC,
pruneInfo_t info,
void* pBuffer)
cusparseStatus_t
cusparseDpruneDense2csrByPercentage(cusparseHandle_t handle,
int m,
int n,
const double* A,
int lda,
float percentage,
const cusparseMatDescr_t descrC,
double* csrValC,
const int* csrRowPtrC,
int* csrColIndC,
pruneInfo_t info,
void* pBuffer)
This function prunes a dense matrix to a sparse matrix by percentage.
Given a dense matrix A
and a non-negative value percentage
, the function computes sparse matrix C
by the following three steps:
Step 1: sort absolute value of A
in ascending order.
\(\begin{matrix} {key\ :=\ sort(\ |A|\ )} \\ \end{matrix}\) |
Step 2: choose threshold by the parameter percentage
\(\begin{matrix} {pos\ =\ ceil(m*n*(percentage/100))\ -\ 1} \\ {pos\ =\ min(pos,\ m*n-1)} \\ {pos\ =\ max(pos,\ 0)} \\ {threshold\ =\ key\lbrack pos\rbrack} \\ \end{matrix}\) |
Step 3: call pruneDense2csr()
by with the parameter threshold
.
The implementation adopts a two-step approach to do the conversion. First, the user allocates csrRowPtrC
of m+1
elements and uses function pruneDense2csrNnzByPercentage()
to determine the number of nonzeros columns per row. Second, the user gathers nnzC
(number of nonzeros of matrix C
) from either (nnzC=*nnzTotalDevHostPtr)
or (nnzC=csrRowPtrC[m]-csrRowPtrC[0])
and allocates csrValC
of nnzC
elements and csrColIndC
of nnzC
integers. Finally function pruneDense2csrByPercentage()
is called to complete the conversion.
The user must obtain the size of the buffer required by pruneDense2csrByPercentage()
by calling pruneDense2csrByPercentage_bufferSizeExt()
, allocate the buffer, and pass the buffer pointer to pruneDense2csrByPercentage()
.
Remark 1: the value of percentage
must be not greater than 100. Otherwise, CUSPARSE_STATUS_INVALID_VALUE
is returned.
Remark 2: the zeros of A
are not ignored. All entries are sorted, including zeros. This is different from pruneCsr2csrByPercentage()
Examples of prune chapter provides a simple example of pruneDense2csrNnzByPercentage()
.
The routine cusparse<t>pruneDense2csrNnzByPercentage()
has the following properties:
This function requires temporary extra storage that is allocated internally
The routine supports asynchronous execution if the Stream Ordered Memory Allocator is available
The routine supports CUDA graph capture if the Stream Ordered Memory Allocator is available
The routine cusparse<t>pruneDense2csrByPercentage()
has the following properties:
The routine requires no extra storage
The routine supports asynchronous execution
The routine supports CUDA graph capture
Input
|
|
|
|
|
handle to the cuSPARSE library context. |
|
|
number of rows of matrix |
|
|
number of columns of matrix |
|
|
array of dimension (lda, n). |
|
|
leading dimension of |
|
|
percentage <=100 and percentage >= 0 |
|
|
the descriptor of matrix |
|
|
buffer allocated by the user; the size is returned by |
Output
|
|
|
|
|
total number of nonzero of matrix |
|
|
<type> array of |
|
|
integer array of |
|
|
integer array of |
|
|
number of bytes of the buffer. |
See cusparseStatus_t for the description of the return status.
13.18. cusparseXpruneCsr2csrByPercentage()
cusparseStatus_t
cusparseHpruneCsr2csrByPercentage_bufferSizeExt(cusparseHandle_t handle,
int m,
int n,
int nnzA,
const cusparseMatDescr_t descrA,
const __half* csrValA,
const int* csrRowPtrA,
const int* csrColIndA,
float percentage,
const cusparseMatDescr_t descrC,
const __half* csrValC,
const int* csrRowPtrC,
const int* csrColIndC,
pruneInfo_t info,
size_t* pBufferSizeInBytes)
cusparseStatus_t
cusparseSpruneCsr2csrByPercentage_bufferSizeExt(cusparseHandle_t handle,
int m,
int n,
int nnzA,
const cusparseMatDescr_t descrA,
const float* csrValA,
const int* csrRowPtrA,
const int* csrColIndA,
float percentage,
const cusparseMatDescr_t descrC,
const float* csrValC,
const int* csrRowPtrC,
const int* csrColIndC,
pruneInfo_t info,
size_t* pBufferSizeInBytes)
cusparseStatus_t
cusparseDpruneCsr2csrByPercentage_bufferSizeExt(cusparseHandle_t handle,
int m,
int n,
int nnzA,
const cusparseMatDescr_t descrA,
const double* csrValA,
const int* csrRowPtrA,
const int* csrColIndA,
float percentage,
const cusparseMatDescr_t descrC,
const double* csrValC,
const int* csrRowPtrC,
const int* csrColIndC,
pruneInfo_t info,
size_t* pBufferSizeInBytes)
cusparseStatus_t
cusparseHpruneCsr2csrNnzByPercentage(cusparseHandle_t handle,
int m,
int n,
int nnzA,
const cusparseMatDescr_t descrA,
const __half* csrValA,
const int* csrRowPtrA,
const int* csrColIndA,
float percentage,
const cusparseMatDescr_t descrC,
int* csrRowPtrC,
int* nnzTotalDevHostPtr,
pruneInfo_t info,
void* pBuffer)
cusparseStatus_t
cusparseSpruneCsr2csrNnzByPercentage(cusparseHandle_t handle,
int m,
int n,
int nnzA,
const cusparseMatDescr_t descrA,
const float* csrValA,
const int* csrRowPtrA,
const int* csrColIndA,
float percentage,
const cusparseMatDescr_t descrC,
int* csrRowPtrC,
int* nnzTotalDevHostPtr,
pruneInfo_t info,
void* pBuffer)
cusparseStatus_t
cusparseDpruneCsr2csrNnzByPercentage(cusparseHandle_t handle,
int m,
int n,
int nnzA,
const cusparseMatDescr_t descrA,
const double* csrValA,
const int* csrRowPtrA,
const int* csrColIndA,
float percentage,
const cusparseMatDescr_t descrC,
int* csrRowPtrC,
int* nnzTotalDevHostPtr,
pruneInfo_t info,
void* pBuffer)
cusparseStatus_t
cusparseHpruneCsr2csrByPercentage(cusparseHandle_t handle,
int m,
int n,
int nnzA,
const cusparseMatDescr_t descrA,
const __half* csrValA,
const int* csrRowPtrA,
const int* csrColIndA,
float percentage,
const cusparseMatDescr_t descrC,
__half* csrValC,
const int* csrRowPtrC,
int* csrColIndC,
pruneInfo_t info,
void* pBuffer)
cusparseStatus_t
cusparseSpruneCsr2csrByPercentage(cusparseHandle_t handle,
int m,
int n,
int nnzA,
const cusparseMatDescr_t descrA,
const float* csrValA,
const int* csrRowPtrA,
const int* csrColIndA,
float percentage,
const cusparseMatDescr_t descrC,
float* csrValC,
const int* csrRowPtrC,
int* csrColIndC,
pruneInfo_t info,
void* pBuffer)
cusparseStatus_t
cusparseDpruneCsr2csrByPercentage(cusparseHandle_t handle,
int m,
int n,
int nnzA,
const cusparseMatDescr_t descrA,
const double* csrValA,
const int* csrRowPtrA,
const int* csrColIndA,
float percentage,
const cusparseMatDescr_t descrC,
double* csrValC,
const int* csrRowPtrC,
int* csrColIndC,
pruneInfo_t info,
void* pBuffer)
This function prunes a sparse matrix to a sparse matrix by percentage.
Given a sparse matrix A
and a non-negative value percentage
, the function computes sparse matrix C
by the following three steps:
Step 1: sort absolute value of A
in ascending order.
\(\begin{matrix} {key\ :=\ sort(\ |csrValA|\ )} \\ \end{matrix}\) |
Step 2: choose threshold by the parameter percentage
\(\begin{matrix} {pos\ =\ ceil(nnzA*(percentage/100))\ -\ 1} \\ {pos\ =\ min(pos,\ nnzA-1)} \\ {pos\ =\ max(pos,\ 0)} \\ {threshold\ =\ key\lbrack pos\rbrack} \\ \end{matrix}\) |
Step 3: call pruneCsr2csr()
by with the parameter threshold
.
The implementation adopts a two-step approach to do the conversion. First, the user allocates csrRowPtrC
of m+1
elements and uses function pruneCsr2csrNnzByPercentage()
to determine the number of nonzeros columns per row. Second, the user gathers nnzC
(number of nonzeros of matrix C
) from either (nnzC=*nnzTotalDevHostPtr)
or (nnzC=csrRowPtrC[m]-csrRowPtrC[0])
and allocates csrValC
of nnzC
elements and csrColIndC
of nnzC
integers. Finally function pruneCsr2csrByPercentage()
is called to complete the conversion.
The user must obtain the size of the buffer required by pruneCsr2csrByPercentage()
by calling pruneCsr2csrByPercentage_bufferSizeExt()
, allocate the buffer, and pass the buffer pointer to pruneCsr2csrByPercentage()
.
Remark 1: the value of percentage
must be not greater than 100. Otherwise, CUSPARSE_STATUS_INVALID_VALUE
is returned.
Examples of prune chapter provides a simple example of pruneCsr2csrByPercentage()
.
The routine cusparse<t>pruneCsr2csrNnzByPercentage()
has the following properties:
This function requires temporary extra storage that is allocated internally
The routine supports asynchronous execution if the Stream Ordered Memory Allocator is available
The routine supports CUDA graph capture if the Stream Ordered Memory Allocator is available
The routine cusparse<t>pruneCsr2csrByPercentage()
has the following properties:
The routine requires no extra storage
The routine supports asynchronous execution
The routine supports CUDA graph capture
Input
|
|
|
|
|
handle to the cuSPARSE library context. |
|
|
number of rows of matrix |
|
|
number of columns of matrix |
|
|
number of nonzeros of matrix |
|
|
the descriptor of matrix |
|
|
<type> array of |
|
|
integer array of |
|
|
integer array of |
|
|
percentage <=100 and percentage >= 0 |
|
|
the descriptor of matrix |
|
|
buffer allocated by the user; the size is returned by |
Output
|
|
|
|
|
total number of nonzero of matrix |
|
|
<type> array of |
|
|
integer array of |
|
|
integer array of |
|
|
number of bytes of the buffer. |
See cusparseStatus_t for the description of the return status.
13.19. cusparse<t>nnz_compress()
cusparseStatus_t
cusparseSnnz_compress(cusparseHandle_t handle,
int m,
const cusparseMatDescr_t descr,
const float* csrValA,
const int* csrRowPtrA,
int* nnzPerRow,
int* nnzC,
float tol)
cusparseStatus_t
cusparseDnnz_compress(cusparseHandle_t handle,
int m,
const cusparseMatDescr_t descr,
const double* csrValA,
const int* csrRowPtrA,
int* nnzPerRow,
int* nnzC,
double tol)
cusparseStatus_t
cusparseCnnz_compress(cusparseHandle_t handle,
int m,
const cusparseMatDescr_t descr,
const cuComplex* csrValA,
const int* csrRowPtrA,
int* nnzPerRow,
int* nnzC,
cuComplex tol)
cusparseStatus_t
cusparseZnnz_compress(cusparseHandle_t handle,
int m,
const cusparseMatDescr_t descr,
const cuDoubleComplex* csrValA,
const int* csrRowPtrA,
int* nnzPerRow,
int* nnzC,
cuDoubleComplex tol)
This function is the step one to convert from csr format to compressed csr format.
Given a sparse matrix A and a non-negative value threshold, the function returns nnzPerRow(the number of nonzeros columns per row) and nnzC(the total number of nonzeros) of a sparse matrix C, defined by
\(\begin{matrix} {{C(i,j)} = {A(i,j)}} & \text{if\ |A(i,j)|\ >\ threshold} \\ \end{matrix}\) |
A key assumption for the cuComplex and cuDoubleComplex case is that this tolerance is given as the real part. For example tol = 1e-8 + 0*i and we extract cureal, that is the x component of this struct.
This function requires temporary extra storage that is allocated internally
The routine supports asynchronous execution if the Stream Ordered Memory Allocator is available
The routine supports CUDA graph capture if the Stream Ordered Memory Allocator is available
Input
|
handle to the cuSPARSE library context. |
|
number of rows of matrix |
|
the descriptor of matrix |
|
csr noncompressed values array |
|
the corresponding input noncompressed row pointer. |
|
non-negative tolerance to determine if a number less than or equal to it. |
Output
|
this array contains the number of elements whose absolute values are greater than tol per row. |
|
host/device pointer of the total number of elements whose absolute values are greater than tol. |
See cusparseStatus_t for the description of the return status.
14. cuSPARSE Generic API Reference
The cuSPARSE Generic APIs allow computing the most common sparse linear algebra operations, such as sparse matrix-vector (SpMV) and sparse matrix-matrix multiplication (SpMM), in a flexible way. The new APIs have the following capabilities and features:
Set matrix data layouts, number of batches, and storage formats (for example, CSR, COO, and so on).
Set input/output/compute data types. This also allows mixed data-type computation.
Set types of sparse matrix indices.
Choose the algorithm for the computation.
Provide external device memory for internal operations.
Provide extensive consistency checks across input matrices and vectors for a given routine. This includes the validation of matrix sizes, data types, layout, allowed operations, and so.
Provide constant descriptors for vector and matrix inputs to support const-safe interface and guarantee that the APIs do not modify their inputs.
14.1. Generic Types Reference
The cuSPARSE generic type references are described in this section.
14.1.1. cudaDataType_t
The section describes the types shared by multiple CUDA Libraries and defined in the header file library_types.h
. The cudaDataType
type is an enumerator to specify the data precision. It is used when the data reference does not carry the type itself (e.g. void*
). For example, it is used in the routine cusparseSpMM()
.
Value |
Meaning |
Data Type |
Header |
---|---|---|---|
|
The data type is 16-bit IEEE-754 floating-point |
|
cuda_fp16.h |
|
The data type is 16-bit complex IEEE-754 floating-point |
|
cuda_fp16.h |
|
The data type is 16-bit bfloat floating-point |
|
cuda_bf16.h |
|
The data type is 16-bit complex bfloat floating-point |
|
cuda_bf16.h |
|
The data type is 32-bit IEEE-754 floating-point |
|
|
|
The data type is 32-bit complex IEEE-754 floating-point |
|
cuComplex.h |
|
The data type is 64-bit IEEE-754 floating-point |
|
|
|
The data type is 64-bit complex IEEE-754 floating-point |
|
cuComplex.h |
|
The data type is 8-bit integer |
|
stdint.h |
|
The data type is 32-bit integer |
|
stdint.h |
IMPORTANT: The Generic API routines allow all data types reported in the respective section of the documentation only on GPU architectures with native support for them. If a specific GPU model does not provide native support for a given data type, the routine returns CUSPARSE_STATUS_ARCH_MISMATCH
error.
Unsupported data types and Compute Capability (CC):
__half
on GPUs withCC < 53
(e.g. Kepler)__nv_bfloat16
on GPUs withCC < 80
(e.g. Kepler, Maxwell, Pascal, Volta, Turing)
14.1.2. cusparseFormat_t
This type indicates the format of the sparse matrix.
Value |
Meaning |
---|---|
|
The matrix is stored in Coordinate (COO) format organized in Structure of Arrays (SoA) layout |
|
The matrix is stored in Compressed Sparse Row (CSR) format |
|
The matrix is stored in Compressed Sparse Column (CSC) format |
|
The matrix is stored in Blocked-Ellpack (Blocked-ELL) format |
14.1.3. cusparseOrder_t
This type indicates the memory layout of a dense matrix.
Value |
Meaning |
---|---|
|
The matrix is stored in row-major |
|
The matrix is stored in column-major |
14.1.4. cusparseIndexType_t
This type indicates the index type for representing the sparse matrix indices.
Value |
Meaning |
---|---|
|
16-bit unsigned integer [1, 65535] |
|
32-bit signed integer [1, 2^31 - 1] |
|
64-bit signed integer [1, 2^63 - 1] |
14.2. Sparse Vector APIs
The cuSPARSE helper functions for sparse vector descriptor are described in this section.
14.2.1. cusparseCreateSpVec()
cusparseStatus_t
cusparseCreateSpVec(cusparseSpVecDescr_t* spVecDescr,
int64_t size,
int64_t nnz,
void* indices,
void* values,
cusparseIndexType_t idxType,
cusparseIndexBase_t idxBase,
cudaDataType valueType)
cusparseStatus_t
cusparseCreateConstSpVec(cusparseSpVecDescr_t* spVecDescr, //const descriptor
int64_t size,
int64_t nnz,
void* indices,
void* values,
cusparseIndexType_t idxType,
cusparseIndexBase_t idxBase,
cudaDataType valueType)
This function initializes the sparse matrix descriptor spVecDescr
.
Param. |
Memory |
In/out |
Meaning |
---|---|---|---|
|
HOST |
OUT |
Sparse vector descriptor |
|
HOST |
IN |
Size of the sparse vector |
|
HOST |
IN |
Number of non-zero entries of the sparse vector |
|
DEVICE |
IN |
Indices of the sparse vector. Array of size |
|
DEVICE |
IN |
Values of the sparse vector. Array of size |
|
HOST |
IN |
Enumerator specifying the data type of |
|
HOST |
IN |
Enumerator specifying the the base index of |
|
HOST |
IN |
Enumerator specifying the datatype of |
Note
It is safe to cast away constness (const_cast
) for input pointers if the descriptor will not be used as an output parameter of a routine (e.g. conversion functions).
Note
It is recommended to use constness (that is, by using
cusparseCreateConst...
) for sparse and dense vector and matrix pointers if the descriptor will not be used as an output parameter of a routine (such as conversion functions).The new generic API functions pass input vector and matrix pointers as constant descriptors (that is,
//const descriptors
).
See cusparseStatus_t for the description of the return status.
14.2.2. cusparseDestroySpVec()
cusparseStatus_t
cusparseDestroySpVec(cusparseSpVecDescr_t spVecDescr)
This function releases the host memory allocated for the sparse vector descriptor spVecDescr
.
Param. |
Memory |
In/out |
Meaning |
---|---|---|---|
|
HOST |
IN |
Sparse vector descriptor |
See cusparseStatus_t for the description of the return status.
14.2.3. cusparseSpVecGet()
cusparseStatus_t
cusparseSpVecGet(cusparseSpVecDescr_t spVecDescr,
int64_t* size,
int64_t* nnz,
void** indices,
void** values,
cusparseIndexType_t* idxType,
cusparseIndexBase_t* idxBase,
cudaDataType* valueType)
cusparseStatus_t
cusparseConstSpVecGet(cusparseSpVecDescr_t spVecDescr, //const descriptor
int64_t* size,
int64_t* nnz,
void** indices,
void** values,
cusparseIndexType_t* idxType,
cusparseIndexBase_t* idxBase,
cudaDataType* valueType)
This function returns the fields of the sparse vector descriptor spVecDescr
.
Param. |
Memory |
In/out |
Meaning |
---|---|---|---|
|
HOST |
IN |
Sparse vector descriptor |
|
HOST |
OUT |
Size of the sparse vector |
|
HOST |
OUT |
Number of non-zero entries of the sparse vector |
|
DEVICE |
OUT |
Indices of the sparse vector. Array of size |
|
DEVICE |
OUT |
Values of the sparse vector. Array of size |
|
HOST |
OUT |
Enumerator specifying the data type of |
|
HOST |
OUT |
Enumerator specifying the the base index of |
|
HOST |
OUT |
Enumerator specifying the datatype of |
See cusparseStatus_t for the description of the return status.
14.2.4. cusparseSpVecGetIndexBase()
cusparseStatus_t
cusparseSpVecGetIndexBase(cusparseSpVecDescr_t spVecDescr, //const descriptor
cusparseIndexBase_t* idxBase)
This function returns the idxBase
field of the sparse vector descriptor spVecDescr
.
Param. |
Memory |
In/out |
Meaning |
---|---|---|---|
|
HOST |
IN |
Sparse vector descriptor |
|
HOST |
OUT |
Enumerator specifying the the base index of |
See cusparseStatus_t for the description of the return status.
14.2.5. cusparseSpVecGetValues()
cusparseStatus_t
cusparseSpVecGetValues(cusparseSpVecDescr_t spVecDescr,
void** values)
cusparseStatus_t
cusparseConstSpVecGetValues(cusparseSpVecDescr_t spVecDescr, //const descriptor
void** values)
This function returns the values
field of the sparse vector descriptor spVecDescr
.
Param. |
Memory |
In/out |
Meaning |
---|---|---|---|
|
HOST |
IN |
Sparse vector descriptor |
|
DEVICE |
OUT |
Values of the sparse vector. Array of size |
See cusparseStatus_t for the description of the return status.
14.2.6. cusparseSpVecSetValues()
cusparseStatus_t
cusparseSpVecSetValues(cusparseSpVecDescr_t spVecDescr,
void* values)
This function set the values
field of the sparse vector descriptor spVecDescr
.
Param. |
Memory |
In/out |
Meaning |
---|---|---|---|
|
HOST |
IN |
Sparse vector descriptor |
|
DEVICE |
IN |
Values of the sparse vector. Array of size |
See cusparseStatus_t for the description of the return status.
14.3. Sparse Matrix APIs
The cuSPARSE helper functions for sparse matrix descriptor are described in this section.
14.3.1. cusparseCreateCoo()
cusparseStatus_t
cusparseCreateCoo(cusparseSpMatDescr_t* spMatDescr,
int64_t rows,
int64_t cols,
int64_t nnz,
void* cooRowInd,
void* cooColInd,
void* cooValues,
cusparseIndexType_t cooIdxType,
cusparseIndexBase_t idxBase,
cudaDataType valueType)
cusparseStatus_t
cusparseCreateConstCoo(cusparseSpMatDescr_t* spMatDescr, //const descriptor
int64_t rows,
int64_t cols,
int64_t nnz,
void* cooRowInd,
void* cooColInd,
void* cooValues,
cusparseIndexType_t cooIdxType,
cusparseIndexBase_t idxBase,
cudaDataType valueType)
This function initializes the sparse matrix descriptor spMatDescr
in the COO format (Structure of Arrays layout).
Param. |
Memory |
In/out |
Meaning |
---|---|---|---|
|
HOST |
OUT |
Sparse matrix descriptor |
|
HOST |
IN |
Number of rows of the sparse matrix |
|
HOST |
IN |
Number of columns of the sparse matrix |
|
HOST |
IN |
Number of non-zero entries of the sparse matrix |
|
DEVICE |
IN |
Row indices of the sparse matrix. Array of size |
|
DEVICE |
IN |
Column indices of the sparse matrix. Array of size |
|
DEVICE |
IN |
Values of the sparse matrix. Array of size |
|
HOST |
IN |
Data type of |
|
HOST |
IN |
Base index of |
|
HOST |
IN |
Datatype of |
NOTE: it is safe to cast away constness (const_cast
) for input pointers if the descriptor will not be used as an output parameter of a routine (e.g. conversion functions).
See cusparseStatus_t for the description of the return status.
14.3.2. cusparseCreateCsr()
cusparseStatus_t
cusparseCreateCsr(cusparseSpMatDescr_t* spMatDescr,
int64_t rows,
int64_t cols,
int64_t nnz,
void* csrRowOffsets,
void* csrColInd,
void* csrValues,
cusparseIndexType_t csrRowOffsetsType,
cusparseIndexType_t csrColIndType,
cusparseIndexBase_t idxBase,
cudaDataType valueType)
cusparseStatus_t
cusparseCreateConstCsr(cusparseSpMatDescr_t* spMatDescr, //const descriptor
int64_t rows,
int64_t cols,
int64_t nnz,
void* csrRowOffsets,
void* csrColInd,
void* csrValues,
cusparseIndexType_t csrRowOffsetsType,
cusparseIndexType_t csrColIndType,
cusparseIndexBase_t idxBase,
cudaDataType valueType)
This function initializes the sparse matrix descriptor spMatDescr
in the CSR format.
Param. |
Memory |
In/out |
Meaning |
---|---|---|---|
|
HOST |
OUT |
Sparse matrix descriptor |
|
HOST |
IN |
Number of rows of the sparse matrix |
|
HOST |
IN |
Number of columns of the sparse matrix |
|
HOST |
IN |
Number of non-zero entries of the sparse matrix |
|
DEVICE |
IN |
Row offsets of the sparse matrix. Array of size |
|
DEVICE |
IN |
Column indices of the sparse matrix. Array of size |
|
DEVICE |
IN |
Values of the sparse matrix. Array of size |
|
HOST |
IN |
Data type of |
|
HOST |
IN |
Data type of |
|
HOST |
IN |
Base index of |
|
HOST |
IN |
Datatype of |
NOTE: it is safe to cast away constness (const_cast
) for input pointers if the descriptor will not be used as an output parameter of a routine (e.g. conversion functions).
See cusparseStatus_t for the description of the return status.
14.3.3. cusparseCreateCsc()
cusparseStatus_t
cusparseCreateCsc(cusparseSpMatDescr_t* spMatDescr,
int64_t rows,
int64_t cols,
int64_t nnz,
void* cscColOffsets,
void* cscRowInd,
void* cscValues,
cusparseIndexType_t cscColOffsetsType,
cusparseIndexType_t cscRowIndType,
cusparseIndexBase_t idxBase,
cudaDataType valueType)
cusparseStatus_t
cusparseCreateConstCsc(cusparseSpMatDescr_t* spMatDescr, //const descriptor
int64_t rows,
int64_t cols,
int64_t nnz,
void* cscColOffsets,
void* cscRowInd,
void* cscValues,
cusparseIndexType_t cscColOffsetsType,
cusparseIndexType_t cscRowIndType,
cusparseIndexBase_t idxBase,
cudaDataType valueType)
This function initializes the sparse matrix descriptor spMatDescr
in the CSC format.
Param. |
Memory |
In/out |
Meaning |
---|---|---|---|
|
HOST |
OUT |
Sparse matrix descriptor |
|
HOST |
IN |
Number of rows of the sparse matrix |
|
HOST |
IN |
Number of columns of the sparse matrix |
|
HOST |
IN |
Number of non-zero entries of the sparse matrix |
|
DEVICE |
IN |
Column offsets of the sparse matrix. Array of size |
|
DEVICE |
IN |
Row indices of the sparse matrix. Array of size |
|
DEVICE |
IN |
Values of the sparse matrix. Array of size |
|
HOST |
IN |
Data type of |
|
HOST |
IN |
Data type of |
|
HOST |
IN |
Base index of |
|
HOST |
IN |
Datatype of |
14.3.4. cusparseCreateBlockedEll()
cusparseStatus_t
cusparseCreateBlockedEll(cusparseSpMatDescr_t* spMatDescr,
int64_t rows,
int64_t cols,
int64_t ellBlockSize,
int64_t ellCols,
void* ellColInd,
void* ellValue,
cusparseIndexType_t ellIdxType,
cusparseIndexBase_t idxBase,
cudaDataType valueType)
cusparseStatus_t
cusparseCreateConstBlockedEll(cusparseSpMatDescr_t* spMatDescr, //const descriptor
int64_t rows,
int64_t cols,
int64_t ellBlockSize,
int64_t ellCols,
void* ellColInd,
void* ellValue,
cusparseIndexType_t ellIdxType,
cusparseIndexBase_t idxBase,
cudaDataType valueType)
This function initializes the sparse matrix descriptor spMatDescr
for the Blocked-Ellpack (ELL) format.
Param. |
Memory |
In/out |
Meaning |
---|---|---|---|
|
HOST |
OUT |
Sparse matrix descriptor |
|
HOST |
IN |
Number of rows of the sparse matrix |
|
HOST |
IN |
Number of columns of the sparse matrix |
|
HOST |
IN |
Size of the ELL-Block |
|
HOST |
IN |
Actual number of columns of the Blocked-Ellpack format ( |
|
DEVICE |
IN |
Blocked-ELL Column indices. Array of size |
|
DEVICE |
IN |
Values of the sparse matrix. Array of size |
|
HOST |
IN |
Data type of |
|
HOST |
IN |
Base index of |
|
HOST |
IN |
Datatype of |
Blocked-ELL Column indices (ellColInd
) are in the range [0, cols / ellBlockSize -1]
. The array can contain -1
values for indicating empty blocks.
Note
It is safe to cast away constness (const_cast
) for input pointers if the descriptor will not be used as an output parameter of a routine (e.g. conversion functions).
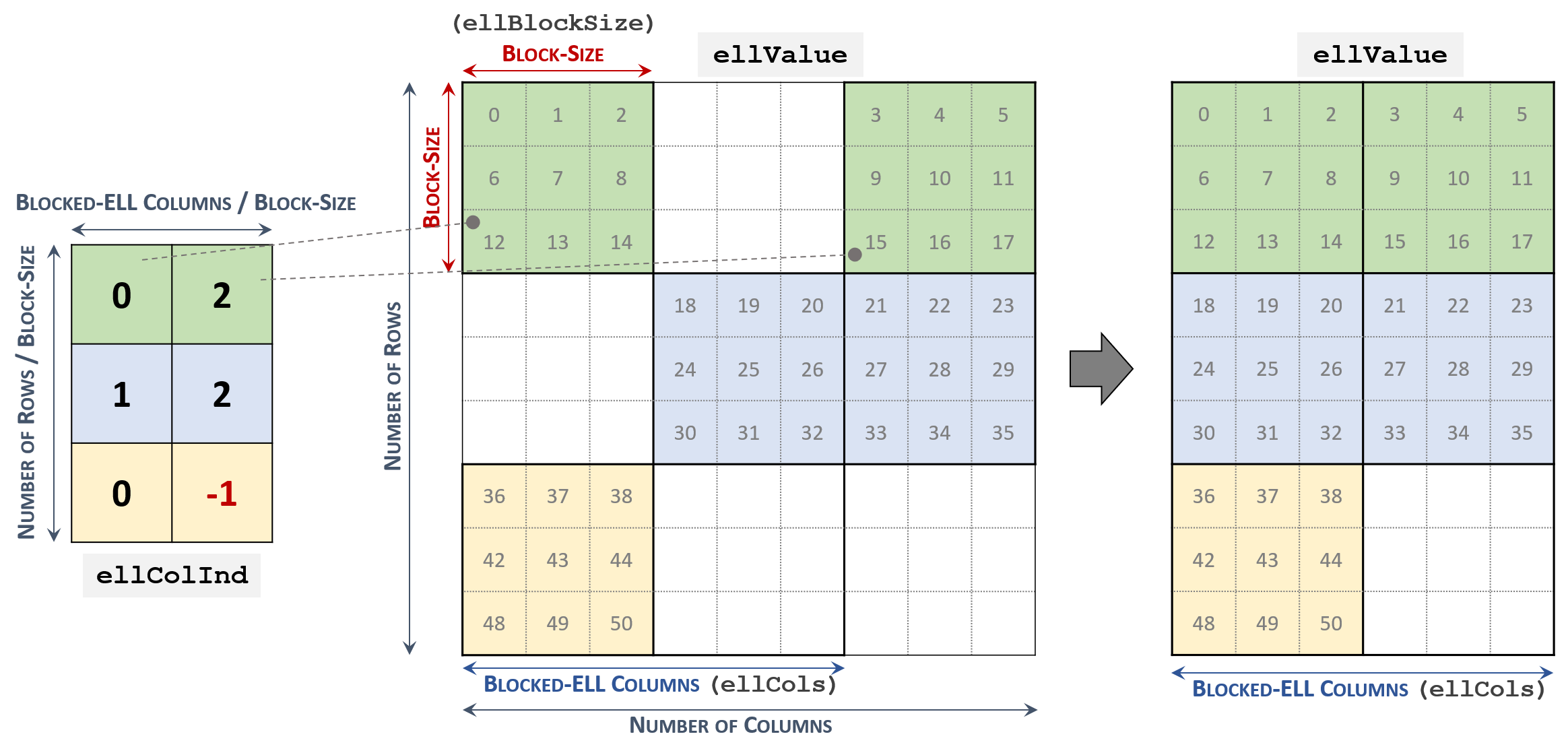
Blocked-ELL representation
See cusparseStatus_t for the description of the return status.
14.3.5. cusparseDestroySpMat()
cusparseStatus_t
cusparseDestroySpMat(cusparseConstSpMatDescr_t spMatDescrr /*const descriptor*/)
This function releases the host memory allocated for the sparse matrix descriptor spMatDescr
.
Param. |
Memory |
In/out |
Meaning |
---|---|---|---|
|
HOST |
IN |
Sparse matrix descriptor |
See cusparseStatus_t for the description of the return status.
14.3.6. cusparseCooGet()
cusparseStatus_t
cusparseCooGet(cusparseSpMatDescr_t spMatDescr,
int64_t* rows,
int64_t* cols,
int64_t* nnz,
void** cooRowInd,
void** cooColInd,
void** cooValues,
cusparseIndexType_t* idxType,
cusparseIndexBase_t* idxBase,
cudaDataType* valueType)
cusparseStatus_t
cusparseConstCooGet(cusparseSpMatDescr_t spMatDescr, //const descriptor
int64_t* rows,
int64_t* cols,
int64_t* nnz,
void** cooRowInd,
void** cooColInd,
void** cooValues,
cusparseIndexType_t* idxType,
cusparseIndexBase_t* idxBase,
cudaDataType* valueType)
This function returns the fields of the sparse matrix descriptor spMatDescr
stored in COO format (Array of Structures layout).
Param. |
Memory |
In/out |
Meaning |
---|---|---|---|
|
HOST |
IN |
Sparse matrix descriptor |
|
HOST |
OUT |
Number of rows of the sparse matrix |
|
HOST |
OUT |
Number of columns of the sparse matrix |
|
HOST |
OUT |
Number of non-zero entries of the sparse matrix |
|
DEVICE |
OUT |
Row indices of the sparse matrix. Array of size |
|
DEVICE |
OUT |
Column indices of the sparse matrix. Array of size |
|
DEVICE |
OUT |
Values of the sparse matrix. Array of size |
|
HOST |
OUT |
Data type of |
|
HOST |
OUT |
Base index of |
|
HOST |
OUT |
Datatype of |
See cusparseStatus_t for the description of the return status.
14.3.7. cusparseCsrGet()
cusparseStatus_t
cusparseCsrGet(cusparseSpMatDescr_t spMatDescr,
int64_t* rows,
int64_t* cols,
int64_t* nnz,
void** csrRowOffsets,
void** csrColInd,
void** csrValues,
cusparseIndexType_t* csrRowOffsetsType,
cusparseIndexType_t* csrColIndType,
cusparseIndexBase_t* idxBase,
cudaDataType* valueType)
cusparseStatus_t
cusparseConstCsrGet(cusparseSpMatDescr_t spMatDescr, //const descriptor
int64_t* rows,
int64_t* cols,
int64_t* nnz,
void** csrRowOffsets,
void** csrColInd,
void** csrValues,
cusparseIndexType_t* csrRowOffsetsType,
cusparseIndexType_t* csrColIndType,
cusparseIndexBase_t* idxBase,
cudaDataType* valueType)
This function returns the fields of the sparse matrix descriptor spMatDescr
stored in CSR format.
Param. |
Memory |
In/out |
Meaning |
---|---|---|---|
|
HOST |
IN |
Sparse matrix descriptor |
|
HOST |
OUT |
Number of rows of the sparse matrix |
|
HOST |
OUT |
Number of columns of the sparse matrix |
|
HOST |
OUT |
Number of non-zero entries of the sparse matrix |
|
DEVICE |
OUT |
Row offsets of the sparse matrix. Array of size |
|
DEVICE |
OUT |
Column indices of the sparse matrix. Array of size |
|
DEVICE |
OUT |
Values of the sparse matrix. Array of size |
|
HOST |
OUT |
Data type of |
|
HOST |
OUT |
Data type of |
|
HOST |
OUT |
Base index of |
|
HOST |
OUT |
Datatype of |
See cusparseStatus_t for the description of the return status.
14.3.8. cusparseCscGet()
cusparseStatus_t
cusparseCscGet(cusparseSpMatDescr_t spMatDescr,
int64_t* rows,
int64_t* cols,
int64_t* nnz,
void** cscRowOffsets,
void** cscColInd,
void** cscValues,
cusparseIndexType_t* cscRowOffsetsType,
cusparseIndexType_t* cscColIndType,
cusparseIndexBase_t* idxBase,
cudaDataType* valueType)
cusparseStatus_t
cusparseConstCscGet(cusparseSpMatDescr_t spMatDescr, //const descriptor
int64_t* rows,
int64_t* cols,
int64_t* nnz,
void** cscRowOffsets,
void** cscColInd,
void** cscValues,
cusparseIndexType_t* cscRowOffsetsType,
cusparseIndexType_t* cscColIndType,
cusparseIndexBase_t* idxBase,
cudaDataType* valueType)
This function returns the fields of the sparse matrix descriptor spMatDescr
stored in CSC format.
Param. |
Memory |
In/out |
Meaning |
---|---|---|---|
|
HOST |
IN |
Sparse matrix descriptor |
|
HOST |
OUT |
Number of rows of the sparse matrix |
|
HOST |
OUT |
Number of columns of the sparse matrix |
|
HOST |
OUT |
Number of non-zero entries of the sparse matrix |
|
DEVICE |
OUT |
Row offsets of the sparse matrix. Array of size |
|
DEVICE |
OUT |
Column indices of the sparse matrix. Array of size |
|
DEVICE |
OUT |
Values of the sparse matrix. Array of size |
|
HOST |
OUT |
Data type of |
|
HOST |
OUT |
Data type of |
|
HOST |
OUT |
Base index of |
|
HOST |
OUT |
Datatype of |
See cusparseStatus_t for the description of the return status.
14.3.9. cusparseCsrSetPointers()
cusparseStatus_t
cusparseCsrSetPointers(cusparseSpMatDescr_t spMatDescr,
void* csrRowOffsets,
void* csrColInd,
void* csrValues)
This function sets the pointers of the sparse matrix descriptor spMatDescr
.
Param. |
Memory |
In/out |
Meaning |
---|---|---|---|
|
HOST |
IN |
Sparse matrix descriptor |
|
DEVICE |
IN |
Row offsets of the sparse matrix. Array of size |
|
DEVICE |
IN |
Column indices of the sparse matrix. Array of size |
|
DEVICE |
IN |
Values of the sparse matrix. Array of size |
See cusparseStatus_t for the description of the return status.
14.3.10. cusparseCscSetPointers()
cusparseStatus_t
cusparseCscSetPointers(cusparseSpMatDescr_t spMatDescr,
void* cscColOffsets,
void* cscRowInd,
void* cscValues)
This function sets the pointers of the sparse matrix descriptor spMatDescr
.
Param. |
Memory |
In/out |
Meaning |
---|---|---|---|
|
HOST |
IN |
Sparse matrix descriptor |
|
DEVICE |
IN |
Col offsets of the sparse matrix. Array of size |
|
DEVICE |
IN |
Row indices of the sparse matrix. Array of size |
|
DEVICE |
IN |
Values of the sparse matrix. Array of size |
See cusparseStatus_t for the description of the return status.
14.3.11. cusparseCooSetPointers()
cusparseStatus_t
cusparseCooSetPointers(cusparseSpMatDescr_t spMatDescr,
void* cooRows,
void* cooColumns,
void* cooValues)
This function sets the pointers of the sparse matrix descriptor spMatDescr
.
Param. |
Memory |
In/out |
Meaning |
---|---|---|---|
|
HOST |
IN |
Sparse matrix descriptor |
|
DEVICE |
IN |
Row indices of the sparse matrix. Array of size |
|
DEVICE |
IN |
Column indices of the sparse matrix. Array of size |
|
DEVICE |
IN |
Values of the sparse matrix. Array of size |
See cusparseStatus_t for the description of the return status.
14.3.12. cusparseBlockedEllGet()
cusparseStatus_t
cusparseBlockedEllGet(cusparseSpMatDescr_t spMatDescr,
int64_t* rows,
int64_t* cols,
int64_t* ellBlockSize,
int64_t* ellCols,
void** ellColInd,
void** ellValue,
cusparseIndexType_t* ellIdxType,
cusparseIndexBase_t* idxBase,
cudaDataType* valueType)
cusparseStatus_t
cusparseConstBlockedEllGet(cusparseSpMatDescr_t spMatDescr, //const descriptor
int64_t* rows,
int64_t* cols,
int64_t* ellBlockSize,
int64_t* ellCols,
void** ellColInd,
void** ellValue,
cusparseIndexType_t* ellIdxType,
cusparseIndexBase_t* idxBase,
cudaDataType* valueType)
This function returns the fields of the sparse matrix descriptor spMatDescr
stored in Blocked-Ellpack (ELL) format.
Param. |
Memory |
In/out |
Meaning |
---|---|---|---|
|
HOST |
IN |
Sparse matrix descriptor |
|
HOST |
OUT |
Number of rows of the sparse matrix |
|
HOST |
OUT |
Number of columns of the sparse matrix |
|
HOST |
OUT |
Size of the ELL-Block |
|
HOST |
OUT |
Actual number of columns of the Blocked-Ellpack format |
|
DEVICE |
OUT |
Column indices for the ELL-Block. Array of size |
|
DEVICE |
OUT |
Values of the sparse matrix. Array of size |
|
HOST |
OUT |
Data type of |
|
HOST |
OUT |
Base index of |
|
HOST |
OUT |
Datatype of |
See cusparseStatus_t for the description of the return status.
14.3.13. cusparseSpMatGetSize()
cusparseStatus_t
cusparseSpMatGetSize(cusparseSpMatDescr_t spMatDescr, //const descriptor
int64_t* rows,
int64_t* cols,
int64_t* nnz)
This function returns the sizes of the sparse matrix spMatDescr
.
Param. |
Memory |
In/out |
Meaning |
---|---|---|---|
|
HOST |
IN |
Sparse matrix descriptor |
|
HOST |
OUT |
Number of rows of the sparse matrix |
|
HOST |
OUT |
Number of columns of the sparse matrix |
|
HOST |
OUT |
Number of non-zero entries of the sparse matrix |
See cusparseStatus_t for the description of the return status.
14.3.14. cusparseSpMatGetFormat()
cusparseStatus_t
cusparseSpMatGetFormat(cusparseSpMatDescr_t spMatDescr, //const descriptor
cusparseFormat_t* format)
This function returns the format
field of the sparse matrix descriptor spMatDescr
.
Param. |
Memory |
In/out |
Meaning |
---|---|---|---|
|
HOST |
IN |
Sparse matrix descriptor |
|
HOST |
OUT |
Storage format of the sparse matrix |
See cusparseStatus_t for the description of the return status.
14.3.15. cusparseSpMatGetIndexBase()
cusparseStatus_t
cusparseSpMatGetIndexBase(cusparseSpMatDescr_t spMatDescr, //const descriptor
cusparseIndexBase_t* idxBase)
This function returns the idxBase
field of the sparse matrix descriptor spMatDescr
.
Param. |
Memory |
In/out |
Meaning |
---|---|---|---|
|
HOST |
IN |
Sparse matrix descriptor |
|
HOST |
OUT |
Base index of the sparse matrix |
See cusparseStatus_t for the description of the return status.
14.3.16. cusparseSpMatGetValues()
cusparseStatus_t
cusparseSpMatGetValues(cusparseSpMatDescr_t spMatDescr,
void** values)
cusparseStatus_t
cusparseConstSpMatGetValues(cusparseSpMatDescr_t spMatDescr, //const descriptor
void** values)
This function returns the values
field of the sparse matrix descriptor spMatDescr
.
Param. |
Memory |
In/out |
Meaning |
---|---|---|---|
|
HOST |
IN |
Sparse matrix descriptor |
|
DEVICE |
OUT |
Values of the sparse matrix. Array of size |
See cusparseStatus_t for the description of the return status.
14.3.17. cusparseSpMatSetValues()
cusparseStatus_t
cusparseSpMatSetValues(cusparseSpMatDescr_t spMatDescr,
void* values)
This function sets the values
field of the sparse matrix descriptor spMatDescr
.
Param. |
Memory |
In/out |
Meaning |
---|---|---|---|
|
HOST |
IN |
Sparse matrix descriptor |
|
DEVICE |
IN |
Values of the sparse matrix. Array of size |
See cusparseStatus_t for the description of the return status.
14.3.18. cusparseSpMatGetStridedBatch()
cusparseStatus_t
cusparseSpMatGetStridedBatch(cusparseSpMatDescr_t spMatDescr, //const descriptor
int* batchCount)
This function returns the batchCount
field of the sparse matrixdescriptor spMatDescr
.
Param. |
Memory |
In/out |
Meaning |
---|---|---|---|
|
HOST |
IN |
Sparse matrix descriptor |
|
HOST |
OUT |
Number of batches of the sparse matrix |
See cusparseStatus_t for the description of the return status.
14.3.19. cusparseCooSetStridedBatch()
cusparseStatus_t
cusparseCooSetStridedBatch(cusparseSpMatDescr_t spMatDescr,
int batchCount,
int64_t batchStride)
This function sets the batchCount
and the batchStride
fields of the sparse matrix descriptor spMatDescr
.
Param. |
Memory |
In/out |
Meaning |
---|---|---|---|
|
HOST |
IN |
Sparse matrix descriptor |
|
HOST |
IN |
Number of batches of the sparse matrix |
|
HOST |
IN |
address offset between consecutive batches |
See cusparseStatus_t for the description of the return status.
14.3.20. cusparseCsrSetStridedBatch()
cusparseStatus_t
cusparseCsrSetStridedBatch(cusparseSpMatDescr_t spMatDescr,
int batchCount,
int64_t offsetsBatchStride,
int64_t columnsValuesBatchStride)
This function sets the batchCount
and the batchStride
fields of the sparse matrix descriptor spMatDescr
.
Param. |
Memory |
In/out |
Meaning |
---|---|---|---|
|
HOST |
IN |
Sparse matrix descriptor |
|
HOST |
IN |
Number of batches of the sparse matrix |
|
HOST |
IN |
Address offset between consecutive batches for the row offset array |
|
HOST |
IN |
Address offset between consecutive batches for the column and value arrays |
See cusparseStatus_t for the description of the return status.
14.3.21. cusparseSpMatGetAttribute()
cusparseStatus_t
cusparseSpMatGetAttribute(cusparseSpMatDescr_t spMatDescr, //const descriptor
cusparseSpMatAttribute_t attribute,
void* data,
size_t dataSize)
The function gets the attributes of the sparse matrix descriptor spMatDescr
.
Param. |
Memory |
In/out |
Meaning |
---|---|---|---|
|
HOST |
IN |
Sparse matrix descriptor |
|
HOST |
IN |
Attribute enumerator |
|
HOST |
OUT |
Attribute value |
|
HOST |
IN |
Size of the attribute in bytes for safety |
Attribute |
Meaning |
Possible Values |
---|---|---|
|
Indicates if the lower or upper part of a matrix is stored in sparse storage |
|
|
Indicates if the matrix diagonal entries are unity |
|
See cusparseStatus_t for the description of the return status.
14.3.22. cusparseSpMatSetAttribute()
cusparseStatus_t
cusparseSpMatSetAttribute(cusparseSpMatDescr_t spMatDescr,
cusparseSpMatAttribute_t attribute,
const void* data,
size_t dataSize)
The function sets the attributes of the sparse matrix descriptor spMatDescr
Param. |
Memory |
In/out |
Meaning |
---|---|---|---|
|
HOST |
OUT |
Sparse matrix descriptor |
|
HOST |
IN |
Attribute enumerator |
|
HOST |
IN |
Attribute value |
|
HOST |
IN |
Size of the attribute in bytes for safety |
Attribute |
Meaning |
Possible Values |
---|---|---|
|
Indicates if the lower or upper part of a matrix is stored in sparse storage |
|
|
Indicates if the matrix diagonal entries are unity |
|
See cusparseStatus_t
for the description of the return status.
14.4. Dense Vector APIs
The cuSPARSE helper functions for dense vector descriptor are described in this section.
14.4.1. cusparseCreateDnVec()
cusparseStatus_t
cusparseCreateDnVec(cusparseDnVecDescr_t* dnVecDescr,
int64_t size,
void* values,
cudaDataType valueType)
cusparseStatus_t
cusparseCreateConstDnVec(cusparseDnVecDescr_t* dnVecDescr, //const descriptor
int64_t size,
void* values,
cudaDataType valueType)
This function initializes the dense vector descriptor dnVecDescr
.
Param. |
Memory |
In/out |
Meaning |
---|---|---|---|
|
HOST |
OUT |
Dense vector descriptor |
|
HOST |
IN |
Size of the dense vector |
|
DEVICE |
IN |
Values of the dense vector. Array of size |
|
HOST |
IN |
Enumerator specifying the datatype of |
NOTE: it is safe to cast away constness (const_cast
) for input pointers if the descriptor will not be used as an output parameter of a routine (e.g. conversion functions).
See cusparseStatus_t for the description of the return status.
14.4.2. cusparseDestroyDnVec()
cusparseStatus_t
cusparseDestroyDnVec(cusparseDnVecDescr_t dnVecDescr /*const descriptor*/)
This function releases the host memory allocated for the dense vector descriptor dnVecDescr
.
Param. |
Memory |
In/out |
Meaning |
---|---|---|---|
|
HOST |
IN |
Dense vector descriptor |
See cusparseStatus_t for the description of the return status.
14.4.3. cusparseDnVecGet()
cusparseStatus_t
cusparseDnVecGet(cusparseDnVecDescr_t dnVecDescr,
int64_t* size,
void** values,
cudaDataType* valueType)
cusparseStatus_t
cusparseConstDnVecGet(cusparseDnVecDescr_t dnVecDescr, //const descriptor
int64_t* size,
void** values,
cudaDataType* valueType)
This function returns the fields of the dense vector descriptor dnVecDescr
.
Param. |
Memory |
In/out |
Meaning |
---|---|---|---|
|
HOST |
IN |
Dense vector descriptor |
|
HOST |
OUT |
Size of the dense vector |
|
DEVICE |
OUT |
Values of the dense vector. Array of size |
|
HOST |
OUT |
Enumerator specifying the datatype of |
See cusparseStatus_t for the description of the return status.
14.4.4. cusparseDnVecGetValues()
cusparseStatus_t
cusparseDnVecGetValues(cusparseDnVecDescr_t dnVecDescr,
void** values)
cusparseStatus_t
cusparseConstDnVecGetValues(cusparseDnVecDescr_t dnVecDescr, //const descriptor
void** values)
This function returns the values
field of the dense vector descriptor dnVecDescr
.
Param. |
Memory |
In/out |
Meaning |
---|---|---|---|
|
HOST |
IN |
Dense vector descriptor |
|
DEVICE |
OUT |
Values of the dense vector |
See cusparseStatus_t for the description of the return status.
14.4.5. cusparseDnVecSetValues()
cusparseStatus_t
cusparseDnVecSetValues(cusparseDnVecDescr_t dnVecDescr,
void* values)
This function set the values
field of the dense vector descriptor dnVecDescr
.
Param. |
Memory |
In/out |
Meaning |
---|---|---|---|
|
HOST |
IN |
Dense vector descriptor |
|
DEVICE |
IN |
Values of the dense vector. Array of size |
The possible error values returned by this function and their meanings are listed below:
See cusparseStatus_t for the description of the return status.
14.5. Dense Matrix APIs
The cuSPARSE helper functions for dense matrix descriptor are described in this section.
14.5.1. cusparseCreateDnMat()
cusparseStatus_t
cusparseCreateDnMat(cusparseDnMatDescr_t* dnMatDescr,
int64_t rows,
int64_t cols,
int64_t ld,
void* values,
cudaDataType valueType,
cusparseOrder_t order)
cusparseStatus_t
cusparseCreateConstDnMat(cusparseDnMatDescr_t* dnMatDescr, //const descriptor
int64_t rows,
int64_t cols,
int64_t ld,
void* values,
cudaDataType valueType,
cusparseOrder_t order)
The function initializes the dense matrix descriptor dnMatDescr
.
Param. |
Memory |
In/out |
Meaning |
---|---|---|---|
|
HOST |
OUT |
Dense matrix descriptor |
|
HOST |
IN |
Number of rows of the dense matrix |
|
HOST |
IN |
Number of columns of the dense matrix |
|
HOST |
IN |
Leading dimension of the dense matrix |
|
DEVICE |
IN |
Values of the dense matrix. Array of size |
|
HOST |
IN |
Enumerator specifying the datatype of |
|
HOST |
IN |
Enumerator specifying the memory layout of the dense matrix |
NOTE: it is safe to cast away constness (const_cast
) for input pointers if the descriptor will not be used as an output parameter of a routine (e.g. conversion functions).
See cusparseStatus_t for the description of the return status.
14.5.2. cusparseDestroyDnMat()
cusparseStatus_t
cusparseDestroyDnMat(cusparseDnMatDescr_t dnMatDescr /*const descriptor*/)
This function releases the host memory allocated for the dense matrix descriptor dnMatDescr
.
Param. |
Memory |
In/out |
Meaning |
---|---|---|---|
|
HOST |
IN |
Dense matrix descriptor |
See cusparseStatus_t for the description of the return status.
14.5.3. cusparseDnMatGet()
cusparseStatus_t
cusparseDnMatGet(cusparseDnMatDescr_t dnMatDescr,
int64_t* rows,
int64_t* cols,
int64_t* ld,
void** values,
cudaDataType* type,
cusparseOrder_t* order)
cusparseStatus_t
cusparseConstDnMatGet(cusparseDnMatDescr_t dnMatDescr, //const descriptor
int64_t* rows,
int64_t* cols,
int64_t* ld,
void** values,
cudaDataType* type,
cusparseOrder_t* order)
This function returns the fields of the dense matrix descriptor dnMatDescr
.
Param. |
Memory |
In/out |
Meaning |
---|---|---|---|
|
HOST |
IN |
Dense matrix descriptor |
|
HOST |
OUT |
Number of rows of the dense matrix |
|
HOST |
OUT |
Number of columns of the dense matrix |
|
HOST |
OUT |
Leading dimension of the dense matrix |
|
DEVICE |
OUT |
Values of the dense matrix. Array of size |
|
HOST |
OUT |
Enumerator specifying the datatype of |
|
HOST |
OUT |
Enumerator specifying the memory layout of the dense matrix |
See cusparseStatus_t for the description of the return status.
14.5.4. cusparseDnMatGetValues()
cusparseStatus_t
cusparseDnMatGetValues(cusparseDnMatDescr_t dnMatDescr,
void** values)
cusparseStatus_t
cusparseConstDnMatGetValues(cusparseDnMatDescr_t dnMatDescr, //const descriptor
void** values)
This function returns the values
field of the dense matrix descriptor dnMatDescr
.
Param. |
Memory |
In/out |
Meaning |
---|---|---|---|
|
HOST |
IN |
Dense matrix descriptor |
|
DEVICE |
OUT |
Values of the dense matrix. Array of size |
See cusparseStatus_t for the description of the return status.
14.5.5. cusparseDnSetValues()
cusparseStatus_t
cusparseDnMatSetValues(cusparseDnMatDescr_t dnMatDescr,
void* values)
This function sets the values
field of the dense matrix descriptor dnMatDescr
.
Param. |
Memory |
In/out |
Meaning |
---|---|---|---|
|
HOST |
IN |
Dense matrix descriptor |
|
DEVICE |
IN |
Values of the dense matrix. Array of size |
See cusparseStatus_t for the description of the return status.
14.5.6. cusparseDnMatGetStridedBatch()
cusparseStatus_t
cusparseDnMatGetStridedBatch(cusparseDnMatDescr_t dnMatDescr, //const descriptor
int* batchCount,
int64_t* batchStride)
The function returns the number of batches and the batch stride of the dense matrix descriptor dnMatDescr
.
Param. |
Memory |
In/out |
Meaning |
---|---|---|---|
|
HOST |
IN |
Dense matrix descriptor |
|
HOST |
OUT |
Number of batches of the dense matrix |
|
HOST |
OUT |
Address offset between a matrix and the next one in the batch |
See cusparseStatus_t for the description of the return status.
14.5.7. cusparseDnMatSetStridedBatch()
cusparseStatus_t
cusparseDnMatSetStridedBatch(cusparseDnMatDescr_t dnMatDescr,
int batchCount,
int64_t batchStride)
The function sets the number of batches and the batch stride of the dense matrix descriptor dnMatDescr
.
Param. |
Memory |
In/out |
Meaning |
---|---|---|---|
|
HOST |
IN |
Dense matrix descriptor |
|
HOST |
IN |
Number of batches of the dense matrix |
|
HOST |
IN |
Address offset between a matrix and the next one in the batch. |
See cusparseStatus_t for the description of the return status.
14.6. Generic API Functions
14.6.1. cusparseSparseToDense()
cusparseStatus_t
cusparseSparseToDense_bufferSize(cusparseHandle_t handle,
cusparseSpMatDescr_t matA, //const descriptor
cusparseDnMatDescr_t matB,
cusparseSparseToDenseAlg_t alg,
size_t* bufferSize)
cusparseStatus_t
cusparseSparseToDense(cusparseHandle_t handle,
cusparseSpMatDescr_t matA, //const descriptor
cusparseDnMatDescr_t matB,
cusparseSparseToDenseAlg_t alg,
void* buffer)
The function converts the sparse matrix matA
in CSR, CSC, or COO format into its dense representation matB
. Blocked-ELL is not currently supported.
The function cusparseSparseToDense_bufferSize()
returns the size of the workspace needed by cusparseSparseToDense()
.
Param. |
Memory |
In/out |
Meaning |
---|---|---|---|
|
HOST |
IN |
Handle to the cuSPARSE library context |
|
HOST |
IN |
Sparse matrix |
|
HOST |
OUT |
Dense matrix |
|
HOST |
IN |
Algorithm for the computation |
|
HOST |
OUT |
Number of bytes of workspace needed by |
|
DEVICE |
IN |
Pointer to workspace buffer |
cusparseSparseToDense()
supports the following index type for representing the sparse matrix matA
:
32-bit indices (
CUSPARSE_INDEX_32I
)64-bit indices (
CUSPARSE_INDEX_64I
)
cusparseSparseToDense()
supports the following data types:
|
---|
|
|
|
|
|
|
|
|
cusparseSparse2Dense()
supports the following algorithm:
Algorithm |
Notes |
---|---|
|
Default algorithm |
cusparseSparseToDense()
has the following properties:
The routine requires no extra storage
The routine supports asynchronous execution
Provides deterministic (bit-wise) results for each run
cusparseSparseToDense()
supports the following optimizations:
CUDA graph capture
Hardware Memory Compression
See cusparseStatus_t for the description of the return status.
Please visit cuSPARSE Library Samples - cusparseSparseToDense for a code example.
14.6.2. cusparseDenseToSparse()
cusparseStatus_t
cusparseDenseToSparse_bufferSize(cusparseHandle_t handle,
cusparseDnMatDescr_t matA, //const descriptor
cusparseSpMatDescr_t matB,
cusparseDenseToSparseAlg_t alg,
size_t* bufferSize)
cusparseStatus_t
cusparseDenseToSparse_analysis(cusparseHandle_t handle,
cusparseDnMatDescr_t matA, //const descriptor
cusparseSpMatDescr_t matB,
cusparseDenseToSparseAlg_t alg,
void* buffer)
cusparseStatus_t
cusparseDenseToSparse_convert(cusparseHandle_t handle,
cusparseDnMatDescr_t matA, //const descriptor
cusparseSpMatDescr_t matB,
cusparseDenseToSparseAlg_t alg,
void* buffer)
The function converts the dense matrix matA
into a sparse matrix matB
in CSR, CSC, COO, or Blocked-ELL format.
The function cusparseDenseToSparse_bufferSize()
returns the size of the workspace needed by cusparseDenseToSparse_analysis()
.
The function cusparseDenseToSparse_analysis()
updates the number of non-zero elements in the sparse matrix descriptor matB
. The user is responsible to allocate the memory required by the sparse matrix:
Row/Column indices and value arrays for CSC and CSR respectively
Row, column, value arrays for COO
Column (
ellColInd
), value (ellValue
) arrays for Blocked-ELL
Finally, we call cusparseDenseToSparse_convert()
for filling the arrays allocated in the previous step.
Param. |
Memory |
In/out |
Meaning |
---|---|---|---|
|
HOST |
IN |
Handle to the cuSPARSE library context |
|
HOST |
IN |
Dense matrix |
|
HOST |
OUT |
Sparse matrix |
|
HOST |
IN |
Algorithm for the computation |
|
HOST |
OUT |
Number of bytes of workspace needed by |
|
DEVICE |
IN |
Pointer to workspace buffer |
cusparseDenseToSparse()
supports the following index type for representing the sparse vector matB
:
32-bit indices (
CUSPARSE_INDEX_32I
)64-bit indices (
CUSPARSE_INDEX_64I
)
cusparseDenseToSparse()
supports the following data types:
|
---|
|
|
|
|
|
|
|
|
cusparseDense2Sparse()
supports the following algorithm:
Algorithm |
Notes |
---|---|
|
Default algorithm |
cusparseDenseToSparse()
has the following properties:
The routine requires no extra storage
The routine supports asynchronous execution
Provides deterministic (bit-wise) results for each run
cusparseDenseToSparse()
supports the following optimizations:
The routine supports does not support CUDA graph capture for CSR, CSC, COO formats
Hardware Memory Compression
See cusparseStatus_t for the description of the return status.
Please visit cuSPARSE Library Samples - cusparseDenseToSparse (CSR) and cuSPARSE Library Samples - cusparseDenseToSparse (Blocked-ELL) for code examples.
14.6.3. cusparseAxpby()
cusparseStatus_t
cusparseAxpby(cusparseHandle_t handle,
const void* alpha,
cusparseSpVecDescr_t vecX, //const descriptor
const void* beta,
cusparseDnVecDescr_t vecY)
The function computes the sum of a sparse vector vecX
and a dense vector vecY
.
\(\mathbf{Y} = \alpha\mathbf{X} + \beta\mathbf{Y}\) |
In other words,
for i=0 to n-1
Y[i] = beta * Y[i]
for i=0 to nnz-1
Y[X_indices[i]] += alpha * X_values[i]
Param. |
Memory |
In/out |
Meaning |
---|---|---|---|
|
HOST |
IN |
Handle to the cuSPARSE library context |
|
HOST or DEVICE |
IN |
\(\alpha\) scalar used for multiplication of compute type |
|
HOST |
IN |
Sparse vector |
|
HOST or DEVICE |
IN |
\(\beta\) scalar used for multiplication of compute type |
|
HOST |
IN/OUT |
Dense vector |
cusparseAxpby
supports the following index type for representing the sparse vector vecX
:
32-bit indices (
CUSPARSE_INDEX_32I
)64-bit indices (
CUSPARSE_INDEX_64I
)
cusparseAxpby
supports the following data types:
Uniform-precision computation:
|
---|
|
|
|
|
Mixed-precision computation:
|
|
---|---|
|
|
|
|
|
|
|
cusparseAxpby()
has the following constraints:
The arrays representing the sparse vector
vecX
must be aligned to 16 bytes
cusparseAxpby()
has the following properties:
The routine requires no extra storage
The routine supports asynchronous execution
Provides deterministic (bit-wise) results for each run if the the sparse vector
vecX
indices are distinct
cusparseAxpby()
supports the following optimizations:
CUDA graph capture
Hardware Memory Compression
See cusparseStatus_t for the description of the return status.
Please visit cuSPARSE Library Samples - cusparseAxpby for a code example.
14.6.4. cusparseGather()
cusparseStatus_t
cusparseGather(cusparseHandle_t handle,
cusparseDnVecDescr_t vecY, //const descriptor
cusparseSpVecDescr_t vecX)
The function gathers the elements of the dense vector vecY
into the sparse vector vecX
In other words,
for i=0 to nnz-1
X_values[i] = Y[X_indices[i]]
Param. |
Memory |
In/out |
Meaning |
---|---|---|---|
|
HOST |
IN |
Handle to the cuSPARSE library context |
|
HOST |
OUT |
Sparse vector |
|
HOST |
IN |
Dense vector |
cusparseGather
supports the following index type for representing the sparse vector vecX
:
32-bit indices (
CUSPARSE_INDEX_32I
)64-bit indices (
CUSPARSE_INDEX_64I
)
cusparseGather
supports the following data types:
|
---|
|
|
|
|
|
|
|
|
cusparseGather()
has the following constraints:
The arrays representing the sparse vector
vecX
must be aligned to 16 bytes
cusparseGather()
has the following properties:
The routine requires no extra storage
The routine supports asynchronous execution
Provides deterministic (bit-wise) results for each run if the the sparse vector
vecX
indices are distinct
cusparseGather()
supports the following optimizations:
CUDA graph capture
Hardware Memory Compression
See cusparseStatus_t for the description of the return status.
Please visit cuSPARSE Library Samples - cusparseGather for a code example.
14.6.5. cusparseScatter()
cusparseStatus_t
cusparseScatter(cusparseHandle_t handle,
cusparseSpVecDescr_t vecX, //const descriptor
cusparseDnVecDescr_t vecY)
The function scatters the elements of the sparse vector vecX
into the dense vector vecY
In other words,
for i=0 to nnz-1
Y[X_indices[i]] = X_values[i]
Param. |
Memory |
In/out |
Meaning |
---|---|---|---|
|
HOST |
IN |
Handle to the cuSPARSE library context |
|
HOST |
IN |
Sparse vector |
|
HOST |
OUT |
Dense vector |
cusparseScatter
supports the following index type for representing the sparse vector vecX
:
32-bit indices (
CUSPARSE_INDEX_32I
)64-bit indices (
CUSPARSE_INDEX_64I
)
cusparseScatter
supports the following data types:
|
---|
|
|
|
|
|
|
|
|
|
cusparseScatter()
has the following constraints:
The arrays representing the sparse vector
vecX
must be aligned to 16 bytes
cusparseScatter()
has the following properties:
The routine requires no extra storage
The routine supports asynchronous execution
Provides deterministic (bit-wise) results for each run if the the sparse vector
vecX
indices are distinct
cusparseScatter()
supports the following optimizations:
CUDA graph capture
Hardware Memory Compression
See cusparseStatus_t for the description of the return status.
Please visit cuSPARSE Library Samples - cusparseScatter for a code example.
14.6.6. cusparseRot()
cusparseStatus_t
cusparseRot(cusparseHandle_t handle,
const void* c_coeff,
const void* s_coeff,
cusparseSpVecDescr_t vecX,
cusparseDnVecDescr_t vecY)
The function computes the Givens rotation matrix
\(G = \begin{bmatrix} c & s \\ {- s} & c \\ \end{bmatrix}\) |
to a sparse vecX
and a dense vector vecY
In other words,
for i=0 to nnz-1
Y[X_indices[i]] = c * Y[X_indices[i]] - s * X_values[i]
X_values[i] = c * X_values[i] + s * Y[X_indices[i]]
Param. |
Memory |
In/out |
Meaning |
---|---|---|---|
|
HOST |
IN |
Handle to the cuSPARSE library context |
|
HOST or DEVICE |
IN |
cosine element of the rotation matrix |
|
HOST |
IN/OUT |
Sparse vector |
|
HOST or DEVICE |
IN |
sine element of the rotation matrix |
|
HOST |
IN/OUT |
Dense vector |
cusparseRot
supports the following index type for representing the sparse vector vecX
:
32-bit indices (
CUSPARSE_INDEX_32I
)64-bit indices (
CUSPARSE_INDEX_64I
)
cusparseRot
supports the following data types:
Uniform-precision computation:
|
---|
|
|
|
|
Mixed-precision computation:
|
|
---|---|
|
|
|
|
|
|
|
cusparseRot()
has the following constraints:
The arrays representing the sparse vector
vecX
must be aligned to 16 bytes
cusparseRot()
has the following properties:
The routine requires no extra storage
The routine supports asynchronous execution
Provides deterministic (bit-wise) results for each run if the the sparse vector
vecX
indices are distinct
cusparseRot()
supports the following optimizations:
CUDA graph capture
Hardware Memory Compression
See cusparseStatus_t for the description of the return status.
Please visit cuSPARSE Library Samples - cusparseRot for a code example.
14.6.7. cusparseSpVV()
cusparseStatus_t
cusparseSpVV_bufferSize(cusparseHandle_t handle,
cusparseOperation_t opX,
cusparseSpVecDescr_t vecX, //const descriptor
cusparseDnVecDescr_t vecY, //const descriptor
void* result,
cudaDataType computeType,
size_t* bufferSize)
cusparseStatus_t
cusparseSpVV(cusparseHandle_t handle,
cusparseOperation_t opX,
cusparseSpVecDescr_t vecX, //const descriptor
cusparseDnVecDescr_t vecY, //const descriptor
void* result,
cudaDataType computeType,
void* externalBuffer)
The function computes the inner dot product of a sparse vector vecX
and a dense vector vecY
\(result = \mathbf{X}' \cdot \mathbf{Y}\) |
In other words,
result = 0;
for i=0 to nnz-1
result += X_values[i] * Y[X_indices[i]]
\(\text{op}(X) = \begin{cases} X & \text{if op(X) == CUSPARSE_OPERATION_NON_TRANSPOSE} \\ \overline{X} & \text{if op(X) == CUSPARSE_OPERATION_CONJUGATE_TRANSPOSE} \\ \end{cases}\)
The function cusparseSpVV_bufferSize()
returns the size of the workspace needed by cusparseSpVV()
Param. |
Memory |
In/out |
Meaning |
---|---|---|---|
|
HOST |
IN |
Handle to the cuSPARSE library context |
|
HOST |
IN |
Operation |
|
HOST |
IN |
Sparse vector |
|
HOST |
IN |
Dense vector |
|
HOST or DEVICE |
OUT |
The resulting dot product |
|
HOST |
IN |
Datatype in which the computation is executed |
|
HOST |
OUT |
Number of bytes of workspace needed by |
|
DEVICE |
IN |
Pointer to a workspace buffer of at least |
cusparseSpVV
supports the following index type for representing the sparse vector vecX
:
32-bit indices (
CUSPARSE_INDEX_32I
)64-bit indices (
CUSPARSE_INDEX_64I
)
The data types combinations currently supported for cusparseSpVV
are listed below:
Uniform-precision computation:
|
---|
|
|
|
|
Mixed-precision computation:
|
|
---|---|
|
|
|
|
|
|
|
|
|
|
|
|
cusparseSpVV()
has the following constraints:
The arrays representing the sparse vector
vecX
must be aligned to 16 bytes
cusparseSpVV()
has the following properties:
The routine requires no extra storage
The routine supports asynchronous execution
Provides deterministic (bit-wise) results for each run if the the sparse vector
vecX
indices are distinct
cusparseSpVV()
supports the following optimizations:
CUDA graph capture
Hardware Memory Compression
See cusparseStatus_t for the description of the return status.
Please visit cuSPARSE Library Samples - cusparseSpVV for a code example.
14.6.8. cusparseSpMV()
cusparseStatus_t
cusparseSpMV_bufferSize(cusparseHandle_t handle,
cusparseOperation_t opA,
const void* alpha,
cusparseSpMatDescr_t matA, //const descriptor
cusparseDnVecDescr_t vecX, //const descriptor
const void* beta,
cusparseDnVecDescr_t vecY,
cudaDataType computeType,
cusparseSpMVAlg_t alg,
size_t* bufferSize)
cusparseStatus_t
cusparseSpMV(cusparseHandle_t handle,
cusparseOperation_t opA,
const void* alpha,
cusparseSpMatDescr_t matA, //const descriptor
cusparseDnVecDescr_t vecX, //const descriptor
const void* beta,
cusparseDnVecDescr_t vecY,
cudaDataType computeType,
cusparseSpMVAlg_t alg,
void* externalBuffer)
This function performs the multiplication of a sparse matrix matA
and a dense vector vecX
\(\mathbf{Y} = \alpha op\left( \mathbf{A} \right) \cdot \mathbf{X} + \beta\mathbf{Y}\) |
where
op(A)
is a sparse matrix of size \(m \times k\)X
is a dense vector of size \(k\)Y
is a dense vector of size \(m\)\(\alpha\) and \(\beta\) are scalars
Also, for matrix A
\(\text{op}(A) == \begin{cases} A & \text{if op(A) == CUSPARSE_OPERATION_NON_TRANSPOSE} \\ A^{T} & \text{if op(A) == CUSPARSE_OPERATION_TRANSPOSE} \\ A^{H} & \text{if op(A) ==CUSPARSE_OPERATION_CONJUGATE_TRANSPOSE} \\ \end{cases}\)
The function cusparseSpMV_bufferSize()
returns the size of the workspace needed by cusparseSpMV()
Param. |
Memory |
In/out |
Meaning |
---|---|---|---|
|
HOST |
IN |
Handle to the cuSPARSE library context |
|
HOST |
IN |
Operation |
|
HOST or DEVICE |
IN |
\(\alpha\) scalar used for multiplication of type |
|
HOST |
IN |
Sparse matrix |
|
HOST |
IN |
Dense vector |
|
HOST or DEVICE |
IN |
\(\beta\) scalar used for multiplication of type |
|
HOST |
IN/OUT |
Dense vector |
|
HOST |
IN |
Datatype in which the computation is executed |
|
HOST |
IN |
Algorithm for the computation |
|
HOST |
OUT |
Number of bytes of workspace needed by |
|
DEVICE |
IN |
Pointer to a workspace buffer of at least |
The sparse matrix formats currently supported are listed below:
CUSPARSE_FORMAT_COO
CUSPARSE_FORMAT_CSR
CUSPARSE_FORMAT_CSC
cusparseSpMV
supports the following index type for representing the sparse matrix matA
:
32-bit indices (
CUSPARSE_INDEX_32I
)64-bit indices (
CUSPARSE_INDEX_64I
)
cusparseSpMV
supports the following data types:
Uniform-precision computation:
|
---|
|
|
|
|
Mixed-precision computation:
|
|
|
---|---|---|
|
|
|
|
|
|
|
||
|
||
|
|
|
|
|
|
|
|
|
|
|
|
|
|
Mixed Regular/Complex computation:
|
|
---|---|
|
|
|
|
NOTE: CUDA_R_16F
, CUDA_R_16BF
, CUDA_C_16F
, and CUDA_C_16BF
data types always imply mixed-precision computation.
cusparseSpMV()
supports the following algorithms:
Algorithm |
Notes |
---|---|
|
Default algorithm for any sparse matrix format. |
|
Default algorithm for COO sparse matrix format. May produce slightly different results during different runs with the same input parameters. |
|
Provides deterministic (bit-wise) results for each run. If |
|
Default algorithm for CSR/CSC sparse matrix format. May produce slightly different results during different runs with the same input parameters. |
|
Provides deterministic (bit-wise) results for each run. If |
Performance notes:
CUSPARSE_SPMV_COO_ALG1
andCUSPARSE_SPMV_CSR_ALG1
provide higher performance thanCUSPARSE_SPMV_COO_ALG2
andCUSPARSE_SPMV_CSR_ALG2
.In general,
opA == CUSPARSE_OPERATION_NON_TRANSPOSE
is 3x faster thanopA != CUSPARSE_OPERATION_NON_TRANSPOSE
.
cusparseSpMV()
has the following properties:
The routine requires extra storage for CSR/CSC format (all algorithms) and for COO format with
CUSPARSE_SPMV_COO_ALG2
algorithm.Provides deterministic (bit-wise) results for each run only for
CUSPARSE_SPMV_COO_ALG2
andCUSPARSE_SPMV_CSR_ALG2
algorithms, andopA == CUSPARSE_OPERATION_NON_TRANSPOSE
.The routine supports asynchronous execution.
compute-sanitizer could report false race conditions for this routine when
beta == 0
. This is for optimization purposes and does not affect the correctness of the computation.
cusparseSpMV()
supports the following optimizations:
CUDA graph capture
Hardware Memory Compression
See cusparseStatus_t for the description of the return status.
Please visit cuSPARSE Library Samples - cusparseSpMV CSR and cusparseSpMV COO for a code example.
14.6.9. cusparseSpSV()
cusparseStatus_t
cusparseSpSV_createDescr(cusparseSpSVDescr_t* spsvDescr);
cusparseStatus_t
cusparseSpSV_destroyDescr(cusparseSpSVDescr_t spsvDescr);
cusparseStatus_t
cusparseSpSV_bufferSize(cusparseHandle_t handle,
cusparseOperation_t opA,
const void* alpha,
cusparseSpMatDescr_t matA, //const descriptor
cusparseDnVecDescr_t vecX, //const descriptor
cusparseDnVecDescr_t vecY,
cudaDataType computeType,
cusparseSpSVAlg_t alg,
cusparseSpSVDescr_t spsvDescr,
size_t* bufferSize)
cusparseStatus_t
cusparseSpSV_analysis(cusparseHandle_t handle,
cusparseOperation_t opA,
const void* alpha,
cusparseSpMatDescr_t matA, //const descriptor
cusparseDnVecDescr_t vecX, //const descriptor
cusparseDnVecDescr_t vecY,
cudaDataType computeType,
cusparseSpSVAlg_t alg,
cusparseSpSVDescr_t spsvDescr
void* externalBuffer)
cusparseStatus_t
cusparseSpSV_solve(cusparseHandle_t handle,
cusparseOperation_t opA,
const void* alpha,
cusparseSpMatDescr_t matA, //const descriptor
cusparseDnVecDescr_t vecX, //const descriptor
cusparseDnVecDescr_t vecY,
cudaDataType computeType,
cusparseSpSVAlg_t alg,
cusparseSpSVDescr_t spsvDescr)
The function solves a system of linear equations whose coefficients are represented in a sparse triangular matrix:
\(op\left( \mathbf{A} \right) \cdot \mathbf{Y} = \alpha\mathbf{X}\) |
where
op(A)
is a sparse square matrix of size \(m \times m\)X
is a dense vector of size \(m\)Y
is a dense vector of size \(m\)\(\alpha\) is a scalar
Also, for matrix A
\(\text{op}(A) = \begin{cases} A & \text{if op(A) == CUSPARSE_OPERATION_NON_TRANSPOSE} \\ A^{T} & \text{if op(A) == CUSPARSE_OPERATION_TRANSPOSE} \\ A^{H} & \text{if op(A) == CUSPARSE_OPERATION_CONJUGATE_TRANSPOSE} \\ \end{cases}\)
The function cusparseSpSV_bufferSize()
returns the size of the workspace needed by cusparseSpSV_analysis()
and cusparseSpSV_solve()
. The function cusparseSpSV_analysis()
performs the analysis phase, while cusparseSpSV_solve()
executes the solve phase for a sparse triangular linear system. The opaque data structure spsvDescr
is used to share information among all functions.
The routine supports arbitrary sparsity for the input matrix, but only the upper or lower triangular part is taken into account in the computation.
NOTE: all parameters must be consistent across cusparseSpSV
API calls and the matrix descriptions must not be modified between cusparseSpSV_analysis()
and cusparseSpSV_solve()
Param. |
Memory |
In/out |
Meaning |
---|---|---|---|
|
HOST |
IN |
Handle to the cuSPARSE library context |
|
HOST |
IN |
Operation |
|
HOST or DEVICE |
IN |
\(\alpha\) scalar used for multiplication of type |
|
HOST |
IN |
Sparse matrix |
|
HOST |
IN |
Dense vector |
|
HOST |
IN/OUT |
Dense vector |
|
HOST |
IN |
Datatype in which the computation is executed |
|
HOST |
IN |
Algorithm for the computation |
|
HOST |
OUT |
Number of bytes of workspace needed by |
|
DEVICE |
IN |
Pointer to a workspace buffer of at least |
|
HOST |
IN/OUT |
Opaque descriptor for storing internal data used across the three steps |
The sparse matrix formats currently supported are listed below:
CUSPARSE_FORMAT_CSR
CUSPARSE_FORMAT_COO
The cusparseSpSV()
supports the following shapes and properties:
CUSPARSE_FILL_MODE_LOWER
andCUSPARSE_FILL_MODE_UPPER
fill modesCUSPARSE_DIAG_TYPE_NON_UNIT
andCUSPARSE_DIAG_TYPE_UNIT
diagonal types
The fill mode and diagonal type can be set by cusparseSpMatSetAttribute()
cusparseSpSV()
supports the following index type for representing the sparse matrix matA
:
32-bit indices (
CUSPARSE_INDEX_32I
)64-bit indices (
CUSPARSE_INDEX_64I
)
cusparseSpSV()
supports the following data types:
Uniform-precision computation:
|
---|
|
|
|
|
cusparseSpSV()
supports the following algorithms:
Algorithm |
Notes |
---|---|
|
Default algorithm |
cusparseSpSV()
has the following properties:
The routine requires extra storage for the analysis phase which is proportional to number of non-zero entries of the sparse matrix
Provides deterministic (bit-wise) results for each run for the solving phase
cusparseSpSV_solve()
The routine supports asynchronous execution
cusparseSpSV()
supports the following optimizations:
CUDA graph capture
Hardware Memory Compression
See cusparseStatus_t for the description of the return status.
Please visit cuSPARSE Library Samples - cusparseSpSV CSR and cuSPARSE Library Samples - cusparseSpSV COO for code examples.
14.6.10. cusparseSpMM()
cusparseStatus_t
cusparseSpMM_bufferSize(cusparseHandle_t handle,
cusparseOperation_t opA,
cusparseOperation_t opB,
const void* alpha,
cusparseSpMatDescr_t matA, //const descriptor
cusparseDnMatDescr_t matB, //const descriptor
const void* beta,
cusparseDnMatDescr_t matC,
cudaDataType computeType,
cusparseSpMMAlg_t alg,
size_t* bufferSize)
cusparseStatus_t
cusparseSpMM_preprocess(cusparseHandle_t handle,
cusparseOperation_t opA,
cusparseOperation_t opB,
const void* alpha,
cusparseSpMatDescr_t matA, //const descriptor
cusparseDnMatDescr_t matB, //const descriptor
const void* beta,
cusparseDnMatDescr_t matC,
cudaDataType computeType,
cusparseSpMMAlg_t alg,
void* externalBuffer)
cusparseStatus_t
cusparseSpMM(cusparseHandle_t handle,
cusparseOperation_t opA,
cusparseOperation_t opB,
const void* alpha,
cusparseSpMatDescr_t matA, //const descriptor
cusparseDnMatDescr_t matB, //const descriptor
const void* beta,
cusparseDnMatDescr_t matC,
cudaDataType computeType,
cusparseSpMMAlg_t alg,
void* externalBuffer)
The function performs the multiplication of a sparse matrix matA
and a dense matrix matB
.
\(\mathbf{C} = \alpha op\left( \mathbf{A} \right) \cdot op\left( \mathbf{B} \right) + \beta\mathbf{C}\) |
where
op(A)
is a sparse matrix of size \(m \times k\)op(B)
is a dense matrix of size \(k \times n\)C
is a dense matrix of size \(m \times n\)\(\alpha\) and \(\beta\) are scalars
The routine can be also used to perform the multiplication of a dense matrix and a sparse matrix by switching the dense matrices layout:
\(\begin{array}{l} \left. \mathbf{C}_{C} = \mathbf{B}_{C} \cdot \mathbf{A} + \beta\mathbf{C}_{C}\rightarrow \right. \\ {\mathbf{C}_{R} = \mathbf{A}^{T} \cdot \mathbf{B}_{R} + \beta\mathbf{C}_{R}} \\ \end{array}\) |
where \(\mathbf{B}_{C}\) , \(\mathbf{C}_{C}\) indicate column-major layout, while \(\mathbf{B}_{R}\) , \(\mathbf{C}_{R}\) refer to row-major layout
Also, for matrix A
and B
\(\text{op}(A) = \begin{cases} A & \text{if op(A) == CUSPARSE_OPERATION_NON_TRANSPOSE} \\ A^{T} & \text{if op(A) == CUSPARSE_OPERATION_TRANSPOSE} \\ A^{H} & \text{if op(A) == CUSPARSE_OPERATION_CONJUGATE_TRANSPOSE} \\ \end{cases}\)
\(\text{op}(B) = \begin{cases} B & \text{if op(B) == CUSPARSE_OPERATION_NON_TRANSPOSE} \\ B^{T} & \text{if op(B) == CUSPARSE_OPERATION_TRANSPOSE} \\ B^{H} & \text{if op(B) == CUSPARSE_OPERATION_CONJUGATE_TRANSPOSE} \\ \end{cases}\)
When using the (conjugate) transpose of the sparse matrix A
, this routine may produce slightly different results during different runs with the same input parameters.
The function cusparseSpMM_bufferSize()
returns the size of the workspace needed by cusparseSpMM()
The function cusparseSpMM_preprocess()
can be called before cusparseSpMM
to speedup the actual computation. It is useful when cusparseSpMM
is called multiple times with the same sparsity pattern (matA
). The values of the matrices (matA
, matB
, matC
) can change arbitrarily. It provides performance advantages is used with CUSPARSE_SPMM_CSR_ALG1
or CUSPARSE_SPMM_CSR_ALG3
. For all other formats and algorithms have no effect.
Param. |
Memory |
In/out |
Meaning |
---|---|---|---|
|
HOST |
IN |
Handle to the cuSPARSE library context |
|
HOST |
IN |
Operation |
|
HOST |
IN |
Operation |
|
HOST or DEVICE |
IN |
\(\alpha\) scalar used for multiplication of type |
|
HOST |
IN |
Sparse matrix |
|
HOST |
IN |
Dense matrix |
|
HOST or DEVICE |
IN |
\(\beta\) scalar used for multiplication of type |
|
HOST |
IN/OUT |
Dense matrix |
|
HOST |
IN |
Datatype in which the computation is executed |
|
HOST |
IN |
Algorithm for the computation |
|
HOST |
OUT |
Number of bytes of workspace needed by |
|
DEVICE |
IN |
Pointer to workspace buffer of at least |
cusparseSpMM
supports the following sparse matrix formats:
CUSPARSE_FORMAT_COO
CUSPARSE_FORMAT_CSR
CUSPARSE_FORMAT_CSC
CUSPARSE_FORMAT_BLOCKED_ELL
(1) |
COO/CSR/CSC FORMATS |
cusparseSpMM
supports the following index type for representing the sparse matrix matA
:
32-bit indices (
CUSPARSE_INDEX_32I
)64-bit indices (
CUSPARSE_INDEX_64I
)
cusparseSpMM
supports the following data types:
Uniform-precision computation:
|
---|
|
|
|
|
Mixed-precision computation:
|
|
|
---|---|---|
|
|
|
|
|
|
|
||
|
||
|
|
|
|
|
|
|
|
|
|
|
NOTE: CUDA_R_16F
, CUDA_R_16BF
, CUDA_C_16F
, and CUDA_C_16BF
data types always imply mixed-precision computation.
cusparseSpMM
supports the following algorithms:
Algorithm |
Notes |
---|---|
|
Default algorithm for any sparse matrix format |
|
Algorithm 1 for COO sparse matrix format
|
|
Algorithm 2 for COO sparse matrix format
|
|
Algorithm 3 for COO sparse matrix format
|
|
Algorithm 4 for COO sparse matrix format
|
|
Algorithm 1 for CSR/CSC sparse matrix format
|
|
Algorithm 2 for CSR/CSC sparse matrix format
|
|
Algorithm 3 for CSR/CSC sparse matrix format
|
Performance notes:
Row-major layout provides higher performance than column-major
CUSPARSE_SPMM_COO_ALG4
andCUSPARSE_SPMM_CSR_ALG2
should be used with row-major layout, whileCUSPARSE_SPMM_COO_ALG1
,CUSPARSE_SPMM_COO_ALG2
,CUSPARSE_SPMM_COO_ALG3
, andCUSPARSE_SPMM_CSR_ALG1
with column-major layoutFor
beta != 1
, the output matrix is scaled before the actual computationFor
n == 1
, the routine usescusparseSpMV()
as fallback
cusparseSpMM()
with all algorithms support the following batch modes except for CUSPARSE_SPMM_CSR_ALG3
:
\(C_{i} = A \cdot B_{i}\)
\(C_{i} = A_{i} \cdot B\)
\(C_{i} = A_{i} \cdot B_{i}\)
The number of batches and their strides can be set by using cusparseCooSetStridedBatch
, cusparseCsrSetStridedBatch
, and cusparseDnMatSetStridedBatch
.
cusparseSpMM()
has the following properties:
The routine requires no extra storage for
CUSPARSE_SPMM_COO_ALG1
,CUSPARSE_SPMM_COO_ALG3
,CUSPARSE_SPMM_COO_ALG4
The routine supports asynchronous execution
Provides deterministic (bit-wise) results for each run only for
CUSPARSE_SPMM_COO_ALG2
andCUSPARSE_SPMM_CSR_ALG3
algorithmscompute-sanitizer
could report false race conditions for this routine. This is for optimization purposes and does not affect the correctness of the computation.
cusparseSpMM()
supports the following optimizations:
CUDA graph capture
Hardware Memory Compression
Please visit cuSPARSE Library Samples - cusparseSpMM CSR and cusparseSpMM COO for a code example. For batched computation please visit cusparseSpMM CSR Batched and cusparseSpMM COO Batched.
(2) |
BLOCKED-ELLPACK FORMAT |
cusparseSpMM
supports the following data types for CUSPARSE_FORMAT_BLOCKED_ELL
format and the following GPU architectures for exploiting NVIDIA Tensor Cores:
|
|
|
|
|
---|---|---|---|---|
|
|
|
|
|
|
|
|
|
|
|
|
|
|
|
|
|
|
|
|
|
|
|
|
|
|
|
|
|
|
|
|
|
|
|
|
|
|
|
|
cusparseSpMM
supports the following algorithms with CUSPARSE_FORMAT_BLOCKED_ELL
format:
Algorithm |
Notes |
---|---|
|
Default algorithm for any sparse matrix format |
|
Default algorithm for Blocked-ELL format |
Performance notes:
Blocked-ELL SpMM provides the best performance with Power-of-2 Block-Sizes.
Large Block-Sizes (e.g. ≥ 64) provide the best performance.
The function has the following limitations:
The pointer mode must be equal to
CUSPARSE_POINTER_MODE_HOST
Only
opA == CUSPARSE_OPERATION_NON_TRANSPOSE
is supported.opB == CUSPARSE_OPERATION_CONJUGATE_TRANSPOSE
is not supported.
Please visit cuSPARSE Library Samples - cusparseSpMM Blocked-ELL for a code example.
See cusparseStatus_t for the description of the return status.
14.6.11. cusparseSpMMOp()
cusparseStatus_t CUSPARSEAPI
cusparseSpMMOp_createPlan(cusparseHandle_t handle,
cusparseSpMMOpPlan_t* plan,
cusparseOperation_t opA,
cusparseOperation_t opB,
cusparseSpMatDescr_t matA, //const descriptor
cusparseDnMatDescr_t matB, //const descriptor
cusparseDnMatDescr_t matC,
cudaDataType computeType,
cusparseSpMMOpAlg_t alg,
const void* addOperationNvvmBuffer,
size_t addOperationBufferSize,
const void* mulOperationNvvmBuffer,
size_t mulOperationBufferSize,
const void* epilogueNvvmBuffer,
size_t epilogueBufferSize,
size_t* SpMMWorkspaceSize)
cusparseStatus_t
cusparseSpMMOp_destroyPlan(cusparseSpMMOpPlan_t plan)
cusparseStatus_t
cusparseSpMMOp(cusparseSpMMOpPlan_t plan,
void* externalBuffer)
NOTE 1: The routine requires CUDA driver 495.XX or later (CUDA 11.5).
NOTE 2: NVRTC and nvJitLink are not currently available on Arm64 Android platforms.
NOTE 3: The routine does not support Android and Tegra platforms except Judy (sm87).
Experimental: The function performs the multiplication of a sparse matrix matA
and a dense matrix matB
with custom operators.
\({C^{\prime}}_{ij} = \text{epilogue}\left( {\sum_{k}^{\oplus}{op\left( A_{ik} \right) \otimes op\left( B_{kj} \right),C_{ij}}} \right)\) |
where
op(A)
is a sparse matrix of size \(m \times k\)op(B)
is a dense matrix of size \(k \times n\)C
is a dense matrix of size \(m \times n\)\(\oplus\) , \(\otimes\) , and \(\text{epilogue}\) are custom add, mul, and epilogue operators respectively.
Also, for matrix A
and B
\(\text{op}(A) = \begin{cases} A & \text{if op(A) == CUSPARSE_OPERATION_NON_TRANSPOSE} \\ A^{T} & \text{if op(A) == CUSPARSE_OPERATION_TRANSPOSE} \\ \end{cases}\)
\(\text{op}(B) = \begin{cases} B & {\text{if op(}B\text{) == CUSPARSE_OPERATION_NON_TRANSPOSE}} \\ B^{T} & {\text{if op(}B\text{) == CUSPARSE_OPERATION_TRANSPOSE}} \\ \end{cases}\)
Only opA == CUSPARSE_OPERATION_NON_TRANSPOSE
and opB == CUSPARSE_OPERATION_NON_TRANSPOSE
is currently supported
The function cusparseSpMMOp_createPlan()
returns the size of the workspace and the compiled kernel needed by cusparseSpMMOp()
Param. |
Memory |
In/out |
Meaning |
---|---|---|---|
|
HOST |
IN |
Handle to the cuSPARSE library context |
|
HOST |
IN |
Operation |
|
HOST |
IN |
Operation |
|
HOST |
IN |
Sparse matrix |
|
HOST |
IN |
Dense matrix |
|
HOST |
IN/OUT |
Dense matrix |
|
HOST |
IN |
Datatype in which the computation is executed |
|
HOST |
IN |
Algorithm for the computation |
|
HOST |
IN |
Pointer to the NVVM buffer containing the custom add operator |
|
HOST |
IN |
Size in bytes of |
|
HOST |
IN |
Pointer to the NVVM buffer containing the custom mul operator |
|
HOST |
IN |
Size in bytes of |
|
HOST |
IN |
Pointer to the NVVM buffer containing the custom epilogue operator |
|
HOST |
IN |
Size in bytes of |
|
HOST |
OUT |
Number of bytes of workspace needed by |
The operators must have the following signature and return type
__device__ <computetype> add_op(<computetype> value1, <computetype> value2);
__device__ <computetype> mul_op(<computetype> value1, <computetype> value2);
__device__ <computetype> epilogue(<computetype> value1, <computetype> value2);
<computetype>
is one of float
, double
, cuComplex
, cuDoubleComplex
, or int
,
cusparseSpMMOp
supports the following sparse matrix formats:
CUSPARSE_FORMAT_CSR
cusparseSpMMOp
supports the following index type for representing the sparse matrix matA
:
32-bit indices (
CUSPARSE_INDEX_32I
)64-bit indices (
CUSPARSE_INDEX_64I
)
cusparseSpMMOp
supports the following data types:
Uniform-precision computation:
|
---|
|
|
|
|
Mixed-precision computation:
|
|
|
---|---|---|
|
|
|
|
|
|
|
||
|
||
|
|
|
|
|
cusparseSpMMOp
supports the following algorithms:
Algorithm |
Notes |
---|---|
|
Default algorithm for any sparse matrix format |
Performance notes:
Row-major layout provides higher performance than column-major.
cusparseSpMMOp()
has the following properties:
The routine requires extra storage
The routine supports asynchronous execution
Provides deterministic (bit-wise) results for each run
cusparseSpMMOp()
supports the following optimizations:
CUDA graph capture
Hardware Memory Compression
Please visit cuSPARSE Library Samples - cusparseSpMMOp
See cusparseStatus_t for the description of the return status.
14.6.12. cusparseSpSM()
cusparseStatus_t
cusparseSpSM_createDescr(cusparseSpSMDescr_t* spsmDescr);
cusparseStatus_t
cusparseSpSM_destroyDescr(cusparseSpSMDescr_t spsmDescr);
cusparseStatus_t
cusparseSpSM_bufferSize(cusparseHandle_t handle,
cusparseOperation_t opA,
cusparseOperation_t opB,
const void* alpha,
cusparseSpMatDescr_t matA, //const descriptor
cusparseDnMatDescr_t matB, //const descriptor
cusparseDnMatDescr_t matC,
cudaDataType computeType,
cusparseSpSMAlg_t alg,
cusparseSpSMDescr_t spsmDescr,
size_t* bufferSize)
cusparseStatus_t
cusparseSpSM_analysis(cusparseHandle_t handle,
cusparseOperation_t opA,
cusparseOperation_t opB,
const void* alpha,
cusparseSpMatDescr_t matA, //const descriptor
cusparseDnMatDescr_t matB, //const descriptor
cusparseDnMatDescr_t matC,
cudaDataType computeType,
cusparseSpSMAlg_t alg,
cusparseSpSMDescr_t spsmDescr,
void* externalBuffer)
cusparseStatus_t
cusparseSpSM_solve(cusparseHandle_t handle,
cusparseOperation_t opA,
cusparseOperation_t opB,
const void* alpha,
cusparseSpMatDescr_t matA, //const descriptor
cusparseDnMatDescr_t matB, //const descriptor
cusparseDnMatDescr_t matC,
cudaDataType computeType,
cusparseSpSMAlg_t alg,
cusparseSpSMDescr_t spsmDescr)
The function solves a system of linear equations whose coefficients are represented in a sparse triangular matrix:
\(op\left( \mathbf{A} \right) \cdot \mathbf{C} = \mathbf{\alpha}op\left( \mathbf{B} \right)\) |
where
op(A)
is a sparse square matrix of size \(m \times m\)op(B)
is a dense matrix of size \(m \times n\)C
is a dense matrix of size \(m \times n\)\(\alpha\) is a scalar
Also, for matrix A
\(\text{op}(A) = \begin{cases} A & \text{if op(A) == CUSPARSE_OPERATION_NON_TRANSPOSE} \\ A^{T} & \text{if op(A) == CUSPARSE_OPERATION_TRANSPOSE} \\ A^{H} & \text{if op(A) == CUSPARSE_OPERATION_CONJUGATE_TRANSPOSE} \\ \end{cases}\)
\(\text{op}(B) = \begin{cases} B & \text{if op(B) == CUSPARSE_OPERATION_NON_TRANSPOSE} \\ B^{T} & \text{if op(B) == CUSPARSE_OPERATION_TRANSPOSE} \\ & \text{ } \\ \end{cases}\)
The function cusparseSpSM_bufferSize()
returns the size of the workspace needed by cusparseSpSM_analysis()
and cusparseSpSM_solve()
. The function cusparseSpSM_analysis()
performs the analysis phase, while cusparseSpSM_solve()
executes the solve phase for a sparse triangular linear system. The opaque data structure spsmDescr
is used to share information among all functions.
The routine supports arbitrary sparsity for the input matrix, but only the upper or lower triangular part is taken into account in the computation.
cusparseSpSM_bufferSize()
requires a buffer size for the analysis phase which is proportional to number of non-zero entries of the sparse matrix
The externalBuffer
is stored into spsmDescr
and used by cusparseSpSM_solve()
. For this reason, the device memory buffer must be deallocated only after cusparseSpSM_solve()
NOTE: all parameters must be consistent across cusparseSpSM
API calls and the matrix descriptions must not be modified between cusparseSpSM_analysis()
and cusparseSpSM_solve()
Param. |
Memory |
In/out |
Meaning |
---|---|---|---|
|
HOST |
IN |
Handle to the cuSPARSE library context |
|
HOST |
IN |
Operation |
|
HOST |
IN |
Operation |
|
HOST or DEVICE |
IN |
\(\alpha\) scalar used for multiplication of type |
|
HOST |
IN |
Sparse matrix |
|
HOST |
IN |
Dense matrix |
|
HOST |
IN/OUT |
Dense matrix |
|
HOST |
IN |
Datatype in which the computation is executed |
|
HOST |
IN |
Algorithm for the computation |
|
HOST |
OUT |
Number of bytes of workspace needed by |
|
DEVICE |
IN |
Pointer to a workspace buffer of at least |
|
HOST |
IN/OUT |
Opaque descriptor for storing internal data used across the three steps |
The sparse matrix formats currently supported are listed below:
CUSPARSE_FORMAT_CSR
CUSPARSE_FORMAT_COO
The cusparseSpSM()
supports the following shapes and properties:
CUSPARSE_FILL_MODE_LOWER
andCUSPARSE_FILL_MODE_UPPER
fill modesCUSPARSE_DIAG_TYPE_NON_UNIT
andCUSPARSE_DIAG_TYPE_UNIT
diagonal types
The fill mode and diagonal type can be set by cusparseSpMatSetAttribute()
cusparseSpSM()
supports the following index type for representing the sparse matrix matA
:
32-bit indices (
CUSPARSE_INDEX_32I
)64-bit indices (
CUSPARSE_INDEX_64I
)
cusparseSpSM()
supports the following data types:
Uniform-precision computation:
|
---|
|
|
|
|
cusparseSpSM()
supports the following algorithms:
Algorithm |
Notes |
---|---|
|
Default algorithm |
cusparseSpSM()
has the following properties:
The routine requires no extra storage
Provides deterministic (bit-wise) results for each run for the solving phase
cusparseSpSM_solve()
The routine supports asynchronous execution
cusparseSpSM()
supports the following optimizations:
CUDA graph capture
Hardware Memory Compression
See cusparseStatus_t for the description of the return status.
Please visit cuSPARSE Library Samples - cusparseSpSM CSR and cuSPARSE Library Samples - cusparseSpSM COO for code examples.
14.6.13. cusparseSDDMM()
cusparseStatus_t
cusparseSDDMM_bufferSize(cusparseHandle_t handle,
cusparseOperation_t opA,
cusparseOperation_t opB,
const void* alpha,
cusparseDnMatDescr_t matA, //const descriptor
cusparseDnMatDescr_t matB, //const descriptor
const void* beta,
cusparseSpMatDescr_t matC,
cudaDataType computeType,
cusparseSDDMMAlg_t alg,
size_t* bufferSize)
cusparseStatus_t
cusparseSDDMM_preprocess(cusparseHandle_t handle,
cusparseOperation_t opA,
cusparseOperation_t opB,
const void* alpha,
cusparseDnMatDescr_t matA, //const descriptor
cusparseDnMatDescr_t matB, //const descriptor
const void* beta,
cusparseSpMatDescr_t matC,
cudaDataType computeType,
cusparseSDDMMAlg_t alg,
void* externalBuffer)
cusparseStatus_t
cusparseSDDMM(cusparseHandle_t handle,
cusparseOperation_t opA,
cusparseOperation_t opB,
const void* alpha,
cusparseDnMatDescr_t matA, //const descriptor
cusparseDnMatDescr_t matB, //const descriptor
const void* beta,
cusparseSpMatDescr_t matC,
cudaDataType computeType,
cusparseSDDMMAlg_t alg,
void* externalBuffer)
This function performs the multiplication of matA
and matB
, followed by an element-wise multiplication with the sparsity pattern of matC
. Formally, it performs the following operation:
\(\mathbf{C} = \alpha({op}(\mathbf{A}) \cdot {op}(\mathbf{B})) \circ {spy}(\mathbf{C}) + \beta\mathbf{C}\) |
where
op(A)
is a dense matrix of size \(m \times k\)op(B)
is a dense matrix of size \(k \times n\)C
is a sparse matrix of size \(m \times n\)\(\alpha\) and \(\beta\) are scalars
\(\circ\) denotes the Hadamard (entry-wise) matrix product, and \({spy}\left( \mathbf{C} \right)\) is the sparsity pattern matrix of
C
defined as:\({spy}(\mathbf{C})_{ij} = \left\{ \begin{matrix} 0 & {\text{if}\,\mathbf{C}_{ij} = 0} \\ 1 & \text{otherwise} \\ \end{matrix} \right.\)
Also, for matrix A
and B
\(\text{op}(A) = \begin{cases} A & \text{if op(A) == CUSPARSE_OPERATION_NON_TRANSPOSE} \\ A^{T} & \text{if op(A) == CUSPARSE_OPERATION_TRANSPOSE} \\ A^{H} & \text{if op(A) == CUSPARSE_OPERATION_CONJUGATE_TRANSPOSE} \\ \end{cases}\)
\(\text{op}(B) = \begin{cases} B & \text{if op(B) == CUSPARSE_OPERATION_NON_TRANSPOSE} \\ B^{T} & \text{if op(B) == CUSPARSE_OPERATION_TRANSPOSE} \\ B^{H} & \text{if op(B) == CUSPARSE_OPERATION_CONJUGATE_TRANSPOSE} \\ \end{cases}\)
CUSPARSE_OPERATION_CONJUGATE_TRANSPOSE
is currently not supported.
The function cusparseSDDMM_bufferSize()
returns the size of the workspace needed by cusparseSDDMM
or cusparseSDDMM_preprocess
.
The function cusparseSDDMM_preprocess()
can be called before cusparseSDDMM
to speedup the actual computation. It is useful when cusparseSDDMM
is called multiple times with the same sparsity pattern (matC
). The values of the dense matrices (matA
, matB
) can change arbitrarily.
Param. |
Memory |
In/out |
Meaning |
---|---|---|---|
|
HOST |
IN |
Handle to the cuSPARSE library context |
|
HOST |
IN |
Operation |
|
HOST |
IN |
Operation |
|
HOST or DEVICE |
IN |
\(\alpha\) scalar used for multiplication of type |
|
HOST |
IN |
Dense matrix |
|
HOST |
IN |
Dense matrix |
|
HOST or DEVICE |
IN |
\(\beta\) scalar used for multiplication of type |
|
HOST |
IN/OUT |
Sparse matrix |
|
HOST |
IN |
Datatype in which the computation is executed |
|
HOST |
IN |
Algorithm for the computation |
|
HOST |
OUT |
Number of bytes of workspace needed by |
|
DEVICE |
IN |
Pointer to a workspace buffer of at least |
Currently supported sparse matrix formats:
CUSPARSE_FORMAT_CSR
cusparseSDDMM()
supports the following index type for representing the sparse matrix matA
:
32-bit indices (
CUSPARSE_INDEX_32I
)64-bit indices (
CUSPARSE_INDEX_64I
)
The data types combinations currently supported for cusparseSDDMM
are listed below:
Uniform-precision computation:
|
---|
|
|
|
|
cusparseSDDMM()
supports the following algorithms:
Algorithm |
Notes |
---|---|
|
Default algorithm. It supports batched computation. |
Performance notes: cuspaseSDDMM()
provides the best performance when matA
and matB
satisfy:
matA
:matA
is in row-major order andopA
isCUSPARSE_OPERATION_NON_TRANSPOSE
, ormatA
is in col-major order andopA
is notCUSPARSE_OPERATION_NON_TRANSPOSE
matA
:matB
is in col-major order and opB isCUSPARSE_OPERATION_NON_TRANSPOSE
, ormatB
is in row-major order and opB is notCUSPARSE_OPERATION_NON_TRANSPOSE
cusparseSDDMM()
supports the following batch modes:
\(C_{i} = (A \cdot B) \circ C_{i}\)
\(C_{i} = \left( A_{i} \cdot B \right) \circ C_{i}\)
\(C_{i} = \left( A \cdot B_{i} \right) \circ C_{i}\)
\(C_{i} = \left( A_{i} \cdot B_{i} \right) \circ C_{i}\)
The number of batches and their strides can be set by using cusparseCsrSetStridedBatch
and cusparseDnMatSetStridedBatch
.
cusparseSDDMM()
has the following properties:
The routine requires no extra storage
Provides deterministic (bit-wise) results for each run
The routine supports asynchronous execution
cusparseSDDMM()
supports the following optimizations:
CUDA graph capture
Hardware Memory Compression
See cusparseStatus_t for the description of the return status.
Please visit cuSPARSE Library Samples - cusparseSDDMM for a code example. For batched computation please visit cusparseSDDMM CSR Batched.
14.6.14. cusparseSpGEMM()
cusparseStatus_t
cusparseSpGEMM_createDescr(cusparseSpGEMMDescr_t* descr)
cusparseStatus_t
cusparseSpGEMM_destroyDescr(cusparseSpGEMMDescr_t descr)
cusparseStatus_t
cusparseSpGEMM_workEstimation(cusparseHandle_t handle,
cusparseOperation_t opA,
cusparseOperation_t opB,
const void* alpha,
cusparseSpMatDescr_t matA, //const descriptor
cusparseSpMatDescr_t matB, //const descriptor
const void* beta,
cusparseSpMatDescr_t matC,
cudaDataType computeType,
cusparseSpGEMMAlg_t alg,
cusparseSpGEMMDescr_t spgemmDescr,
size_t* bufferSize1,
void* externalBuffer1)
cusparseStatus_t
cusparseSpGEMM_getNumProducts(cusparseSpGEMMDescr_t spgemmDescr,
int64_t* num_prods)
cusparseStatus_t
cusparseSpGEMM_estimateMemory(cusparseHandle_t handle,
cusparseOperation_t opA,
cusparseOperation_t opB,
const void* alpha,
cusparseConstSpMatDescr_t matA, //const descriptor
cusparseConstSpMatDescr_t matB, //const descriptor
const void* beta,
cusparseSpMatDescr_t matC,
cudaDataType computeType,
cusparseSpGEMMAlg_t alg,
cusparseSpGEMMDescr_t spgemmDescr,
float chunk_fraction,
size_t* bufferSize3,
void* externalBuffer3,
size_t* bufferSize2)
cusparseStatus_t
cusparseSpGEMM_compute(cusparseHandle_t handle,
cusparseOperation_t opA,
cusparseOperation_t opB,
const void* alpha,
cusparseSpMatDescr_t matA, //const descriptor
cusparseSpMatDescr_t matB, //const descriptor
const void* beta,
cusparseSpMatDescr_t matC,
cudaDataType computeType,
cusparseSpGEMMAlg_t alg,
cusparseSpGEMMDescr_t spgemmDescr,
size_t* bufferSize2,
void* externalBuffer2)
cusparseStatus_t
cusparseSpGEMM_copy(cusparseHandle_t handle,
cusparseOperation_t opA,
cusparseOperation_t opB,
const void* alpha,
cusparseSpMatDescr_t matA, //const descriptor
cusparseSpMatDescr_t matB, //const descriptor
const void* beta,
cusparseSpMatDescr_t matC,
cudaDataType computeType,
cusparseSpGEMMAlg_t alg,
cusparseSpGEMMDescr_t spgemmDescr)
This function performs the multiplication of two sparse matrices matA
and matB
.
\(\mathbf{C^{\prime}} = \alpha op\left( \mathbf{A} \right) \cdot op\left( \mathbf{B} \right) + \beta\mathbf{C}\) |
where \(\alpha,\) \(\beta\) are scalars, and \(\mathbf{C},\) \(\mathbf{C^{\prime}}\) have the same sparsity pattern.
The functions cusparseSpGEMM_workEstimation()
, cusparseSpGEMM_estimateMemory()
, and cusparseSpGEMM_compute()
are used for both determining the buffer size and performing the actual computation.
Param. |
Memory |
In/out |
Meaning |
---|---|---|---|
|
HOST |
IN |
Handle to the cuSPARSE library context |
|
HOST |
IN |
Operation |
|
HOST |
IN |
Operation |
|
HOST or DEVICE |
IN |
\(\alpha\) scalar used for multiplication |
|
HOST |
IN |
Sparse matrix |
|
HOST |
IN |
Sparse matrix |
|
HOST or DEVICE |
IN |
\(\beta\) scalar used for multiplication |
|
HOST |
IN/OUT |
Sparse matrix |
|
HOST |
IN |
Enumerator specifying the datatype in which the computation is executed |
|
HOST |
IN |
Enumerator specifying the algorithm for the computation |
|
HOST |
IN/OUT |
Opaque descriptor for storing internal data used across the three steps |
|
HOST |
OUT |
Pointer to a 64-bit integer that stores the number of intermediate products returned by |
|
HOST |
IN |
The fraction of total intermediate products being computed in a chunk. Used by |
|
HOST |
IN/OUT |
Number of bytes of workspace requested by |
|
HOST |
IN/OUT |
Number of bytes of workspace requested by |
|
HOST |
IN/OUT |
Number of bytes of workspace requested by |
|
DEVICE |
IN |
Pointer to workspace buffer needed by |
|
DEVICE |
IN |
Pointer to workspace buffer needed by |
|
DEVICE |
IN |
Pointer to workspace buffer needed by |
Currently, the function has the following limitations:
Only 32-bit indices
CUSPARSE_INDEX_32I
is supportedOnly CSR format
CUSPARSE_FORMAT_CSR
is supportedOnly
opA
,opB
equal toCUSPARSE_OPERATION_NON_TRANSPOSE
are supported
The data types combinations currently supported for cusparseSpGEMM
are listed below :
Uniform-precision computation:
|
---|
|
|
|
|
|
|
|
|
cusparseSpGEMM
routine runs for the following algorithms:
Algorithm |
Notes |
---|---|
|
Default algorithm. Currently, it is |
|
Algorithm 1
|
|
Algorithm 2
|
|
Algorithm 3
|
cusparseSpGEMM()
has the following properties:
The routine requires no extra storage
The routine supports asynchronous execution
The routine supports does not support CUDA graph capture
cusparseSpGEMM()
supports the following optimizations:
Hardware Memory Compression
See cusparseStatus_t for the description of the return status.
Please visit cuSPARSE Library Samples - cusparseSpGEMM for a code example.
14.6.15. cusparseSpGEMMreuse()
cusparseStatus_t
cusparseSpGEMM_createDescr(cusparseSpGEMMDescr_t* descr)
cusparseStatus_t
cusparseSpGEMM_destroyDescr(cusparseSpGEMMDescr_t descr)
cusparseStatus_t
cusparseSpGEMMreuse_workEstimation(cusparseHandle_t handle,
cusparseOperation_t opA,
cusparseOperation_t opB,
cusparseSpMatDescr_t matA, //const descriptor
cusparseSpMatDescr_t matB, //const descriptor
cusparseSpMatDescr_t matC,
cusparseSpGEMMAlg_t alg,
cusparseSpGEMMDescr_t spgemmDescr,
size_t* bufferSize1,
void* externalBuffer1)
cusparseStatus_t
cusparseSpGEMMreuse_nnz(cusparseHandle_t handle,
cusparseOperation_t opA,
cusparseOperation_t opB,
cusparseSpMatDescr_t matA, //const descriptor
cusparseSpMatDescr_t matB, //const descriptor
cusparseSpMatDescr_t matC,
cusparseSpGEMMAlg_t alg,
cusparseSpGEMMDescr_t spgemmDescr,
size_t* bufferSize2,
void* externalBuffer2,
size_t* bufferSize3,
void* externalBuffer3,
size_t* bufferSize4,
void* externalBuffer4)
cusparseStatus_t CUSPARSEAPI
cusparseSpGEMMreuse_copy(cusparseHandle_t handle,
cusparseOperation_t opA,
cusparseOperation_t opB,
cusparseSpMatDescr_t matA, //const descriptor
cusparseSpMatDescr_t matB, //const descriptor
cusparseSpMatDescr_t matC,
cusparseSpGEMMAlg_t alg,
cusparseSpGEMMDescr_t spgemmDescr,
size_t* bufferSize5,
void* externalBuffer5)
cusparseStatus_t CUSPARSEAPI
cusparseSpGEMMreuse_compute(cusparseHandle_t handle,
cusparseOperation_t opA,
cusparseOperation_t opB,
const void* alpha,
cusparseSpMatDescr_t matA, //const descriptor
cusparseSpMatDescr_t matB, //const descriptor
const void* beta,
cusparseSpMatDescr_t matC,
cudaDataType computeType,
cusparseSpGEMMAlg_t alg,
cusparseSpGEMMDescr_t spgemmDescr)
This function performs the multiplication of two sparse matrices matA
and matB
where the structure of the output matrix matC
can be reused for multiple computations with different values.
\(\mathbf{C^{\prime}} = \alpha op\left( \mathbf{A} \right) \cdot op\left( \mathbf{B} \right) + \beta\mathbf{C}\) |
where \(\alpha\) and \(\beta\) are scalars.
The functions cusparseSpGEMMreuse_workEstimation()
, cusparseSpGEMMreuse_nnz()
, and cusparseSpGEMMreuse_copy()
are used for determining the buffer size and performing the actual computation.
Note: cusparseSpGEMMreuse()
output CSR matrix (matC
) is sorted by column indices.
MEMORY REQUIREMENT: cusparseSpGEMMreuse
requires to keep in memory all intermediate products to reuse the structure of the output matrix. On the other hand, the number of intermediate products is orders of magnitude higher than the number of non-zero entries in general. In order to minimize the memory requirements, the routine uses multiple buffers that can be deallocated after they are no more needed. If the number of intermediate product exceeds 2^31-1
, the routine will returns CUSPARSE_STATUS_INSUFFICIENT_RESOURCES
status.
Currently, the function has the following limitations:
Only 32-bit indices
CUSPARSE_INDEX_32I
is supportedOnly CSR format
CUSPARSE_FORMAT_CSR
is supportedOnly
opA
,opB
equal toCUSPARSE_OPERATION_NON_TRANSPOSE
are supported
The data types combinations currently supported for cusparseSpGEMMreuse
are listed below.
Uniform-precision computation:
|
---|
|
|
|
|
|
|
Mixed-precision computation:
|
|
|
---|---|---|
|
|
|
|
|
|
cusparseSpGEMMreuse
routine runs for the following algorithm:
Algorithm |
Notes |
---|---|
|
Default algorithm. Provides deterministic (bit-wise) structure for the output matrix for each run, while value computation is not deterministic. |
|
Provides deterministic (bit-wise) structure for the output matrix and value computation for each run. |
cusparseSpGEMMreuse()
has the following properties:
The routine requires no extra storage
The routine supports asynchronous execution
The routine supports does not support CUDA graph capture
cusparseSpGEMMreuse()
supports the following optimizations:
Hardware Memory Compression
Refer to cusparseStatus_t for the description of the return status.
Please visit cuSPARSE Library Samples - cusparseSpGEMMreuse for a code example.
15. cuSPARSE Fortran Bindings
The cuSPARSE library is implemented using the C-based CUDA toolchain, and it thus provides a C-style API that makes interfacing to applications written in C or C++ trivial. There are also many applications implemented in Fortran that would benefit from using cuSPARSE, and therefore a cuSPARSE Fortran interface has been developed.
Unfortunately, Fortran-to-C calling conventions are not standardized and differ by platform and toolchain. In particular, differences may exist in the following areas:
Symbol names (capitalization, name decoration)
Argument passing (by value or reference)
Passing of pointer arguments (size of the pointer)
To provide maximum flexibility in addressing those differences, the cuSPARSE Fortran interface is provided in the form of wrapper functions, which are written in C and are located in the file cusparse_fortran.c
. This file also contains a few additional wrapper functions (for cudaMalloc()
, cudaMemset
, and so on) that can be used to allocate memory on the GPU.
The cuSPARSE Fortran wrapper code is provided as an example only and needs to be compiled into an application for it to call the cuSPARSE API functions. Providing this source code allows users to make any changes necessary for a particular platform and toolchain.
The cuSPARSE Fortran wrapper code has been used to demonstrate interoperability with the compilers g95 0.91 (on 32-bit and 64-bit Linux) and g95 0.92 (on 32-bit and 64-bit Mac OS X). In order to use other compilers, users have to make any changes to the wrapper code that may be required.
The direct wrappers, intended for production code, substitute device pointers for vector and matrix arguments in all cuSPARSE functions. To use these interfaces, existing applications need to be modified slightly to allocate and deallocate data structures in GPU memory space (using CUDA_MALLOC()
and CUDA_FREE()
) and to copy data between GPU and CPU memory spaces (using the CUDA_MEMCPY()
routines). The sample wrappers provided in cusparse_fortran.c
map device pointers to the OS-dependent type size_t
, which is 32 bits wide on 32-bit platforms and 64 bits wide on a 64-bit platforms.
One approach to dealing with index arithmetic on device pointers in Fortran code is to use C-style macros and to use the C preprocessor to expand them. On Linux and Mac OS X, preprocessing can be done by using the option '-cpp'
with g95 or gfortran. The function GET_SHIFTED_ADDRESS()
, provided with the cuSPARSE Fortran wrappers, can also be used, as shown in example B.
Example B shows the the C++ of example A implemented in Fortran 77 on the host. This example should be compiled with ARCH_64
defined as 1 on a 64-bit OS system and as undefined on a 32-bit OS system. For example, on g95 or gfortran, it can be done directly on the command line using the option -cpp -DARCH_64=1
.
15.1. Fortran Application
c #define ARCH_64 0
c #define ARCH_64 1
program cusparse_fortran_example
implicit none
integer cuda_malloc
external cuda_free
integer cuda_memcpy_c2fort_int
integer cuda_memcpy_c2fort_real
integer cuda_memcpy_fort2c_int
integer cuda_memcpy_fort2c_real
integer cuda_memset
integer cusparse_create
external cusparse_destroy
integer cusparse_get_version
integer cusparse_create_mat_descr
external cusparse_destroy_mat_descr
integer cusparse_set_mat_type
integer cusparse_get_mat_type
integer cusparse_get_mat_fill_mode
integer cusparse_get_mat_diag_type
integer cusparse_set_mat_index_base
integer cusparse_get_mat_index_base
integer cusparse_xcoo2csr
integer cusparse_dsctr
integer cusparse_dcsrmv
integer cusparse_dcsrmm
external get_shifted_address
#if ARCH_64
integer*8 handle
integer*8 descrA
integer*8 cooRowIndex
integer*8 cooColIndex
integer*8 cooVal
integer*8 xInd
integer*8 xVal
integer*8 y
integer*8 z
integer*8 csrRowPtr
integer*8 ynp1
#else
integer*4 handle
integer*4 descrA
integer*4 cooRowIndex
integer*4 cooColIndex
integer*4 cooVal
integer*4 xInd
integer*4 xVal
integer*4 y
integer*4 z
integer*4 csrRowPtr
integer*4 ynp1
#endif
integer status
integer cudaStat1,cudaStat2,cudaStat3
integer cudaStat4,cudaStat5,cudaStat6
integer n, nnz, nnz_vector
parameter (n=4, nnz=9, nnz_vector=3)
integer cooRowIndexHostPtr(nnz)
integer cooColIndexHostPtr(nnz)
real*8 cooValHostPtr(nnz)
integer xIndHostPtr(nnz_vector)
real*8 xValHostPtr(nnz_vector)
real*8 yHostPtr(2*n)
real*8 zHostPtr(2*(n+1))
integer i, j
integer version, mtype, fmode, dtype, ibase
real*8 dzero,dtwo,dthree,dfive
real*8 epsilon
write(*,*) "testing fortran example"
c predefined constants (need to be careful with them)
dzero = 0.0
dtwo = 2.0
dthree= 3.0
dfive = 5.0
c create the following sparse test matrix in COO format
c (notice one-based indexing)
c |1.0 2.0 3.0|
c | 4.0 |
c |5.0 6.0 7.0|
c | 8.0 9.0|
cooRowIndexHostPtr(1)=1
cooColIndexHostPtr(1)=1
cooValHostPtr(1) =1.0
cooRowIndexHostPtr(2)=1
cooColIndexHostPtr(2)=3
cooValHostPtr(2) =2.0
cooRowIndexHostPtr(3)=1
cooColIndexHostPtr(3)=4
cooValHostPtr(3) =3.0
cooRowIndexHostPtr(4)=2
cooColIndexHostPtr(4)=2
cooValHostPtr(4) =4.0
cooRowIndexHostPtr(5)=3
cooColIndexHostPtr(5)=1
cooValHostPtr(5) =5.0
cooRowIndexHostPtr(6)=3
cooColIndexHostPtr(6)=3
cooValHostPtr(6) =6.0
cooRowIndexHostPtr(7)=3
cooColIndexHostPtr(7)=4
cooValHostPtr(7) =7.0
cooRowIndexHostPtr(8)=4
cooColIndexHostPtr(8)=2
cooValHostPtr(8) =8.0
cooRowIndexHostPtr(9)=4
cooColIndexHostPtr(9)=4
cooValHostPtr(9) =9.0
c print the matrix
write(*,*) "Input data:"
do i=1,nnz
write(*,*) "cooRowIndexHostPtr[",i,"]=",cooRowIndexHostPtr(i)
write(*,*) "cooColIndexHostPtr[",i,"]=",cooColIndexHostPtr(i)
write(*,*) "cooValHostPtr[", i,"]=",cooValHostPtr(i)
enddo
c create a sparse and dense vector
c xVal= [100.0 200.0 400.0] (sparse)
c xInd= [0 1 3 ]
c y = [10.0 20.0 30.0 40.0 | 50.0 60.0 70.0 80.0] (dense)
c (notice one-based indexing)
yHostPtr(1) = 10.0
yHostPtr(2) = 20.0
yHostPtr(3) = 30.0
yHostPtr(4) = 40.0
yHostPtr(5) = 50.0
yHostPtr(6) = 60.0
yHostPtr(7) = 70.0
yHostPtr(8) = 80.0
xIndHostPtr(1)=1
xValHostPtr(1)=100.0
xIndHostPtr(2)=2
xValHostPtr(2)=200.0
xIndHostPtr(3)=4
xValHostPtr(3)=400.0
c print the vectors
do j=1,2
do i=1,n
write(*,*) "yHostPtr[",i,",",j,"]=",yHostPtr(i+n*(j-1))
enddo
enddo
do i=1,nnz_vector
write(*,*) "xIndHostPtr[",i,"]=",xIndHostPtr(i)
write(*,*) "xValHostPtr[",i,"]=",xValHostPtr(i)
enddo
c allocate GPU memory and copy the matrix and vectors into it
c cudaSuccess=0
c cudaMemcpyHostToDevice=1
cudaStat1 = cuda_malloc(cooRowIndex,nnz*4)
cudaStat2 = cuda_malloc(cooColIndex,nnz*4)
cudaStat3 = cuda_malloc(cooVal, nnz*8)
cudaStat4 = cuda_malloc(y, 2*n*8)
cudaStat5 = cuda_malloc(xInd,nnz_vector*4)
cudaStat6 = cuda_malloc(xVal,nnz_vector*8)
if ((cudaStat1 /= 0) .OR.
$ (cudaStat2 /= 0) .OR.
$ (cudaStat3 /= 0) .OR.
$ (cudaStat4 /= 0) .OR.
$ (cudaStat5 /= 0) .OR.
$ (cudaStat6 /= 0)) then
write(*,*) "Device malloc failed"
write(*,*) "cudaStat1=",cudaStat1
write(*,*) "cudaStat2=",cudaStat2
write(*,*) "cudaStat3=",cudaStat3
write(*,*) "cudaStat4=",cudaStat4
write(*,*) "cudaStat5=",cudaStat5
write(*,*) "cudaStat6=",cudaStat6
stop 2
endif
cudaStat1 = cuda_memcpy_fort2c_int(cooRowIndex,cooRowIndexHostPtr,
$ nnz*4,1)
cudaStat2 = cuda_memcpy_fort2c_int(cooColIndex,cooColIndexHostPtr,
$ nnz*4,1)
cudaStat3 = cuda_memcpy_fort2c_real(cooVal, cooValHostPtr,
$ nnz*8,1)
cudaStat4 = cuda_memcpy_fort2c_real(y, yHostPtr,
$ 2*n*8,1)
cudaStat5 = cuda_memcpy_fort2c_int(xInd, xIndHostPtr,
$ nnz_vector*4,1)
cudaStat6 = cuda_memcpy_fort2c_real(xVal, xValHostPtr,
$ nnz_vector*8,1)
if ((cudaStat1 /= 0) .OR.
$ (cudaStat2 /= 0) .OR.
$ (cudaStat3 /= 0) .OR.
$ (cudaStat4 /= 0) .OR.
$ (cudaStat5 /= 0) .OR.
$ (cudaStat6 /= 0)) then
write(*,*) "Memcpy from Host to Device failed"
write(*,*) "cudaStat1=",cudaStat1
write(*,*) "cudaStat2=",cudaStat2
write(*,*) "cudaStat3=",cudaStat3
write(*,*) "cudaStat4=",cudaStat4
write(*,*) "cudaStat5=",cudaStat5
write(*,*) "cudaStat6=",cudaStat6
call cuda_free(cooRowIndex)
call cuda_free(cooColIndex)
call cuda_free(cooVal)
call cuda_free(xInd)
call cuda_free(xVal)
call cuda_free(y)
stop 1
endif
c initialize cusparse library
c CUSPARSE_STATUS_SUCCESS=0
status = cusparse_create(handle)
if (status /= 0) then
write(*,*) "CUSPARSE Library initialization failed"
call cuda_free(cooRowIndex)
call cuda_free(cooColIndex)
call cuda_free(cooVal)
call cuda_free(xInd)
call cuda_free(xVal)
call cuda_free(y)
stop 1
endif
c get version
c CUSPARSE_STATUS_SUCCESS=0
status = cusparse_get_version(handle,version)
if (status /= 0) then
write(*,*) "CUSPARSE Library initialization failed"
call cuda_free(cooRowIndex)
call cuda_free(cooColIndex)
call cuda_free(cooVal)
call cuda_free(xInd)
call cuda_free(xVal)
call cuda_free(y)
call cusparse_destroy(handle)
stop 1
endif
write(*,*) "CUSPARSE Library version",version
c create and setup the matrix descriptor
c CUSPARSE_STATUS_SUCCESS=0
c CUSPARSE_MATRIX_TYPE_GENERAL=0
c CUSPARSE_INDEX_BASE_ONE=1
status= cusparse_create_mat_descr(descrA)
if (status /= 0) then
write(*,*) "Creating matrix descriptor failed"
call cuda_free(cooRowIndex)
call cuda_free(cooColIndex)
call cuda_free(cooVal)
call cuda_free(xInd)
call cuda_free(xVal)
call cuda_free(y)
call cusparse_destroy(handle)
stop 1
endif
status = cusparse_set_mat_type(descrA,0)
status = cusparse_set_mat_index_base(descrA,1)
c print the matrix descriptor
mtype = cusparse_get_mat_type(descrA)
fmode = cusparse_get_mat_fill_mode(descrA)
dtype = cusparse_get_mat_diag_type(descrA)
ibase = cusparse_get_mat_index_base(descrA)
write (*,*) "matrix descriptor:"
write (*,*) "t=",mtype,"m=",fmode,"d=",dtype,"b=",ibase
c exercise conversion routines (convert matrix from COO 2 CSR format)
c cudaSuccess=0
c CUSPARSE_STATUS_SUCCESS=0
c CUSPARSE_INDEX_BASE_ONE=1
cudaStat1 = cuda_malloc(csrRowPtr,(n+1)*4)
if (cudaStat1 /= 0) then
call cuda_free(cooRowIndex)
call cuda_free(cooColIndex)
call cuda_free(cooVal)
call cuda_free(xInd)
call cuda_free(xVal)
call cuda_free(y)
call cusparse_destroy_mat_descr(descrA)
call cusparse_destroy(handle)
write(*,*) "Device malloc failed (csrRowPtr)"
stop 2
endif
status= cusparse_xcoo2csr(handle,cooRowIndex,nnz,n,
$ csrRowPtr,1)
if (status /= 0) then
call cuda_free(cooRowIndex)
call cuda_free(cooColIndex)
call cuda_free(cooVal)
call cuda_free(xInd)
call cuda_free(xVal)
call cuda_free(y)
call cuda_free(csrRowPtr)
call cusparse_destroy_mat_descr(descrA)
call cusparse_destroy(handle)
write(*,*) "Conversion from COO to CSR format failed"
stop 1
endif
c csrRowPtr = [0 3 4 7 9]
c exercise Level 1 routines (scatter vector elements)
c CUSPARSE_STATUS_SUCCESS=0
c CUSPARSE_INDEX_BASE_ONE=1
call get_shifted_address(y,n*8,ynp1)
status= cusparse_dsctr(handle, nnz_vector, xVal, xInd,
$ ynp1, 1)
if (status /= 0) then
call cuda_free(cooRowIndex)
call cuda_free(cooColIndex)
call cuda_free(cooVal)
call cuda_free(xInd)
call cuda_free(xVal)
call cuda_free(y)
call cuda_free(csrRowPtr)
call cusparse_destroy_mat_descr(descrA)
call cusparse_destroy(handle)
write(*,*) "Scatter from sparse to dense vector failed"
stop 1
endif
c y = [10 20 30 40 | 100 200 70 400]
c exercise Level 2 routines (csrmv)
c CUSPARSE_STATUS_SUCCESS=0
c CUSPARSE_OPERATION_NON_TRANSPOSE=0
status= cusparse_dcsrmv(handle, 0, n, n, nnz, dtwo,
$ descrA, cooVal, csrRowPtr, cooColIndex,
$ y, dthree, ynp1)
if (status /= 0) then
call cuda_free(cooRowIndex)
call cuda_free(cooColIndex)
call cuda_free(cooVal)
call cuda_free(xInd)
call cuda_free(xVal)
call cuda_free(y)
call cuda_free(csrRowPtr)
call cusparse_destroy_mat_descr(descrA)
call cusparse_destroy(handle)
write(*,*) "Matrix-vector multiplication failed"
stop 1
endif
c print intermediate results (y)
c y = [10 20 30 40 | 680 760 1230 2240]
c cudaSuccess=0
c cudaMemcpyDeviceToHost=2
cudaStat1 = cuda_memcpy_c2fort_real(yHostPtr, y, 2*n*8, 2)
if (cudaStat1 /= 0) then
call cuda_free(cooRowIndex)
call cuda_free(cooColIndex)
call cuda_free(cooVal)
call cuda_free(xInd)
call cuda_free(xVal)
call cuda_free(y)
call cuda_free(csrRowPtr)
call cusparse_destroy_mat_descr(descrA)
call cusparse_destroy(handle)
write(*,*) "Memcpy from Device to Host failed"
stop 1
endif
write(*,*) "Intermediate results:"
do j=1,2
do i=1,n
write(*,*) "yHostPtr[",i,",",j,"]=",yHostPtr(i+n*(j-1))
enddo
enddo
c exercise Level 3 routines (csrmm)
c cudaSuccess=0
c CUSPARSE_STATUS_SUCCESS=0
c CUSPARSE_OPERATION_NON_TRANSPOSE=0
cudaStat1 = cuda_malloc(z, 2*(n+1)*8)
if (cudaStat1 /= 0) then
call cuda_free(cooRowIndex)
call cuda_free(cooColIndex)
call cuda_free(cooVal)
call cuda_free(xInd)
call cuda_free(xVal)
call cuda_free(y)
call cuda_free(csrRowPtr)
call cusparse_destroy_mat_descr(descrA)
call cusparse_destroy(handle)
write(*,*) "Device malloc failed (z)"
stop 2
endif
cudaStat1 = cuda_memset(z, 0, 2*(n+1)*8)
if (cudaStat1 /= 0) then
call cuda_free(cooRowIndex)
call cuda_free(cooColIndex)
call cuda_free(cooVal)
call cuda_free(xInd)
call cuda_free(xVal)
call cuda_free(y)
call cuda_free(z)
call cuda_free(csrRowPtr)
call cusparse_destroy_mat_descr(descrA)
call cusparse_destroy(handle)
write(*,*) "Memset on Device failed"
stop 1
endif
status= cusparse_dcsrmm(handle, 0, n, 2, n, nnz, dfive,
$ descrA, cooVal, csrRowPtr, cooColIndex,
$ y, n, dzero, z, n+1)
if (status /= 0) then
call cuda_free(cooRowIndex)
call cuda_free(cooColIndex)
call cuda_free(cooVal)
call cuda_free(xInd)
call cuda_free(xVal)
call cuda_free(y)
call cuda_free(z)
call cuda_free(csrRowPtr)
call cusparse_destroy_mat_descr(descrA)
call cusparse_destroy(handle)
write(*,*) "Matrix-matrix multiplication failed"
stop 1
endif
c print final results (z)
c cudaSuccess=0
c cudaMemcpyDeviceToHost=2
cudaStat1 = cuda_memcpy_c2fort_real(zHostPtr, z, 2*(n+1)*8, 2)
if (cudaStat1 /= 0) then
call cuda_free(cooRowIndex)
call cuda_free(cooColIndex)
call cuda_free(cooVal)
call cuda_free(xInd)
call cuda_free(xVal)
call cuda_free(y)
call cuda_free(z)
call cuda_free(csrRowPtr)
call cusparse_destroy_mat_descr(descrA)
call cusparse_destroy(handle)
write(*,*) "Memcpy from Device to Host failed"
stop 1
endif
c z = [950 400 2550 2600 0 | 49300 15200 132300 131200 0]
write(*,*) "Final results:"
do j=1,2
do i=1,n+1
write(*,*) "z[",i,",",j,"]=",zHostPtr(i+(n+1)*(j-1))
enddo
enddo
c check the results
epsilon = 0.00000000000001
if ((DABS(zHostPtr(1) - 950.0) .GT. epsilon) .OR.
$ (DABS(zHostPtr(2) - 400.0) .GT. epsilon) .OR.
$ (DABS(zHostPtr(3) - 2550.0) .GT. epsilon) .OR.
$ (DABS(zHostPtr(4) - 2600.0) .GT. epsilon) .OR.
$ (DABS(zHostPtr(5) - 0.0) .GT. epsilon) .OR.
$ (DABS(zHostPtr(6) - 49300.0) .GT. epsilon) .OR.
$ (DABS(zHostPtr(7) - 15200.0) .GT. epsilon) .OR.
$ (DABS(zHostPtr(8) - 132300.0).GT. epsilon) .OR.
$ (DABS(zHostPtr(9) - 131200.0).GT. epsilon) .OR.
$ (DABS(zHostPtr(10) - 0.0) .GT. epsilon) .OR.
$ (DABS(yHostPtr(1) - 10.0) .GT. epsilon) .OR.
$ (DABS(yHostPtr(2) - 20.0) .GT. epsilon) .OR.
$ (DABS(yHostPtr(3) - 30.0) .GT. epsilon) .OR.
$ (DABS(yHostPtr(4) - 40.0) .GT. epsilon) .OR.
$ (DABS(yHostPtr(5) - 680.0) .GT. epsilon) .OR.
$ (DABS(yHostPtr(6) - 760.0) .GT. epsilon) .OR.
$ (DABS(yHostPtr(7) - 1230.0) .GT. epsilon) .OR.
$ (DABS(yHostPtr(8) - 2240.0) .GT. epsilon)) then
write(*,*) "fortran example test FAILED"
else
write(*,*) "fortran example test PASSED"
endif
c deallocate GPU memory and exit
call cuda_free(cooRowIndex)
call cuda_free(cooColIndex)
call cuda_free(cooVal)
call cuda_free(xInd)
call cuda_free(xVal)
call cuda_free(y)
call cuda_free(z)
call cuda_free(csrRowPtr)
call cusparse_destroy_mat_descr(descrA)
call cusparse_destroy(handle)
stop 0
end
16. Examples of prune
16.1. Prune Dense to Sparse
This section provides a simple example in the C programming language of pruning a dense matrix to a sparse matrix of CSR format.
A is a 4x4 dense matrix,
\(A = \begin{pmatrix} {1.0} & {0.0} & {2.0} & {-3.0} \\ {0.0} & {4.0} & {0.0} & {0.0} \\ {5.0} & {0.0} & {6.0} & {7.0} \\ {0.0} & {8.0} & {0.0} & {9.0} \\ \end{pmatrix}\) |
/*
* How to compile (assume cuda is installed at /usr/local/cuda/)
* nvcc -c -I/usr/local/cuda/include prunedense_example.cpp
* g++ -o prunedense_example.cpp prunedense_example.o -L/usr/local/cuda/lib64 -lcusparse -lcudart
*/
#include <stdio.h>
#include <stdlib.h>
#include <assert.h>
#include <cuda_runtime.h>
#include <cusparse.h>
void printMatrix(int m, int n, const float*A, int lda, const char* name)
{
for(int row = 0 ; row < m ; row++){
for(int col = 0 ; col < n ; col++){
float Areg = A[row + col*lda];
printf("%s(%d,%d) = %f\n", name, row+1, col+1, Areg);
}
}
}
void printCsr(
int m,
int n,
int nnz,
const cusparseMatDescr_t descrA,
const float *csrValA,
const int *csrRowPtrA,
const int *csrColIndA,
const char* name)
{
const int base = (cusparseGetMatIndexBase(descrA) != CUSPARSE_INDEX_BASE_ONE)? 0:1 ;
printf("matrix %s is %d-by-%d, nnz=%d, base=%d\n", name, m, n, nnz, base);
for(int row = 0 ; row < m ; row++){
const int start = csrRowPtrA[row ] - base;
const int end = csrRowPtrA[row+1] - base;
for(int colidx = start ; colidx < end ; colidx++){
const int col = csrColIndA[colidx] - base;
const float Areg = csrValA[colidx];
printf("%s(%d,%d) = %f\n", name, row+1, col+1, Areg);
}
}
}
int main(int argc, char*argv[])
{
cusparseHandle_t handle = NULL;
cudaStream_t stream = NULL;
cusparseMatDescr_t descrC = NULL;
cusparseStatus_t status = CUSPARSE_STATUS_SUCCESS;
cudaError_t cudaStat1 = cudaSuccess;
cudaError_t cudaStat2 = cudaSuccess;
cudaError_t cudaStat3 = cudaSuccess;
cudaError_t cudaStat4 = cudaSuccess;
cudaError_t cudaStat5 = cudaSuccess;
const int m = 4;
const int n = 4;
const int lda = m;
/*
* | 1 0 2 -3 |
* | 0 4 0 0 |
* A = | 5 0 6 7 |
* | 0 8 0 9 |
*
*/
const float A[lda*n] = {1, 0, 5, 0, 0, 4, 0, 8, 2, 0, 6, 0, -3, 0, 7, 9};
int* csrRowPtrC = NULL;
int* csrColIndC = NULL;
float* csrValC = NULL;
float *d_A = NULL;
int *d_csrRowPtrC = NULL;
int *d_csrColIndC = NULL;
float *d_csrValC = NULL;
size_t lworkInBytes = 0;
char *d_work = NULL;
int nnzC = 0;
float threshold = 4.1; /* remove Aij <= 4.1 */
// float threshold = 0; /* remove zeros */
printf("example of pruneDense2csr \n");
printf("prune |A(i,j)| <= threshold \n");
printf("threshold = %E \n", threshold);
printMatrix(m, n, A, lda, "A");
/* step 1: create cusparse handle, bind a stream */
cudaStat1 = cudaStreamCreateWithFlags(&stream, cudaStreamNonBlocking);
assert(cudaSuccess == cudaStat1);
status = cusparseCreate(&handle);
assert(CUSPARSE_STATUS_SUCCESS == status);
status = cusparseSetStream(handle, stream);
assert(CUSPARSE_STATUS_SUCCESS == status);
/* step 2: configuration of matrix C */
status = cusparseCreateMatDescr(&descrC);
assert(CUSPARSE_STATUS_SUCCESS == status);
cusparseSetMatIndexBase(descrC,CUSPARSE_INDEX_BASE_ZERO);
cusparseSetMatType(descrC, CUSPARSE_MATRIX_TYPE_GENERAL );
cudaStat1 = cudaMalloc ((void**)&d_A , sizeof(float)*lda*n );
cudaStat2 = cudaMalloc ((void**)&d_csrRowPtrC, sizeof(int)*(m+1) );
assert(cudaSuccess == cudaStat1);
assert(cudaSuccess == cudaStat2);
/* step 3: query workspace */
cudaStat1 = cudaMemcpy(d_A, A, sizeof(float)*lda*n, cudaMemcpyHostToDevice);
assert(cudaSuccess == cudaStat1);
status = cusparseSpruneDense2csr_bufferSizeExt(
handle,
m,
n,
d_A,
lda,
&threshold,
descrC,
d_csrValC,
d_csrRowPtrC,
d_csrColIndC,
&lworkInBytes);
assert(CUSPARSE_STATUS_SUCCESS == status);
printf("lworkInBytes (prune) = %lld \n", (long long)lworkInBytes);
if (NULL != d_work) { cudaFree(d_work); }
cudaStat1 = cudaMalloc((void**)&d_work, lworkInBytes);
assert(cudaSuccess == cudaStat1);
/* step 4: compute csrRowPtrC and nnzC */
status = cusparseSpruneDense2csrNnz(
handle,
m,
n,
d_A,
lda,
&threshold,
descrC,
d_csrRowPtrC,
&nnzC, /* host */
d_work);
assert(CUSPARSE_STATUS_SUCCESS == status);
cudaStat1 = cudaDeviceSynchronize();
assert(cudaSuccess == cudaStat1);
printf("nnzC = %d\n", nnzC);
if (0 == nnzC ){
printf("C is empty \n");
return 0;
}
/* step 5: compute csrColIndC and csrValC */
cudaStat1 = cudaMalloc ((void**)&d_csrColIndC, sizeof(int ) * nnzC );
cudaStat2 = cudaMalloc ((void**)&d_csrValC , sizeof(float) * nnzC );
assert(cudaSuccess == cudaStat1);
assert(cudaSuccess == cudaStat2);
status = cusparseSpruneDense2csr(
handle,
m,
n,
d_A,
lda,
&threshold,
descrC,
d_csrValC,
d_csrRowPtrC,
d_csrColIndC,
d_work);
assert(CUSPARSE_STATUS_SUCCESS == status);
cudaStat1 = cudaDeviceSynchronize();
assert(cudaSuccess == cudaStat1);
/* step 6: output C */
csrRowPtrC = (int* )malloc(sizeof(int )*(m+1));
csrColIndC = (int* )malloc(sizeof(int )*nnzC);
csrValC = (float*)malloc(sizeof(float)*nnzC);
assert( NULL != csrRowPtrC);
assert( NULL != csrColIndC);
assert( NULL != csrValC);
cudaStat1 = cudaMemcpy(csrRowPtrC, d_csrRowPtrC, sizeof(int )*(m+1), cudaMemcpyDeviceToHost);
cudaStat2 = cudaMemcpy(csrColIndC, d_csrColIndC, sizeof(int )*nnzC , cudaMemcpyDeviceToHost);
cudaStat3 = cudaMemcpy(csrValC , d_csrValC , sizeof(float)*nnzC , cudaMemcpyDeviceToHost);
assert(cudaSuccess == cudaStat1);
assert(cudaSuccess == cudaStat2);
assert(cudaSuccess == cudaStat3);
printCsr(m, n, nnzC, descrC, csrValC, csrRowPtrC, csrColIndC, "C");
/* free resources */
if (d_A ) cudaFree(d_A);
if (d_csrRowPtrC ) cudaFree(d_csrRowPtrC);
if (d_csrColIndC ) cudaFree(d_csrColIndC);
if (d_csrValC ) cudaFree(d_csrValC);
if (csrRowPtrC ) free(csrRowPtrC);
if (csrColIndC ) free(csrColIndC);
if (csrValC ) free(csrValC);
if (handle ) cusparseDestroy(handle);
if (stream ) cudaStreamDestroy(stream);
if (descrC ) cusparseDestroyMatDescr(descrC);
cudaDeviceReset();
return 0;
}
16.2. Prune Sparse to Sparse
This section provides a simple example in the C programming language of pruning a sparse matrix to a sparse matrix of CSR format.
A is a 4x4 sparse matrix,
\(A = \begin{pmatrix} {1.0} & {0.0} & {2.0} & {-3.0} \\ {0.0} & {4.0} & {0.0} & {0.0} \\ {5.0} & {0.0} & {6.0} & {7.0} \\ {0.0} & {8.0} & {0.0} & {9.0} \\ \end{pmatrix}\) |
/*
* How to compile (assume cuda is installed at /usr/local/cuda/)
* nvcc -c -I/usr/local/cuda/include prunecsr_example.cpp
* g++ -o prunecsr_example.cpp prunecsr_example.o -L/usr/local/cuda/lib64 -lcusparse -lcudart
*/
#include <stdio.h>
#include <stdlib.h>
#include <assert.h>
#include <cuda_runtime.h>
#include <cusparse.h>
void printCsr(
int m,
int n,
int nnz,
const cusparseMatDescr_t descrA,
const float *csrValA,
const int *csrRowPtrA,
const int *csrColIndA,
const char* name)
{
const int base = (cusparseGetMatIndexBase(descrA) != CUSPARSE_INDEX_BASE_ONE)? 0:1 ;
printf("matrix %s is %d-by-%d, nnz=%d, base=%d, output base-1\n", name, m, n, nnz, base);
for(int row = 0 ; row < m ; row++){
const int start = csrRowPtrA[row ] - base;
const int end = csrRowPtrA[row+1] - base;
for(int colidx = start ; colidx < end ; colidx++){
const int col = csrColIndA[colidx] - base;
const float Areg = csrValA[colidx];
printf("%s(%d,%d) = %f\n", name, row+1, col+1, Areg);
}
}
}
int main(int argc, char*argv[])
{
cusparseHandle_t handle = NULL;
cudaStream_t stream = NULL;
cusparseMatDescr_t descrA = NULL;
cusparseMatDescr_t descrC = NULL;
cusparseStatus_t status = CUSPARSE_STATUS_SUCCESS;
cudaError_t cudaStat1 = cudaSuccess;
const int m = 4;
const int n = 4;
const int nnzA = 9;
/*
* | 1 0 2 -3 |
* | 0 4 0 0 |
* A = | 5 0 6 7 |
* | 0 8 0 9 |
*
*/
const int csrRowPtrA[m+1] = { 1, 4, 5, 8, 10};
const int csrColIndA[nnzA] = { 1, 3, 4, 2, 1, 3, 4, 2, 4};
const float csrValA[nnzA] = {1, 2, -3, 4, 5, 6, 7, 8, 9};
int* csrRowPtrC = NULL;
int* csrColIndC = NULL;
float* csrValC = NULL;
int *d_csrRowPtrA = NULL;
int *d_csrColIndA = NULL;
float *d_csrValA = NULL;
int *d_csrRowPtrC = NULL;
int *d_csrColIndC = NULL;
float *d_csrValC = NULL;
size_t lworkInBytes = 0;
char *d_work = NULL;
int nnzC = 0;
float threshold = 4.1; /* remove Aij <= 4.1 */
// float threshold = 0; /* remove zeros */
printf("example of pruneCsr2csr \n");
printf("prune |A(i,j)| <= threshold \n");
printf("threshold = %E \n", threshold);
/* step 1: create cusparse handle, bind a stream */
cudaStat1 = cudaStreamCreateWithFlags(&stream, cudaStreamNonBlocking);
assert(cudaSuccess == cudaStat1);
status = cusparseCreate(&handle);
assert(CUSPARSE_STATUS_SUCCESS == status);
status = cusparseSetStream(handle, stream);
assert(CUSPARSE_STATUS_SUCCESS == status);
/* step 2: configuration of matrix A and C */
status = cusparseCreateMatDescr(&descrA);
assert(CUSPARSE_STATUS_SUCCESS == status);
/* A is base-1*/
cusparseSetMatIndexBase(descrA,CUSPARSE_INDEX_BASE_ONE);
cusparseSetMatType(descrA, CUSPARSE_MATRIX_TYPE_GENERAL );
status = cusparseCreateMatDescr(&descrC);
assert(CUSPARSE_STATUS_SUCCESS == status);
/* C is base-0 */
cusparseSetMatIndexBase(descrC,CUSPARSE_INDEX_BASE_ZERO);
cusparseSetMatType(descrC, CUSPARSE_MATRIX_TYPE_GENERAL );
printCsr(m, n, nnzA, descrA, csrValA, csrRowPtrA, csrColIndA, "A");
cudaStat1 = cudaMalloc ((void**)&d_csrRowPtrA, sizeof(int)*(m+1) );
assert(cudaSuccess == cudaStat1);
cudaStat1 = cudaMalloc ((void**)&d_csrColIndA, sizeof(int)*nnzA );
assert(cudaSuccess == cudaStat1);
cudaStat1 = cudaMalloc ((void**)&d_csrValA , sizeof(float)*nnzA );
assert(cudaSuccess == cudaStat1);
cudaStat1 = cudaMalloc ((void**)&d_csrRowPtrC, sizeof(int)*(m+1) );
assert(cudaSuccess == cudaStat1);
cudaStat1 = cudaMemcpy(d_csrRowPtrA, csrRowPtrA, sizeof(int)*(m+1), cudaMemcpyHostToDevice);
assert(cudaSuccess == cudaStat1);
cudaStat1 = cudaMemcpy(d_csrColIndA, csrColIndA, sizeof(int)*nnzA, cudaMemcpyHostToDevice);
assert(cudaSuccess == cudaStat1);
cudaStat1 = cudaMemcpy(d_csrValA , csrValA , sizeof(float)*nnzA, cudaMemcpyHostToDevice);
assert(cudaSuccess == cudaStat1);
/* step 3: query workspace */
status = cusparseSpruneCsr2csr_bufferSizeExt(
handle,
m,
n,
nnzA,
descrA,
d_csrValA,
d_csrRowPtrA,
d_csrColIndA,
&threshold,
descrC,
d_csrValC,
d_csrRowPtrC,
d_csrColIndC,
&lworkInBytes);
assert(CUSPARSE_STATUS_SUCCESS == status);
printf("lworkInBytes (prune) = %lld \n", (long long)lworkInBytes);
if (NULL != d_work) { cudaFree(d_work); }
cudaStat1 = cudaMalloc((void**)&d_work, lworkInBytes);
assert(cudaSuccess == cudaStat1);
/* step 4: compute csrRowPtrC and nnzC */
status = cusparseSpruneCsr2csrNnz(
handle,
m,
n,
nnzA,
descrA,
d_csrValA,
d_csrRowPtrA,
d_csrColIndA,
&threshold,
descrC,
d_csrRowPtrC,
&nnzC, /* host */
d_work);
assert(CUSPARSE_STATUS_SUCCESS == status);
cudaStat1 = cudaDeviceSynchronize();
assert(cudaSuccess == cudaStat1);
printf("nnzC = %d\n", nnzC);
if (0 == nnzC ){
printf("C is empty \n");
return 0;
}
/* step 5: compute csrColIndC and csrValC */
cudaStat1 = cudaMalloc ((void**)&d_csrColIndC, sizeof(int ) * nnzC );
assert(cudaSuccess == cudaStat1);
cudaStat1 = cudaMalloc ((void**)&d_csrValC , sizeof(float) * nnzC );
assert(cudaSuccess == cudaStat1);
status = cusparseSpruneCsr2csr(
handle,
m,
n,
nnzA,
descrA,
d_csrValA,
d_csrRowPtrA,
d_csrColIndA,
&threshold,
descrC,
d_csrValC,
d_csrRowPtrC,
d_csrColIndC,
d_work);
assert(CUSPARSE_STATUS_SUCCESS == status);
cudaStat1 = cudaDeviceSynchronize();
assert(cudaSuccess == cudaStat1);
/* step 6: output C */
csrRowPtrC = (int* )malloc(sizeof(int )*(m+1));
csrColIndC = (int* )malloc(sizeof(int )*nnzC);
csrValC = (float*)malloc(sizeof(float)*nnzC);
assert( NULL != csrRowPtrC);
assert( NULL != csrColIndC);
assert( NULL != csrValC);
cudaStat1 = cudaMemcpy(csrRowPtrC, d_csrRowPtrC, sizeof(int )*(m+1), cudaMemcpyDeviceToHost);
assert(cudaSuccess == cudaStat1);
cudaStat1 = cudaMemcpy(csrColIndC, d_csrColIndC, sizeof(int )*nnzC , cudaMemcpyDeviceToHost);
assert(cudaSuccess == cudaStat1);
cudaStat1 = cudaMemcpy(csrValC , d_csrValC , sizeof(float)*nnzC , cudaMemcpyDeviceToHost);
assert(cudaSuccess == cudaStat1);
printCsr(m, n, nnzC, descrC, csrValC, csrRowPtrC, csrColIndC, "C");
/* free resources */
if (d_csrRowPtrA ) cudaFree(d_csrRowPtrA);
if (d_csrColIndA ) cudaFree(d_csrColIndA);
if (d_csrValA ) cudaFree(d_csrValA);
if (d_csrRowPtrC ) cudaFree(d_csrRowPtrC);
if (d_csrColIndC ) cudaFree(d_csrColIndC);
if (d_csrValC ) cudaFree(d_csrValC);
if (csrRowPtrC ) free(csrRowPtrC);
if (csrColIndC ) free(csrColIndC);
if (csrValC ) free(csrValC);
if (handle ) cusparseDestroy(handle);
if (stream ) cudaStreamDestroy(stream);
if (descrA ) cusparseDestroyMatDescr(descrA);
if (descrC ) cusparseDestroyMatDescr(descrC);
cudaDeviceReset();
return 0;
}
16.3. Prune Dense to Sparse by Percentage
This section provides a simple example in the C programming language of pruning a dense matrix to a sparse matrix by percentage.
A is a 4x4 dense matrix,
\(A = \begin{pmatrix} {1.0} & {0.0} & {2.0} & {-3.0} \\ {0.0} & {4.0} & {0.0} & {0.0} \\ {5.0} & {0.0} & {6.0} & {7.0} \\ {0.0} & {8.0} & {0.0} & {9.0} \\ \end{pmatrix}\) |
The percentage is 50, which means to prune 50 percent of the dense matrix. The matrix has 16 elements, so 8 out of 16 must be pruned out. Therefore 7 zeros are pruned out, and value 1.0 is also out because it is the smallest among 9 nonzero elements.
/*
* How to compile (assume cuda is installed at /usr/local/cuda/)
* nvcc -c -I/usr/local/cuda/include prunedense2csrbyP.cpp
* g++ -o prunedense2csrbyP.cpp prunedense2csrbyP.o -L/usr/local/cuda/lib64 -lcusparse -lcudart
*/
#include <stdio.h>
#include <stdlib.h>
#include <assert.h>
#include <cuda_runtime.h>
#include <cusparse.h>
void printMatrix(int m, int n, const float*A, int lda, const char* name)
{
for(int row = 0 ; row < m ; row++){
for(int col = 0 ; col < n ; col++){
float Areg = A[row + col*lda];
printf("%s(%d,%d) = %f\n", name, row+1, col+1, Areg);
}
}
}
void printCsr(
int m,
int n,
int nnz,
const cusparseMatDescr_t descrA,
const float *csrValA,
const int *csrRowPtrA,
const int *csrColIndA,
const char* name)
{
const int base = (cusparseGetMatIndexBase(descrA) != CUSPARSE_INDEX_BASE_ONE)? 0:1 ;
printf("matrix %s is %d-by-%d, nnz=%d, base=%d, output base-1\n", name, m, n, nnz, base);
for(int row = 0 ; row < m ; row++){
const int start = csrRowPtrA[row ] - base;
const int end = csrRowPtrA[row+1] - base;
for(int colidx = start ; colidx < end ; colidx++){
const int col = csrColIndA[colidx] - base;
const float Areg = csrValA[colidx];
printf("%s(%d,%d) = %f\n", name, row+1, col+1, Areg);
}
}
}
int main(int argc, char*argv[])
{
cusparseHandle_t handle = NULL;
cudaStream_t stream = NULL;
cusparseMatDescr_t descrC = NULL;
pruneInfo_t info = NULL;
cusparseStatus_t status = CUSPARSE_STATUS_SUCCESS;
cudaError_t cudaStat1 = cudaSuccess;
cudaError_t cudaStat2 = cudaSuccess;
cudaError_t cudaStat3 = cudaSuccess;
cudaError_t cudaStat4 = cudaSuccess;
cudaError_t cudaStat5 = cudaSuccess;
const int m = 4;
const int n = 4;
const int lda = m;
/*
* | 1 0 2 -3 |
* | 0 4 0 0 |
* A = | 5 0 6 7 |
* | 0 8 0 9 |
*
*/
const float A[lda*n] = {1, 0, 5, 0, 0, 4, 0, 8, 2, 0, 6, 0, -3, 0, 7, 9};
int* csrRowPtrC = NULL;
int* csrColIndC = NULL;
float* csrValC = NULL;
float *d_A = NULL;
int *d_csrRowPtrC = NULL;
int *d_csrColIndC = NULL;
float *d_csrValC = NULL;
size_t lworkInBytes = 0;
char *d_work = NULL;
int nnzC = 0;
float percentage = 50; /* 50% of nnz */
printf("example of pruneDense2csrByPercentage \n");
printf("prune out %.1f percentage of A \n", percentage);
printMatrix(m, n, A, lda, "A");
/* step 1: create cusparse handle, bind a stream */
cudaStat1 = cudaStreamCreateWithFlags(&stream, cudaStreamNonBlocking);
assert(cudaSuccess == cudaStat1);
status = cusparseCreate(&handle);
assert(CUSPARSE_STATUS_SUCCESS == status);
status = cusparseSetStream(handle, stream);
assert(CUSPARSE_STATUS_SUCCESS == status);
status = cusparseCreatePruneInfo(&info);
assert(CUSPARSE_STATUS_SUCCESS == status);
/* step 2: configuration of matrix C */
status = cusparseCreateMatDescr(&descrC);
assert(CUSPARSE_STATUS_SUCCESS == status);
cusparseSetMatIndexBase(descrC,CUSPARSE_INDEX_BASE_ZERO);
cusparseSetMatType(descrC, CUSPARSE_MATRIX_TYPE_GENERAL );
cudaStat1 = cudaMalloc ((void**)&d_A , sizeof(float)*lda*n );
cudaStat2 = cudaMalloc ((void**)&d_csrRowPtrC, sizeof(int)*(m+1) );
assert(cudaSuccess == cudaStat1);
assert(cudaSuccess == cudaStat2);
cudaStat1 = cudaMemcpy(d_A, A, sizeof(float)*lda*n, cudaMemcpyHostToDevice);
assert(cudaSuccess == cudaStat1);
/* step 3: query workspace */
status = cusparseSpruneDense2csrByPercentage_bufferSizeExt(
handle,
m,
n,
d_A,
lda,
percentage,
descrC,
d_csrValC,
d_csrRowPtrC,
d_csrColIndC,
info,
&lworkInBytes);
assert(CUSPARSE_STATUS_SUCCESS == status);
printf("lworkInBytes = %lld \n", (long long)lworkInBytes);
if (NULL != d_work) { cudaFree(d_work); }
cudaStat1 = cudaMalloc((void**)&d_work, lworkInBytes);
assert(cudaSuccess == cudaStat1);
/* step 4: compute csrRowPtrC and nnzC */
status = cusparseSpruneDense2csrNnzByPercentage(
handle,
m,
n,
d_A,
lda,
percentage,
descrC,
d_csrRowPtrC,
&nnzC, /* host */
info,
d_work);
assert(CUSPARSE_STATUS_SUCCESS == status);
cudaStat1 = cudaDeviceSynchronize();
assert(cudaSuccess == cudaStat1);
printf("nnzC = %d\n", nnzC);
if (0 == nnzC ){
printf("C is empty \n");
return 0;
}
/* step 5: compute csrColIndC and csrValC */
cudaStat1 = cudaMalloc ((void**)&d_csrColIndC, sizeof(int ) * nnzC );
cudaStat2 = cudaMalloc ((void**)&d_csrValC , sizeof(float) * nnzC );
assert(cudaSuccess == cudaStat1);
assert(cudaSuccess == cudaStat2);
status = cusparseSpruneDense2csrByPercentage(
handle,
m,
n,
d_A,
lda,
percentage,
descrC,
d_csrValC,
d_csrRowPtrC,
d_csrColIndC,
info,
d_work);
assert(CUSPARSE_STATUS_SUCCESS == status);
cudaStat1 = cudaDeviceSynchronize();
assert(cudaSuccess == cudaStat1);
/* step 7: output C */
csrRowPtrC = (int* )malloc(sizeof(int )*(m+1));
csrColIndC = (int* )malloc(sizeof(int )*nnzC);
csrValC = (float*)malloc(sizeof(float)*nnzC);
assert( NULL != csrRowPtrC);
assert( NULL != csrColIndC);
assert( NULL != csrValC);
cudaStat1 = cudaMemcpy(csrRowPtrC, d_csrRowPtrC, sizeof(int )*(m+1), cudaMemcpyDeviceToHost);
cudaStat2 = cudaMemcpy(csrColIndC, d_csrColIndC, sizeof(int )*nnzC , cudaMemcpyDeviceToHost);
cudaStat3 = cudaMemcpy(csrValC , d_csrValC , sizeof(float)*nnzC , cudaMemcpyDeviceToHost);
assert(cudaSuccess == cudaStat1);
assert(cudaSuccess == cudaStat2);
assert(cudaSuccess == cudaStat3);
printCsr(m, n, nnzC, descrC, csrValC, csrRowPtrC, csrColIndC, "C");
/* free resources */
if (d_A ) cudaFree(d_A);
if (d_csrRowPtrC) cudaFree(d_csrRowPtrC);
if (d_csrColIndC) cudaFree(d_csrColIndC);
if (d_csrValC ) cudaFree(d_csrValC);
if (csrRowPtrC ) free(csrRowPtrC);
if (csrColIndC ) free(csrColIndC);
if (csrValC ) free(csrValC);
if (handle ) cusparseDestroy(handle);
if (stream ) cudaStreamDestroy(stream);
if (descrC ) cusparseDestroyMatDescr(descrC);
if (info ) cusparseDestroyPruneInfo(info);
cudaDeviceReset();
return 0;
}
16.4. Prune Sparse to Sparse by Percentage
This section provides a simple example in the C programming language of pruning a sparse matrix to a sparse matrix by percentage.
A is a 4x4 sparse matrix,
\(A = \begin{pmatrix} {1.0} & {0.0} & {2.0} & {-3.0} \\ {0.0} & {4.0} & {0.0} & {0.0} \\ {5.0} & {0.0} & {6.0} & {7.0} \\ {0.0} & {8.0} & {0.0} & {9.0} \\ \end{pmatrix}\) |
The percentage is 20, which means to prune 20 percent of the nonzeros. The sparse matrix has 9 nonzero elements, so 1.4 elements must be pruned out. The function removes 1.0 and 2.0 which are first two smallest numbers of nonzeros.
/*
* How to compile (assume cuda is installed at /usr/local/cuda/)
* nvcc -c -I/usr/local/cuda/include prunecsr2csrByP.cpp
* g++ -o prunecsr2csrByP.cpp prunecsr2csrByP.o -L/usr/local/cuda/lib64 -lcusparse -lcudart
*/
#include <stdio.h>
#include <stdlib.h>
#include <assert.h>
#include <cuda_runtime.h>
#include <cusparse.h>
void printCsr(
int m,
int n,
int nnz,
const cusparseMatDescr_t descrA,
const float *csrValA,
const int *csrRowPtrA,
const int *csrColIndA,
const char* name)
{
const int base = (cusparseGetMatIndexBase(descrA) != CUSPARSE_INDEX_BASE_ONE)? 0:1 ;
printf("matrix %s is %d-by-%d, nnz=%d, base=%d, output base-1\n", name, m, n, nnz, base);
for(int row = 0 ; row < m ; row++){
const int start = csrRowPtrA[row ] - base;
const int end = csrRowPtrA[row+1] - base;
for(int colidx = start ; colidx < end ; colidx++){
const int col = csrColIndA[colidx] - base;
const float Areg = csrValA[colidx];
printf("%s(%d,%d) = %f\n", name, row+1, col+1, Areg);
}
}
}
int main(int argc, char*argv[])
{
cusparseHandle_t handle = NULL;
cudaStream_t stream = NULL;
cusparseMatDescr_t descrA = NULL;
cusparseMatDescr_t descrC = NULL;
pruneInfo_t info = NULL;
cusparseStatus_t status = CUSPARSE_STATUS_SUCCESS;
cudaError_t cudaStat1 = cudaSuccess;
const int m = 4;
const int n = 4;
const int nnzA = 9;
/*
* | 1 0 2 -3 |
* | 0 4 0 0 |
* A = | 5 0 6 7 |
* | 0 8 0 9 |
*
*/
const int csrRowPtrA[m+1] = { 1, 4, 5, 8, 10};
const int csrColIndA[nnzA] = { 1, 3, 4, 2, 1, 3, 4, 2, 4};
const float csrValA[nnzA] = {1, 2, -3, 4, 5, 6, 7, 8, 9};
int* csrRowPtrC = NULL;
int* csrColIndC = NULL;
float* csrValC = NULL;
int *d_csrRowPtrA = NULL;
int *d_csrColIndA = NULL;
float *d_csrValA = NULL;
int *d_csrRowPtrC = NULL;
int *d_csrColIndC = NULL;
float *d_csrValC = NULL;
size_t lworkInBytes = 0;
char *d_work = NULL;
int nnzC = 0;
float percentage = 20; /* remove 20% of nonzeros */
printf("example of pruneCsr2csrByPercentage \n");
printf("prune %.1f percent of nonzeros \n", percentage);
/* step 1: create cusparse handle, bind a stream */
cudaStat1 = cudaStreamCreateWithFlags(&stream, cudaStreamNonBlocking);
assert(cudaSuccess == cudaStat1);
status = cusparseCreate(&handle);
assert(CUSPARSE_STATUS_SUCCESS == status);
status = cusparseSetStream(handle, stream);
assert(CUSPARSE_STATUS_SUCCESS == status);
status = cusparseCreatePruneInfo(&info);
assert(CUSPARSE_STATUS_SUCCESS == status);
/* step 2: configuration of matrix C */
status = cusparseCreateMatDescr(&descrA);
assert(CUSPARSE_STATUS_SUCCESS == status);
/* A is base-1*/
cusparseSetMatIndexBase(descrA,CUSPARSE_INDEX_BASE_ONE);
cusparseSetMatType(descrA, CUSPARSE_MATRIX_TYPE_GENERAL );
status = cusparseCreateMatDescr(&descrC);
assert(CUSPARSE_STATUS_SUCCESS == status);
/* C is base-0 */
cusparseSetMatIndexBase(descrC,CUSPARSE_INDEX_BASE_ZERO);
cusparseSetMatType(descrC, CUSPARSE_MATRIX_TYPE_GENERAL );
printCsr(m, n, nnzA, descrA, csrValA, csrRowPtrA, csrColIndA, "A");
cudaStat1 = cudaMalloc ((void**)&d_csrRowPtrA, sizeof(int)*(m+1) );
assert(cudaSuccess == cudaStat1);
cudaStat1 = cudaMalloc ((void**)&d_csrColIndA, sizeof(int)*nnzA );
assert(cudaSuccess == cudaStat1);
cudaStat1 = cudaMalloc ((void**)&d_csrValA , sizeof(float)*nnzA );
assert(cudaSuccess == cudaStat1);
cudaStat1 = cudaMalloc ((void**)&d_csrRowPtrC, sizeof(int)*(m+1) );
assert(cudaSuccess == cudaStat1);
cudaStat1 = cudaMemcpy(d_csrRowPtrA, csrRowPtrA, sizeof(int)*(m+1), cudaMemcpyHostToDevice);
assert(cudaSuccess == cudaStat1);
cudaStat1 = cudaMemcpy(d_csrColIndA, csrColIndA, sizeof(int)*nnzA, cudaMemcpyHostToDevice);
assert(cudaSuccess == cudaStat1);
cudaStat1 = cudaMemcpy(d_csrValA , csrValA , sizeof(float)*nnzA, cudaMemcpyHostToDevice);
assert(cudaSuccess == cudaStat1);
/* step 3: query workspace */
status = cusparseSpruneCsr2csrByPercentage_bufferSizeExt(
handle,
m,
n,
nnzA,
descrA,
d_csrValA,
d_csrRowPtrA,
d_csrColIndA,
percentage,
descrC,
d_csrValC,
d_csrRowPtrC,
d_csrColIndC,
info,
&lworkInBytes);
assert(CUSPARSE_STATUS_SUCCESS == status);
printf("lworkInBytes = %lld \n", (long long)lworkInBytes);
if (NULL != d_work) { cudaFree(d_work); }
cudaStat1 = cudaMalloc((void**)&d_work, lworkInBytes);
assert(cudaSuccess == cudaStat1);
/* step 4: compute csrRowPtrC and nnzC */
status = cusparseSpruneCsr2csrNnzByPercentage(
handle,
m,
n,
nnzA,
descrA,
d_csrValA,
d_csrRowPtrA,
d_csrColIndA,
percentage,
descrC,
d_csrRowPtrC,
&nnzC, /* host */
info,
d_work);
assert(CUSPARSE_STATUS_SUCCESS == status);
cudaStat1 = cudaDeviceSynchronize();
assert(cudaSuccess == cudaStat1);
printf("nnzC = %d\n", nnzC);
if (0 == nnzC ){
printf("C is empty \n");
return 0;
}
/* step 5: compute csrColIndC and csrValC */
cudaStat1 = cudaMalloc ((void**)&d_csrColIndC, sizeof(int ) * nnzC );
assert(cudaSuccess == cudaStat1);
cudaStat1 = cudaMalloc ((void**)&d_csrValC , sizeof(float) * nnzC );
assert(cudaSuccess == cudaStat1);
status = cusparseSpruneCsr2csrByPercentage(
handle,
m,
n,
nnzA,
descrA,
d_csrValA,
d_csrRowPtrA,
d_csrColIndA,
percentage,
descrC,
d_csrValC,
d_csrRowPtrC,
d_csrColIndC,
info,
d_work);
assert(CUSPARSE_STATUS_SUCCESS == status);
cudaStat1 = cudaDeviceSynchronize();
assert(cudaSuccess == cudaStat1);
/* step 6: output C */
csrRowPtrC = (int* )malloc(sizeof(int )*(m+1));
csrColIndC = (int* )malloc(sizeof(int )*nnzC);
csrValC = (float*)malloc(sizeof(float)*nnzC);
assert( NULL != csrRowPtrC);
assert( NULL != csrColIndC);
assert( NULL != csrValC);
cudaStat1 = cudaMemcpy(csrRowPtrC, d_csrRowPtrC, sizeof(int )*(m+1), cudaMemcpyDeviceToHost);
assert(cudaSuccess == cudaStat1);
cudaStat1 = cudaMemcpy(csrColIndC, d_csrColIndC, sizeof(int )*nnzC , cudaMemcpyDeviceToHost);
assert(cudaSuccess == cudaStat1);
cudaStat1 = cudaMemcpy(csrValC , d_csrValC , sizeof(float)*nnzC , cudaMemcpyDeviceToHost);
assert(cudaSuccess == cudaStat1);
printCsr(m, n, nnzC, descrC, csrValC, csrRowPtrC, csrColIndC, "C");
/* free resources */
if (d_csrRowPtrA) cudaFree(d_csrRowPtrA);
if (d_csrColIndA) cudaFree(d_csrColIndA);
if (d_csrValA ) cudaFree(d_csrValA);
if (d_csrRowPtrC) cudaFree(d_csrRowPtrC);
if (d_csrColIndC) cudaFree(d_csrColIndC);
if (d_csrValC ) cudaFree(d_csrValC);
if (csrRowPtrC ) free(csrRowPtrC);
if (csrColIndC ) free(csrColIndC);
if (csrValC ) free(csrValC);
if (handle ) cusparseDestroy(handle);
if (stream ) cudaStreamDestroy(stream);
if (descrA ) cusparseDestroyMatDescr(descrA);
if (descrC ) cusparseDestroyMatDescr(descrC);
if (info ) cusparseDestroyPruneInfo(info);
cudaDeviceReset();
return 0;
}
17. Examples of csrsm2
17.1. Forward Triangular Solver
This section provides a simple example in the C programming language of csrsm2.
The example solves a lower triangular system with 2 right hand side vectors.
/*
* How to compile (assume cuda is installed at /usr/local/cuda/)
* nvcc -c -I/usr/local/cuda/include csrms2.cpp
* g++ -o csrm2 csrsm2.o -L/usr/local/cuda/lib64 -lcusparse -lcudart
*/
#include <stdio.h>
#include <stdlib.h>
#include <assert.h>
#include <cuda_runtime.h>
#include <cusparse.h>
/* compute | b - A*x|_inf */
void residaul_eval(
int n,
const cusparseMatDescr_t descrA,
const float *csrVal,
const int *csrRowPtr,
const int *csrColInd,
const float *b,
const float *x,
float *r_nrminf_ptr)
{
const int base = (cusparseGetMatIndexBase(descrA) != CUSPARSE_INDEX_BASE_ONE)? 0:1 ;
const int lower = (CUSPARSE_FILL_MODE_LOWER == cusparseGetMatFillMode(descrA))? 1:0;
const int unit = (CUSPARSE_DIAG_TYPE_UNIT == cusparseGetMatDiagType(descrA))? 1:0;
float r_nrminf = 0;
for(int row = 0 ; row < n ; row++){
const int start = csrRowPtr[row] - base;
const int end = csrRowPtr[row+1] - base;
float dot = 0;
for(int colidx = start ; colidx < end; colidx++){
const int col = csrColInd[colidx] - base;
float Aij = csrVal[colidx];
float xj = x[col];
if ( (row == col) && unit ){
Aij = 1.0;
}
int valid = (row >= col) && lower ||
(row <= col) && !lower ;
if ( valid ){
dot += Aij*xj;
}
}
float ri = b[row] - dot;
r_nrminf = (r_nrminf > fabs(ri))? r_nrminf : fabs(ri);
}
*r_nrminf_ptr = r_nrminf;
}
int main(int argc, char*argv[])
{
cusparseHandle_t handle = NULL;
cudaStream_t stream = NULL;
cusparseMatDescr_t descrA = NULL;
csrsm2Info_t info = NULL;
cusparseStatus_t status = CUSPARSE_STATUS_SUCCESS;
cudaError_t cudaStat1 = cudaSuccess;
const int nrhs = 2;
const int n = 4;
const int nnzA = 9;
const cusparseSolvePolicy_t policy = CUSPARSE_SOLVE_POLICY_NO_LEVEL;
const float h_one = 1.0;
/*
* | 1 0 2 -3 |
* | 0 4 0 0 |
* A = | 5 0 6 7 |
* | 0 8 0 9 |
*
* Regard A as a lower triangle matrix L with non-unit diagonal.
* | 1 5 | | 1 5 |
* Given B = | 2 6 |, X = L \ B = | 0.5 1.5 |
* | 3 7 | | -0.3333 -3 |
* | 4 8 | | 0 -0.4444 |
*/
const int csrRowPtrA[n+1] = { 1, 4, 5, 8, 10};
const int csrColIndA[nnzA] = { 1, 3, 4, 2, 1, 3, 4, 2, 4};
const float csrValA[nnzA] = {1, 2, -3, 4, 5, 6, 7, 8, 9};
const float B[n*nrhs] = {1,2,3,4,5,6,7,8};
float X[n*nrhs];
int *d_csrRowPtrA = NULL;
int *d_csrColIndA = NULL;
float *d_csrValA = NULL;
float *d_B = NULL;
size_t lworkInBytes = 0;
char *d_work = NULL;
const int algo = 0; /* non-block version */
printf("example of csrsm2 \n");
/* step 1: create cusparse handle, bind a stream */
cudaStat1 = cudaStreamCreateWithFlags(&stream, cudaStreamNonBlocking);
assert(cudaSuccess == cudaStat1);
status = cusparseCreate(&handle);
assert(CUSPARSE_STATUS_SUCCESS == status);
status = cusparseSetStream(handle, stream);
assert(CUSPARSE_STATUS_SUCCESS == status);
status = cusparseCreateCsrsm2Info(&info);
assert(CUSPARSE_STATUS_SUCCESS == status);
/* step 2: configuration of matrix A */
status = cusparseCreateMatDescr(&descrA);
assert(CUSPARSE_STATUS_SUCCESS == status);
/* A is base-1*/
cusparseSetMatIndexBase(descrA,CUSPARSE_INDEX_BASE_ONE);
cusparseSetMatType(descrA, CUSPARSE_MATRIX_TYPE_GENERAL);
/* A is lower triangle */
cusparseSetMatFillMode(descrA, CUSPARSE_FILL_MODE_LOWER);
/* A has non unit diagonal */
cusparseSetMatDiagType(descrA, CUSPARSE_DIAG_TYPE_NON_UNIT);
cudaStat1 = cudaMalloc ((void**)&d_csrRowPtrA, sizeof(int)*(n+1) );
assert(cudaSuccess == cudaStat1);
cudaStat1 = cudaMalloc ((void**)&d_csrColIndA, sizeof(int)*nnzA );
assert(cudaSuccess == cudaStat1);
cudaStat1 = cudaMalloc ((void**)&d_csrValA , sizeof(float)*nnzA );
assert(cudaSuccess == cudaStat1);
cudaStat1 = cudaMalloc ((void**)&d_B , sizeof(float)*n*nrhs );
assert(cudaSuccess == cudaStat1);
cudaStat1 = cudaMemcpy(d_csrRowPtrA, csrRowPtrA, sizeof(int)*(n+1), cudaMemcpyHostToDevice);
assert(cudaSuccess == cudaStat1);
cudaStat1 = cudaMemcpy(d_csrColIndA, csrColIndA, sizeof(int)*nnzA, cudaMemcpyHostToDevice);
assert(cudaSuccess == cudaStat1);
cudaStat1 = cudaMemcpy(d_csrValA , csrValA , sizeof(float)*nnzA, cudaMemcpyHostToDevice);
assert(cudaSuccess == cudaStat1);
cudaStat1 = cudaMemcpy(d_B , B , sizeof(float)*n*nrhs, cudaMemcpyHostToDevice);
assert(cudaSuccess == cudaStat1);
/* step 3: query workspace */
status = cusparseScsrsm2_bufferSizeExt(
handle,
algo,
CUSPARSE_OPERATION_NON_TRANSPOSE, /* transA */
CUSPARSE_OPERATION_NON_TRANSPOSE, /* transB */
n,
nrhs,
nnzA,
&h_one,
descrA,
d_csrValA,
d_csrRowPtrA,
d_csrColIndA,
d_B,
n, /* ldb */
info,
policy,
&lworkInBytes);
assert(CUSPARSE_STATUS_SUCCESS == status);
printf("lworkInBytes = %lld \n", (long long)lworkInBytes);
if (NULL != d_work) { cudaFree(d_work); }
cudaStat1 = cudaMalloc((void**)&d_work, lworkInBytes);
assert(cudaSuccess == cudaStat1);
/* step 4: analysis */
status = cusparseScsrsm2_analysis(
handle,
algo,
CUSPARSE_OPERATION_NON_TRANSPOSE, /* transA */
CUSPARSE_OPERATION_NON_TRANSPOSE, /* transB */
n,
nrhs,
nnzA,
&h_one,
descrA,
d_csrValA,
d_csrRowPtrA,
d_csrColIndA,
d_B,
n, /* ldb */
info,
policy,
d_work);
assert(CUSPARSE_STATUS_SUCCESS == status);
/* step 5: solve L * X = B */
status = cusparseScsrsm2_solve(
handle,
algo,
CUSPARSE_OPERATION_NON_TRANSPOSE, /* transA */
CUSPARSE_OPERATION_NON_TRANSPOSE, /* transB */
n,
nrhs,
nnzA,
&h_one,
descrA,
d_csrValA,
d_csrRowPtrA,
d_csrColIndA,
d_B,
n, /* ldb */
info,
policy,
d_work);
assert(CUSPARSE_STATUS_SUCCESS == status);
cudaStat1 = cudaDeviceSynchronize();
assert(cudaSuccess == cudaStat1);
/* step 6:measure residual B - A*X */
cudaStat1 = cudaMemcpy(X, d_B, sizeof(float)*n*nrhs, cudaMemcpyDeviceToHost);
assert(cudaSuccess == cudaStat1);
cudaDeviceSynchronize();
printf("==== x1 = inv(A)*b1 \n");
for(int j = 0 ; j < n; j++){
printf("x1[%d] = %f\n", j, X[j]);
}
float r1_nrminf;
residaul_eval(
n,
descrA,
csrValA,
csrRowPtrA,
csrColIndA,
B,
X,
&r1_nrminf
);
printf("|b1 - A*x1| = %E\n", r1_nrminf);
printf("==== x2 = inv(A)*b2 \n");
for(int j = 0 ; j < n; j++){
printf("x2[%d] = %f\n", j, X[n+j]);
}
float r2_nrminf;
residaul_eval(
n,
descrA,
csrValA,
csrRowPtrA,
csrColIndA,
B+n,
X+n,
&r2_nrminf
);
printf("|b2 - A*x2| = %E\n", r2_nrminf);
/* free resources */
if (d_csrRowPtrA ) cudaFree(d_csrRowPtrA);
if (d_csrColIndA ) cudaFree(d_csrColIndA);
if (d_csrValA ) cudaFree(d_csrValA);
if (d_B ) cudaFree(d_B);
if (handle ) cusparseDestroy(handle);
if (stream ) cudaStreamDestroy(stream);
if (descrA ) cusparseDestroyMatDescr(descrA);
if (info ) cusparseDestroyCsrsm2Info(info);
cudaDeviceReset();
return 0;
}
18. Acknowledgements
NVIDIA would like to thank the following individuals and institutions for their contributions:
The cusparse<t>gtsv implementation is derived from a version developed by Li-Wen Chang from the University of Illinois.
The cusparse<t>gtsvInterleavedBatch adopts cuThomasBatch developed by Pedro Valero-Lara and Ivan Martínez-Pérez from Barcelona Supercomputing Center and BSC/UPC NVIDIA GPU Center of Excellence.
This product includes {fmt} - A modern formatting library https://fmt.dev Copyright (c) 2012 - present, Victor Zverovich.
19. Bibliography
[1] N. Bell and M. Garland, “Implementing Sparse Matrix-Vector Multiplication on Throughput-Oriented Processors”, Supercomputing, 2009.
[2] R. Grimes, D. Kincaid, and D. Young, “ITPACK 2.0 User’s Guide”, Technical Report CNA-150, Center for Numerical Analysis, University of Texas, 1979.
[3] M. Naumov, “Incomplete-LU and Cholesky Preconditioned Iterative Methods Using cuSPARSE and cuBLAS”, Technical Report and White Paper, 2011.
[4] Pedro Valero-Lara, Ivan Martínez-Pérez, Raül Sirvent, Xavier Martorell, and Antonio J. Peña. NVIDIA GPUs Scalability to Solve Multiple (Batch) Tridiagonal Systems. Implementation of cuThomasBatch. In Parallel Processing and Applied Mathematics - 12th International Conference (PPAM), 2017.
20. Notices
20.1. Notice
This document is provided for information purposes only and shall not be regarded as a warranty of a certain functionality, condition, or quality of a product. NVIDIA Corporation (“NVIDIA”) makes no representations or warranties, expressed or implied, as to the accuracy or completeness of the information contained in this document and assumes no responsibility for any errors contained herein. NVIDIA shall have no liability for the consequences or use of such information or for any infringement of patents or other rights of third parties that may result from its use. This document is not a commitment to develop, release, or deliver any Material (defined below), code, or functionality.
NVIDIA reserves the right to make corrections, modifications, enhancements, improvements, and any other changes to this document, at any time without notice.
Customer should obtain the latest relevant information before placing orders and should verify that such information is current and complete.
NVIDIA products are sold subject to the NVIDIA standard terms and conditions of sale supplied at the time of order acknowledgement, unless otherwise agreed in an individual sales agreement signed by authorized representatives of NVIDIA and customer (“Terms of Sale”). NVIDIA hereby expressly objects to applying any customer general terms and conditions with regards to the purchase of the NVIDIA product referenced in this document. No contractual obligations are formed either directly or indirectly by this document.
NVIDIA products are not designed, authorized, or warranted to be suitable for use in medical, military, aircraft, space, or life support equipment, nor in applications where failure or malfunction of the NVIDIA product can reasonably be expected to result in personal injury, death, or property or environmental damage. NVIDIA accepts no liability for inclusion and/or use of NVIDIA products in such equipment or applications and therefore such inclusion and/or use is at customer’s own risk.
NVIDIA makes no representation or warranty that products based on this document will be suitable for any specified use. Testing of all parameters of each product is not necessarily performed by NVIDIA. It is customer’s sole responsibility to evaluate and determine the applicability of any information contained in this document, ensure the product is suitable and fit for the application planned by customer, and perform the necessary testing for the application in order to avoid a default of the application or the product. Weaknesses in customer’s product designs may affect the quality and reliability of the NVIDIA product and may result in additional or different conditions and/or requirements beyond those contained in this document. NVIDIA accepts no liability related to any default, damage, costs, or problem which may be based on or attributable to: (i) the use of the NVIDIA product in any manner that is contrary to this document or (ii) customer product designs.
No license, either expressed or implied, is granted under any NVIDIA patent right, copyright, or other NVIDIA intellectual property right under this document. Information published by NVIDIA regarding third-party products or services does not constitute a license from NVIDIA to use such products or services or a warranty or endorsement thereof. Use of such information may require a license from a third party under the patents or other intellectual property rights of the third party, or a license from NVIDIA under the patents or other intellectual property rights of NVIDIA.
Reproduction of information in this document is permissible only if approved in advance by NVIDIA in writing, reproduced without alteration and in full compliance with all applicable export laws and regulations, and accompanied by all associated conditions, limitations, and notices.
THIS DOCUMENT AND ALL NVIDIA DESIGN SPECIFICATIONS, REFERENCE BOARDS, FILES, DRAWINGS, DIAGNOSTICS, LISTS, AND OTHER DOCUMENTS (TOGETHER AND SEPARATELY, “MATERIALS”) ARE BEING PROVIDED “AS IS.” NVIDIA MAKES NO WARRANTIES, EXPRESSED, IMPLIED, STATUTORY, OR OTHERWISE WITH RESPECT TO THE MATERIALS, AND EXPRESSLY DISCLAIMS ALL IMPLIED WARRANTIES OF NONINFRINGEMENT, MERCHANTABILITY, AND FITNESS FOR A PARTICULAR PURPOSE. TO THE EXTENT NOT PROHIBITED BY LAW, IN NO EVENT WILL NVIDIA BE LIABLE FOR ANY DAMAGES, INCLUDING WITHOUT LIMITATION ANY DIRECT, INDIRECT, SPECIAL, INCIDENTAL, PUNITIVE, OR CONSEQUENTIAL DAMAGES, HOWEVER CAUSED AND REGARDLESS OF THE THEORY OF LIABILITY, ARISING OUT OF ANY USE OF THIS DOCUMENT, EVEN IF NVIDIA HAS BEEN ADVISED OF THE POSSIBILITY OF SUCH DAMAGES. Notwithstanding any damages that customer might incur for any reason whatsoever, NVIDIA’s aggregate and cumulative liability towards customer for the products described herein shall be limited in accordance with the Terms of Sale for the product.
20.2. OpenCL
OpenCL is a trademark of Apple Inc. used under license to the Khronos Group Inc.
20.3. Trademarks
NVIDIA and the NVIDIA logo are trademarks or registered trademarks of NVIDIA Corporation in the U.S. and other countries. Other company and product names may be trademarks of the respective companies with which they are associated.
20.4. Copyright
© 2007-2023, NVIDIA Corporation & Affiliates. All rights reserved.