Overview#
DiffDock is a state-of-the-art generative model used for drug discovery that predicts the three-dimensional structure of a protein-ligand complex, a crucial step in the drug discovery process. It predicts the binding structure of a small molecule ligand to a protein, known as molecular docking or pose prediction.
DiffDock can:
Helps AI drug discovery pipelines and opens new research avenues for downstream task integrations.
Highly accurate and computationally efficient
Fast inference times and provides confidence estimates with high selective accuracy.
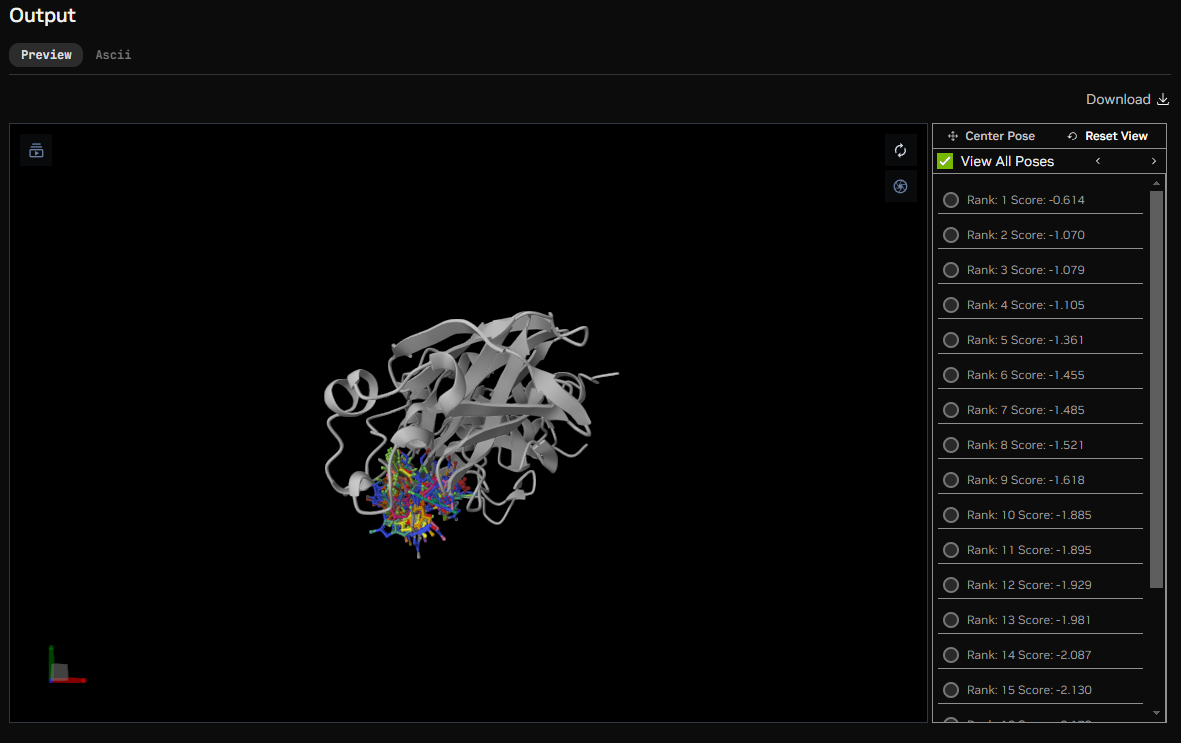
Note
A more detailed description of the model can be found in the Model Card.
DiffDock is an equivariant geometric model for blind molecular docking pose estimation. It requires protein and molecule 3D structures as input and does not require any information about a binding pocket. During its diffusion process, the molecule’s position relative to the protein, its orientation, and the torsion angles can change. By running the learned reverse diffusion process, it transforms a distribution of noisy prior molecule poses to the one learned by the model. As a result, it outputs many sampled poses and ranks them via its confidence model.