Guardrail Concepts#
The NeMo Guardrails microservice is a high-performance, flexible way to improve the safety, security, and trustworthiness of any LLM-based application. While today’s LLMs incorporate rigorous safety alignment into their pre and post-training phases, there are still instances in which they return unsafe or inappropriate answers. Deploying NeMo Guardrails augments an LLM’s safety-alignment while maintaining the same interface for clients. The following diagram shows an example NeMo Guardrails deployment using the NVIDIA Content Safety NIM to protect an existing application.
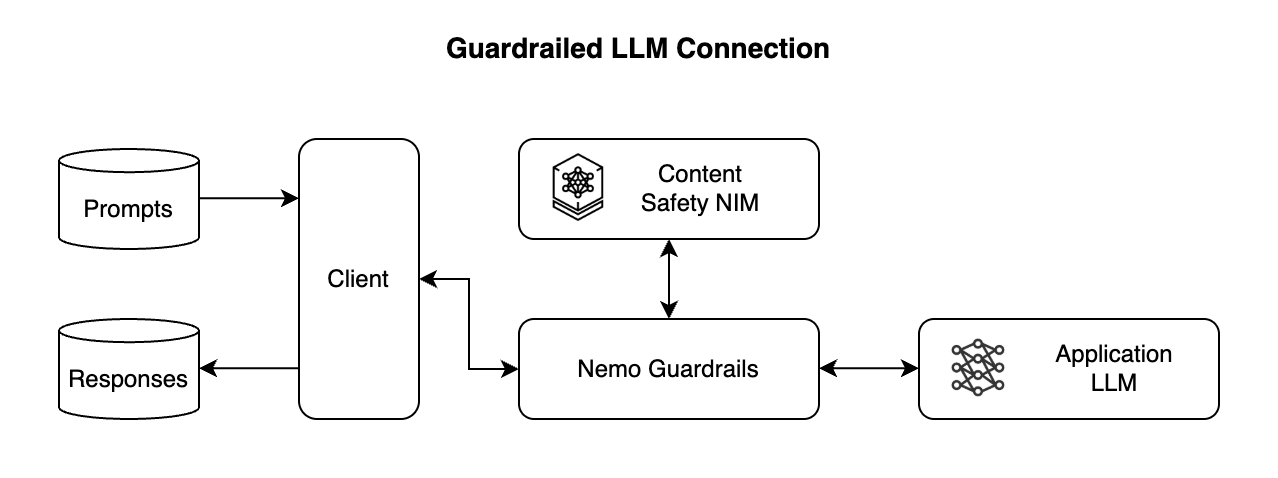
Prompt and Response Checking#
You can configure the NeMo Guardrails microservice to perform both prompt and LLM response-checking using the application LLM, NVIDIA NIM microservices, or third-party models.
Using the Application LLM#
Self-checking guardrails use the same LLM as the end-application. These guardrails can be applied on the user input, LLM response, or both. To configure these checks, you provide a text prompt and Python parser function. The prompt asks the application LLM to determine whether the user input and/or LLM response are safe. The parser function processes the response from this prompt, mapping the free-text output of the LLM to a boolean safety assessment.
Using NVIDIA NIM Microservices#
The NeMo Guardrails microservice is closely integrated with several NIM microservices, with pre-configured prompts and parser functions. Rather than using the application LLM, these NIM microservices use smaller models fine-tuned to the task to reduce latency. These microservices provide a low-friction option to deploy protections against the most common threats to safety and security.
Content Safety: This model checks user input and LLM response against 23 categories of unsafe content, and overrides unsafe LLM responses with a safe refusal to answer.
Topic Control: This model prevents multi-turn conversations from veering off-topic over a number of turns.
Jailbreak Detection: This model detects prompt injection and jailbreak attacks against an LLM, which can result in unwanted responses and behavior.
Using Third-Party Models and APIs#
NeMo Guardrails also supports third-party models and APIs. Supported models include AlignScore-based Fact-Checking, Llama Guard Content Moderation, and Sensitive Data Detection using Presidio. Support for third-party APIs also includes AutoAlign, Cleanlab, GCP Text Moderation, Private AI PII Detection, and many others.
Guiding Conversational Flows using Colang#
Colang is a Domain-Specific Language to model conversational flows between a user and chatbot application. You can define a conversational flow using one or more example text-strings. The Colang runtime then uses a three-step workflow.
Determine user intent. Determine the most likely user intent, given the pre-defined list of user intents with example user input.
Determine the next step. If the user-intent matches a dialog flow, this is selected for use in the next step.
Generate LLM response. If a dialog-flow is matched, return one of the corresponding response. If not, use an LLM to generate the response.
Additional Features#
The NeMo Guardrails microservice supports streaming responses, reducing the time-to-first-token for a low-latency user experience.