BrightnessContrast Example#
This example demonstrates usage of brightness_contrast
operator.
Brighness and Contrast Adjustment#
Brithness and contrast can be adjusted using three parameters: contrast
- makes the dark parts darker and bright parts brighter while keeping the gray (at half dynamic range of the input type) constant,
brightness
- multiplies the intensities,
brightness_shift
- shifts the pixel intensities.
The formula is given as:
where \({contrast\_center}\) denotes a value that is unaffected by contrast changes; by default, it’s at half of the input’s dynamic range (e.g. 128 for uint8
) and \({Max}_{out}\) is the maximum positive value of the output type, for integer types, or 1 for floating point) - but it can also be specified as an argument for the operator. The operator can be used to adjust contrast and brighness as well as to compute negative images (brightness = -1
, brighness_shift = 1
).
Step-by-Step Guide#
Let’s start from importing necessary modules (and DALI itself).
[1]:
from nvidia.dali import pipeline_def
import nvidia.dali.fn as fn
import nvidia.dali.types as types
import matplotlib.pyplot as plt
batch_size = 10
image_filename = "../data/images"
The batch_size
is bigger than one to make it easier to switch images at the end of the example.
Next, let’s implement the pipelines. We’ve presented 2 versions of a pipeline. The CPU one does all the processing (i.e. reading file, decoding it and brightness/contrast manipulation) on the CPU, while the other pipeline conducts these operations on the GPU.
[2]:
@pipeline_def()
def bc_cpu_pipeline():
files, labels = fn.readers.file(file_root=image_filename)
images = fn.decoders.image(files)
converted = fn.brightness_contrast(
images, brightness_shift=0.3, contrast=0.4, contrast_center=100
)
return images, converted
@pipeline_def()
def bc_gpu_pipeline():
files, labels = fn.readers.file(file_root=image_filename)
images = fn.decoders.image(files, device="mixed")
converted = fn.brightness_contrast(
# increase contrast # invert...
images,
contrast=1.5,
brightness_shift=1,
brightness=-1,
) # ...colors
return images, converted
The function below is used to actually display result of manipulation. Since the pipelines we set up return 2 outputs: modified image and original image, the function aquires both of them from the output and displays them. Additional flag (cpu
) is specified, to determine, whether the pipeline output comes from CPU or GPU. In the latter case, we have to tell the output, to return a CPU-accessible copy of the data.
[3]:
def display(output, cpu=True):
i = 2 # Tweak that to have various images from batch
img1 = output[0].at(i) if cpu else output[0].as_cpu().at(i)
img2 = output[1].at(i) if cpu else output[1].as_cpu().at(i)
fig, ax = plt.subplots(1, 2)
ax[0].imshow(img1)
ax[1].imshow(img2);
Now let’s just build the pipelines, run them and display the results. First the GPU one:
[4]:
pipe_gpu = bc_gpu_pipeline(batch_size=batch_size, num_threads=1, device_id=0)
pipe_gpu.build()
[5]:
gpu_output = pipe_gpu.run()
[6]:
display(gpu_output, cpu=False)
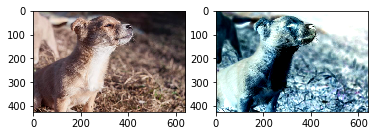
And the CPU:
[7]:
pipe_cpu = bc_cpu_pipeline(batch_size=batch_size, num_threads=1, device_id=0)
pipe_cpu.build()
cpu_output = pipe_cpu.run()
[8]:
display(cpu_output)
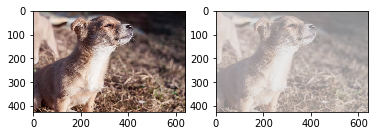