TTS Overview
Contents
TTS Overview#
The text-to-speech (TTS) pipeline implemented for the Riva TTS service is based on a two-stage pipeline. Riva models like FastPitch and RadTTS++ first generates a mel-spectrogram, and then generates speech using the HifiGAN model while MagpieTTS Multilingual generates tokens and then generates speech using the Audio Codec model. This pipeline forms a TTS system that enables you to synthesize natural sounding speech from raw transcripts without any additional information such as patterns or rhythms of speech.
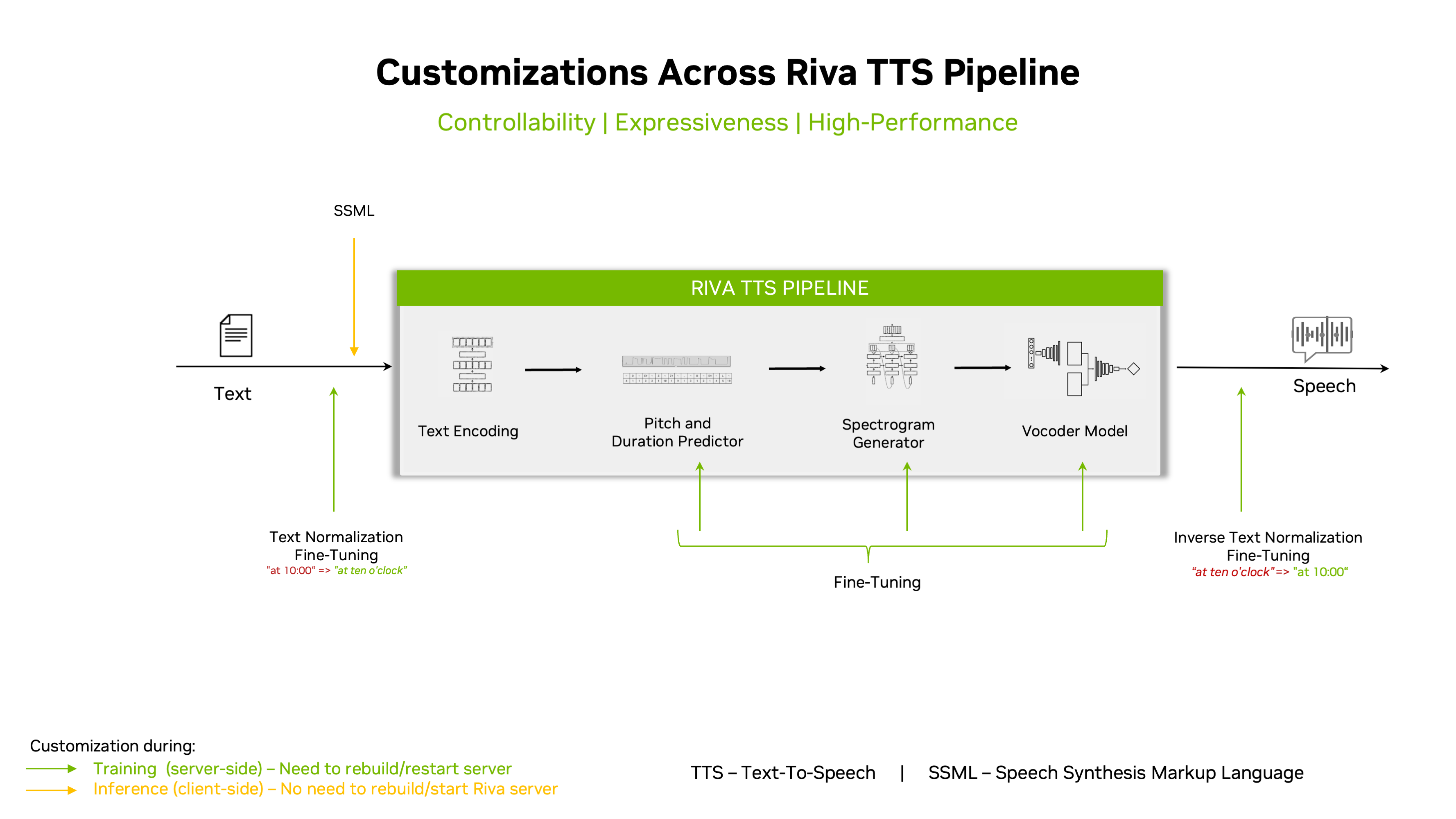
Riva TTS supports both streaming and offline inference modes. In offline mode, audio is not returned until the full audio sequence for the requested text is generated and can achieve higher throughput. When making a streaming request, audio chunks are returned as soon as they are generated, significantly reducing the latency (as measured by time to first audio) for large requests.
Try It Out#
Experience Riva TTS on our demo platform: https://build.nvidia.com/explore/speech
Pretrained TTS Models#
The .riva
models used to generate the RMIRs in the Quick Start scripts can be found at the following NGC locations. Supported voice names and samples generated with these models are also mentioned in the following table.
Language |
Model |
Dataset |
G2P |
Gender |
Voices |
Voice Samples |
---|---|---|---|---|---|---|
English, French, Spanish (en-US, fr-FR, es-US) |
Multilingual |
IPA |
Multi-speaker |
|
|
|
English (en-US) |
English-US |
IPA |
Multi-speaker |
|
||
English (en-US) |
English-US |
IPA |
Multi-speaker |
|
||
English (en-US) |
LJSpeech |
ARPABET |
|
|||
English (en-US) |
English-US |
ARPABET |
Multi-speaker |
|
||
Mandarin (zh-CN) |
Mandarin-CN |
IPA |
Multi-speaker |
|
||
Spanish (es-ES) |
Public/Proprietary |
IPA |
Female |
|
||
Spanish (es-ES) |
Public/Proprietary |
IPA |
Male |
|
||
Spanish-US (es-US) |
Public/Proprietary |
IPA |
Multi-speaker |
|
||
Italian (it-IT) |
Public/Proprietary |
IPA |
Female |
|
||
Italian (it-IT) |
Public/Proprietary |
IPA |
Male |
|
||
German (de-DE) |
Public/Proprietary |
IPA |
Male |
|
Features#
Riva TTS supports the following features:
Streaming and offline inference modes
Output audio encoding
SSML input
Zero Shot TTS (Beta Feature)
Emotion mixing (Beta Feature)
Custom pronunciation dictionary
Language and Model Support#
Riva Speech AI Skills provides pretrained models across a variety of languages that are listed in above section. Upgraded models and new languages are released regularly.
To select which language and model to deploy, simply change the variables tts_model
and tts_language_code
in the config.sh
file within the quickstart
directory of the Quick Start scripts.
Zero Shot TTS (Beta Feature)#
Riva introduces Zero Shot TTS as a beta feature. This feature allows users to provide a speech prompt, enabling the model to adapt to the voice in prompt and synthesize speech using it.
SSML input#
Riva TTS supports SSML inputs for both streaming and offline inference modes. Using SSML tags to provide finer control over the generated speech, users can specify the following tags: prosody
, phoneme
, and sub
. You can use the phoneme
tag to specify the phoneme for a given word, the sub
tag to specify the substitution for a given word, and the prosody
tag to specify the prosody attributes like pitch
, rate
, and volume
for a given text. Refer to the notebook here for more details.
Model |
|
|
|
---|---|---|---|
FastPitch |
β |
β |
β |
RadTTS++ |
β |
β |
β |
MagpieTTS Multilingual |
β |
Emotion mixing (Beta feature)#
Riva now supports the mixing of emotional intensities as a beta feature. This will allow the users to control the emotion in an audio. This feature is accessible through SSML emotion attribute. Currently the quantization is only supported for calm
, angry
, fearful
, neutral
and happy
for Female
, and calm
, happy
and neutral
for male
. Currently emotion mixing is only supported in the RadTTS++ model.
Custom pronunciation dictionary#
Riva TTS supports providing a text dictionary to get the desired pronunciation for specific words synthesized by the server. This custom dictionary must contain a word (grapheme) followed by the desired pronunciation (phoneme), both separated by two spaces. Different such words and pronunciation pairs can be provided on a new line in the input dictionary file. The input dictionary file can be passed in the custom_dictionary
field while configuring a request from the client. In the Python client, this can be done by passing the dictionary in the custom_dictionary
field while configuring a request from the client like here.
Checking deployed models#
Once a server is running, retrieving the available models can be done via the GetRivaSynthesisConfig
RPC.
For each model available to make inference requests, the RPC returns the parameters used when the model was deployed.
Output Audio Encoding#
Besides the default Pulse-Code Modulation (PCM) output stream, you can choose Opus encoded and compressed stream. Compression enables you to significantly reduce the network bandwidth.